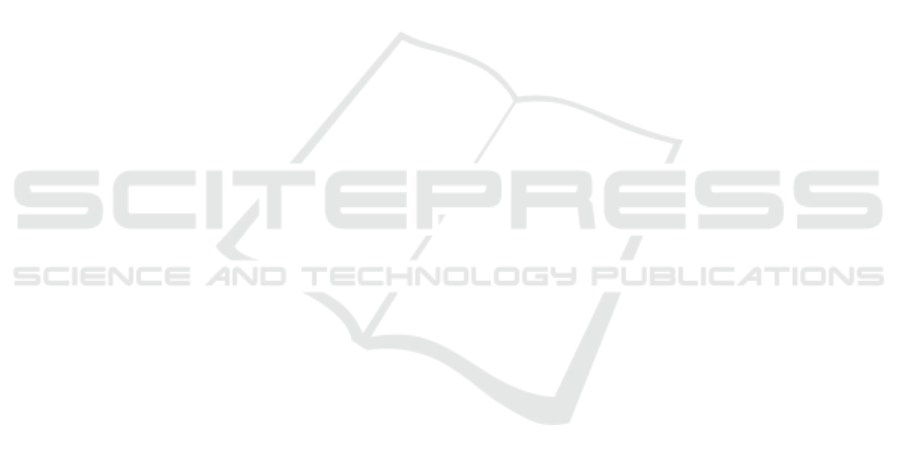
6 CONCLUSIONS
This study implements all variances of RRM consist-
ing of CRRM, µRRM, and PRRM. To compare which
results are better between RUM and RRM, we use
the statistic tests of Final Loglikelihood, Rho-square,
Akaike and Bayesian Information Criterion, and Hit
Rate. Our modelling result indicates that RRM out-
performs RUM. Even though RUM still produce the
better rho square, RRM could produce the lower of
Final Loglikelihood and Akaike and Bayesian Infor-
mation Criterion. The probability of choice gener-
ated by RRM could estimate more precisely shown
by the value of hit rate and the average probability
value for chosen mode and non-chosen mode. Among
all variances of RRM, it can be generally concluded
that µRRM could produce the best model fit although
there is a propensity that PRRM delivers a better
model fit than µRRM in a case of travel mode choice
in Palembang.
The value of travel time saving produced by RUM
and RRM shows that RRM tends to provide a higher
VTTS than RUM. The highest VTTS in both cities
generated by RRM is on LRT mode indicating that
people are willing to pay more when using LRT if
there is a reduction in the travel time unit. Meanwhile,
the demand elasticity shows that the travel time elas-
ticity generated by RUM and all variances of RRM
(except µRRM in Yogyakarta) is higher than the cost
elasticity showing that travellers are more concerned
with the travel time than travel cost in deciding what
kind of transport means that they use. Both RUM
and RRM produce the lowest cost elasticity for mo-
torcycle mode and lowest travel time elasticity for bus
mode, saying that motorcyclists and bus users are the
most unwilling travellers to shift to other modes due
to the change of travel cost and travel time respec-
tively.
REFERENCES
Bastarianto, F. F., Irawan, M. Z., Choudhury, C., Palma, D.,
and Muthohar, I. (2019). A tour-based mode choice
model for commuters in indonesia. Sustainability,
11(3):788.
BELGIAWAN, P. F., Ilahi, A., and Axhausen, K. W. (2017).
Bali trans sarbagita: Comparison between utility max-
imization and regret minimization. In Proceedings of
the Eastern Asia Society for Transportation Studies,
volume 11. Eastern Asia Society for Transportation
Studies.
Belgiawan, P. F., Ilahi, A., and Axhausen, K. W. (2019). In-
fluence of pricing on mode choice decision in jakarta:
A random regret minimization model. Case Studies
on Transport Policy, 7(1):87–95.
Belgiawan, P. F., Schmid, B., Dubernet, I., and Axhausen,
K. W. (2017). Comparison between rum, rrm variants,
and ram: Swiss sp and rp data sets. In 17th Swiss
Transport Research Conference (STRC 2017). STRC.
Ben-Akiva, M. E., Lerman, S. R., and Lerman, S. R. (1985).
Discrete choice analysis: theory and application to
travel demand, volume 9. MIT press.
Bierlaire, M. (2016). Pythonbiogeme: a short introduction
report transp-or 160706 transport and mobility labo-
ratory, school of architecture, civil and environmental
engineering, ecole polytecnique f
´
ed
´
erale de lausanne.
Boeri, M. and Masiero, L. (2014). Regret minimisation
and utility maximisation in a freight transport context.
Transportmetrica A: Transport Science, 10(6):548–
560.
Budi, N. S. and ZUSMAN, E. (2015). Governing public
transport improvement program in indonesia:-a com-
parative case study of trans musi palembang and trans
metro bandung. Journal of the Eastern Asia Society
for Transportation Studies, 11:2528–2542.
Chorus, C., van Cranenburgh, S., and Dekker, T. (2014).
Random regret minimization for consumer choice
modeling: Assessment of empirical evidence. Jour-
nal of Business Research, 67(11):2428–2436.
Chorus, C. G. (2010). A new model of random regret min-
imization. European Journal of Transport and Infras-
tructure Research, 10(2).
Chorus, C. G., Arentze, T. A., and Timmermans, H. J.
(2008). A random regret-minimization model of travel
choice. Transportation Research Part B: Methodolog-
ical, 42(1):1–18.
Ding, C., Chen, Y., Duan, J., Lu, Y., and Cui, J. (2017). Ex-
ploring the influence of attitudes to walking and cy-
cling on commute mode choice using a hybrid choice
model. Journal of advanced transportation, 2017.
Dong, B., Ma, X., Chen, F., and Chen, S. (2018). Investigat-
ing the differences of single-vehicle and multivehicle
accident probability using mixed logit model. Journal
of Advanced Transportation, 2018.
Golshani, N., Shabanpour, R., Auld, J., and Moham-
madian, A. (2018). Activity start time and dura-
tion: incorporating regret theory into joint discrete–
continuous models. Transportmetrica A: transport
science, 14(9):809–827.
Hensher, D. A., Greene, W. H., and Chorus, C. G. (2013).
Random regret minimization or random utility max-
imization: an exploratory analysis in the context of
automobile fuel choice. Journal of Advanced Trans-
portation, 47(7):667–678.
Ilahi, A., WARO, A. I., and Sumarsono, P. (2015). Public
transport reform in indonesian cities. In Proceedings
of the Eastern Asia Society for transportation stud-
ies, volume 10. Eastern Asia Society for Transporta-
tion Studies.
Irawan, M. Z., Belgiawan, P. F., Widyaparaga, A., Budiman,
A., Muthohar, I., Sopha, B. M., et al. (2018). A mar-
ket share analysis for hybrid cars in indonesia. Case
studies on transport policy, 6(3):336–341.
Irawan, M. Z., Putri, M. K., Belgiawan, P. F., and Dwitasari,
R. (2017). Analyzing commuters’ behavior on egress
ICASESS 2019 - International Conference on Applied Science, Engineering and Social Science
198