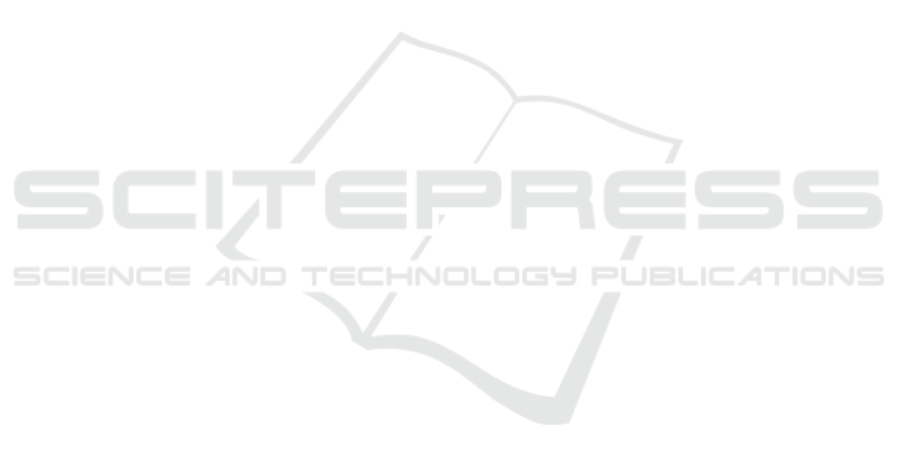
ing or partial knowledge.
• (M. Snoussi, 2014) propose a classification that
concerns a large set of imperfections that was built
specifically to characterize spatial data. This tax-
onomy distinguishes three types of imperfection:
Imprecision occurs when the true value is located
in a defined subset of values, inconsistency is the
conflict or incoherence in the values and uncer-
tainty is the partial knowledge about the true value
of information.
• (Sta, 2016) proposes several types of imperfect
data during the process of information retrieval
and data integration in smart cities. This im-
perfection can have several forms: Uncertain in-
formation reflects the lack of knowledge, impre-
cision information translates the non-specificity,
vague information reflects an ambiguous infor-
mation and missing information reflects the not
found or incomplete information.
2.3 Discussion
There is a big number of typologies of data im-
perfection. Authors such as (Niskanen, 1989) and
(Bouchon-Meunier, 1995) start by proposing generic
typologies. Then, to respond to several domains, au-
thors such as (Fisher, 2005), (Desjardin et al., 2012)
and (Sta, 2016) propose typologies for specific fields.
However, some correspond better to a reality than
others. Also, there is not a definitive terminology
and definition of terms used to qualify imperfect data,
such as uncertainty and imprecision. We also note
that the majority of these typologies share three com-
mon concepts which are the imprecision, the uncer-
tainty and the incompleteness. Imperfection types
are interdependent. According to (Bouchon-Meunier,
1995), ”Incompleteness leads to uncertainties, im-
precision can also be associated with incomplete-
ness and they generate uncertainties during their han-
dling”. According to (Smets, 1998), imprecision al-
ways refers to incompleteness. Imprecision may be
the source of uncertainty, but not necessarily (Smets,
1998).
Temporal data can have more imperfections com-
pared to the ones proposed in the generic typologies
because of the complexity of this type of data and the
specificity that it contains (i.e., It can be numeric or
natural language-based. It can also depend on the
general context of the information that contains the
temporal data and they may also be subject to several
factors such as the multiculturalism). Thus, generic
typologies cannot be adapted to temporal data (i.e,
they are inadequate). For instance, if we have the in-
formation ”I forget when last time I visited my uncle
who lives in Japan ”, the temporal data indicates a
”Missing” which is a type of imperfection that no one
of the generic typologies contains. Another example
is ”The first day of the week, we will have a meeting”.
In this example the temporal data indicates a ”circum-
locution” which is another type of imperfection that
no one of the generic typologies contains. Finally, to
the best of our knowledge, there is no typologies ded-
icated to temporal data imperfection.
3 OUR TYPOLOGY OF
TEMPORAL DATA
IMPERFECTION
In this section, we introduce our typology of tempo-
ral data imperfections illustrated by Figure 1 . Our
typology is based on the studied mentioned typolo-
gies and collected real examples. We divide our ty-
pology into direct imperfections, indirect imperfec-
tions and granularity. The direct ones are those which
can be deduced directly from the given data: uncer-
tainty, typing error, imprecision, missing and useless-
ness. The Indirect imperfections are those that can be
deduced from the direct ones. We distinguish three
types of indirect imperfections: (i) The incoherence
can be generated from the uncertainty and typing er-
ror. (ii) The incompleteness can be generated from
the imprecision and the missing. (iii) The redundancy
can be generated from the uselessness. Granularity is
the general context of the given temporal data which
can determine the type of imperfection.
3.1 Direct Imperfection Types
We distinguish seven direct imperfection types: un-
certainty, typing error, imprecision, missing, circum-
locution and uselessness which will be detailed one
by one and illustrated by examples.
• Uncertainty. According to (Smets, 1999), each
statement is whether true or false. However, the
knowledge about the statement, if it is uncertain,
does not allow you to decide either it is true or
false. Uncertainty is partial knowledge of the true
value of the data. It is the lack of information.
In our typology, uncertainty has two categories.
The first category includes uncertain data that re-
spect the rules of common sense. It can be due
to a doubt. As an example, ”I’m not sure if it
was the last summer or the one before when my
aunt came from Paris to spend the holidays with
us”. In this example, the person is doubting about
the given temporal data. However, the latter re-
A Typology of Temporal Data Imperfection
307