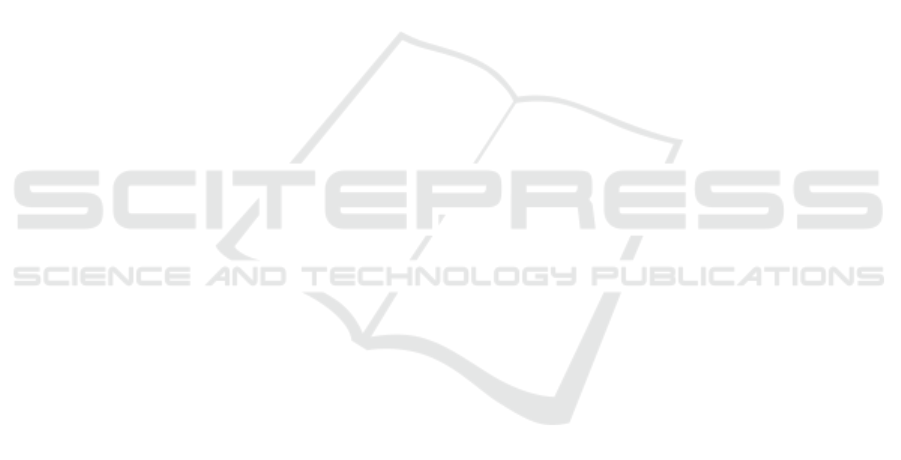
stimulation frequencies (or even more) in the range
between 13 and 17 Hz (Allison et al., 2010; Ehlers et
al., 2012; Stawicki et al., 2018). Individual frequency
sets that may improve processing accuracy of high
frequency SSVEP BCIs could not be established
during this study. However, it is to be noted that we
excluded numerous frequencies from our screening
(31, 33, 35, 37, 39, 41, 43, 45 Hz) due to the overall
duration of a session. Considering the selectivity of
cortical responses to IPS, it cannot be ruled out to
identify further resonance frequencies above 30 Hz.
Due to only two stable and recurring resonance
frequencies so far (32 & 40 Hz), high frequency based
BCI usage will continue to presuppose individual
calibration beforehand. However, for multimodal
interaction concepts that include various
physiological input options (e.g. eye movements), the
application of 32 and 40 Hz stimulation may provide
a further promising communication channel.
ACKNOWLEDGEMENTS
The current research has received funding from the
European Community's Seventh Framework
Programme under grant agreement No 224156. The
authors express their gratitude to all volunteers,
especially the tenants of the Cedar Foundation. We
sincerely thank Melanie Ware and Alexander
McRoberts from the University of Ulster for the
smooth cooperation during the patient testing.
REFERENCES
Allison, B., Luth, T., Valbuena, D., Teymourian, A.,
Volosyak, I. and Graser, A. (2010). BCI Demographics:
How Many (and What Kinds of) People Can Use an
SSVEP BCI? IEEE Transactions on Neural Systems
and Rehabilitation Engineering, 18(2), pp.107-116.
Başar, E., Emek-Savaş, D., Güntekin, B. and Yener, G.
(2016). Delay of cognitive gamma responses in
Alzheimer's disease. NeuroImage: Clinical, 11, pp.106-
115.
Busch, N., Debener, S., Kranczioch, C., Engel, A. and
Herrmann, C. (2004). Size matters: effects of stimulus
size, duration and eccentricity on the visual gamma-
band response. Clinical Neurophysiology, 115(8),
pp.1810-1820.
Chabuda, A., Durka, P. and Zygierewicz, J. (2018). High
Frequency SSVEP-BCI With Hardware Stimuli
Control and Phase-Synchronized Comb Filter. IEEE
Transactions on Neural Systems and Rehabilitation
Engineering, 26(2), pp.344-352.
Chatrian, G., Lettich, E. and Nelson, P. (1985). Ten Percent
Electrode System for Topographic Studies of
Spontaneous and Evoked EEG Activities. American
Journal of EEG Technology, 25(2), pp.83-92.
Ehlers, J., Valbuena, D., Stiller, A. and Gräser, A. (2012).
Age-Specific Mechanisms in an SSVEP-Based BCI
Scenario: Evidences from Spontaneous Rhythms and
Neuronal Oscillators. Computational Intelligence and
Neuroscience, pp.1-9.
Friman, O., Luth, T., Volosyak, I. and Gräser, A. (2007).
Spelling with steady-state visual evoked potentials. In
Neural Engineering, 2007. CNE'07. 3rd International
IEEE/EMBS Conference on IEEE, pp.354-357.
Herrmann, C. (2001). Human EEG responses to 1-100 Hz
flicker: resonance phenomena in visual cortex and their
potential correlation to cognitive phenomena.
Experimental Brain Research, 137(3-4), pp.346-353.
Herrmann, C., Mecklinger, A. and Pfeifer, E. (1999).
Gamma responses and ERPs in a visual classification
task. Clinical Neurophysiology, 110(4), pp.636-642.
Jin, Y., Castellanos, A., Solis, E. and Potkin, S. (2000).
EEG Resonant Responses in Schizophrenia: a Photic
Driving Study with Improved Harmonic Resolution.
Schizophrenia Research, 44(3), pp.213-220.
Mandel, C., Lüth, T., Laue, T., Röfer, T., Gräser, A. and
Krieg-Brückner, B. (2009). Navigating a smart
wheelchair with a brain-computer interface interpreting
steady-state visual evoked potentials. Intelligent Robots
and Systems, 2009. IROS 2009. IEEE/RSJ International
Conference on IEEE, pp. 1118-1125.
Makeig, S. (2002). Dynamic Brain Sources of Visual
Evoked Responses. Science, 295(5555), pp.690-694.
Molina, G. G., Ibanez, D., Mihajlović, V. and Chestakov,
D. (2009). Detection of high frequency steady state
visual evoked potentials for brain-computer interfaces.
17th European Signal Processing Conference. IEEE,
pp. 646-650.
Müller, S. M. T., Diez, P. F., Bastos-Filho, T. F., Sarcinelli-
Filho, M., Mut, V., Laciar, E. and Avila, E. (2015).
Robotic wheelchair commanded by people with
disabilities using low/high-frequency ssvep-based BCI.
World Congress on Medical Physics and Biomedical
Engineering, pp. 1177-1180.
Pastor, M., Artieda, J., Arbizu, J., Valencia, M. and
Masdeu, J. (2003). Human Cerebral Activation during
Steady-State Visual-Evoked Responses. The Journal of
Neuroscience, 23(37), pp.11621-11627.
Schalk, G., McFarland, D., Hinterberger, T., Birbaumer, N.
and Wolpaw, J. (2004). BCI2000: A General-Purpose
Brain-Computer Interface (BCI) System. IEEE
Transactions on Biomedical Engineering, 51(6),
pp.1034-1043.
Stawicki, P., Gembler, F. and Volosyak, I. (2016). Driving
a Semiautonomous Mobile Robotic Car Controlled by
an SSVEP-Based BCI. Computational Intelligence and
Neuroscience, 2016, pp.1-14.
Volosyak, I., Valbuena, D., Malechka, T., Peuscher, J. and
Gräser, A. (2010). Brain–computer interface using
water-based electrodes. Journal of Neural Engineering,
7(6), p.066007.
High Frequency Steady-State Visual Evoked Potentials: An Empirical Study on Re-test Stability for Brain-Computer Interface Usage
169