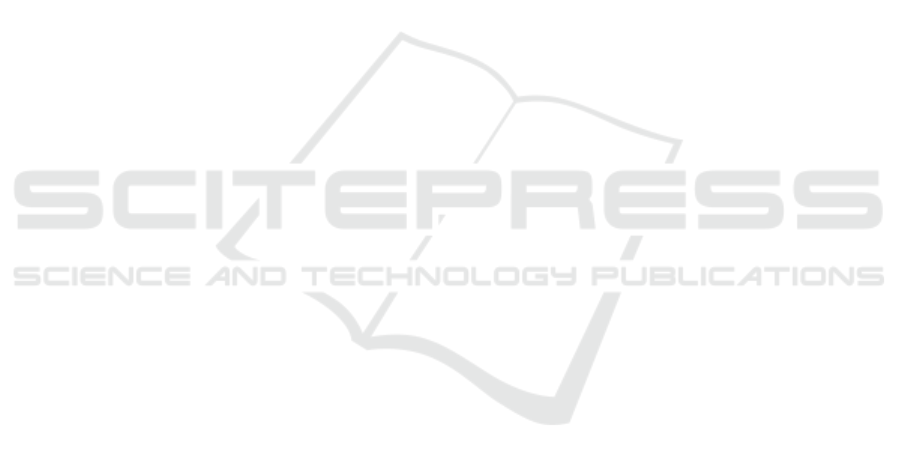
(2015)) that help to make use of implicitly deduced
or predicted and potential social ties.
Finally, researchers should try to identify new le-
gal, sustainable, and innovative ways to access social
media data. These include buying social media data,
collaboration with other researchers in gaining nec-
essary social media data, and also combining addi-
tional data and other data sets to existing social media
data. For example, making better use of location and
co-location data and combining it with existing social
media data to make new social tie strength models.
6.2 Limitations and Future Research
This study has certain limitations. Firstly, this study
only focused on analyzing the changes to data ac-
cess policy of two social media platform - Facebook
and Twitter and not the other social media platforms.
Secondly, this study did not explain the exact techni-
cal details of the limitations introduced by the differ-
ent social media API versions(e.g., exact rate limits,
exact accessibility of different data items). Finally,
this study did not analyze how the new social media
platform feature changes (e.g., increase in the Tweet
length from 140 to 280 characters) can impact the cur-
rent social tie strength models.
This study leaves room for future studies. First,
in the future, a similar study could be done for some
other popular social media platforms Second, a study
about how the current social tie strength models are
adapted to the current data access situation. Finally,
do new studies to develop tie strength models which
can deduce social relationships(implicit relationship)
using just publicly available social media comments
and discussions.
REFERENCES
Burgess, J. and Bruns, A. (2012). Twitter archives and the
challenges of “big social data” for media and commu-
nication research. M/C J., 15.
Burgess, J. and Puschmann, C. (2014). Twitter and Soci-
ety: The Politics of Twitter. In Weller, K., Bruns, A.,
Marth, M., and Puschmann, C., editors, Twitter and
society, volume 89, chapter 4, pages xxix–xxxviii. Pe-
ter Lang Inc.
De Meo, P., Ferrara, E., Fiumara, G., and Provetti, A.
(2014). On Facebook, most ties are weak. Commu-
nications of the ACM, 57(11):78–84.
Fogues, R. L., Such, J. M., Espinosa, A., and Garcia-Fornes,
A. (2018). Tie and tag: A study of tie strength and tags
for photo sharing. PLoS ONE, 13(8):e0202540.
Gilbert, E. (2012). Predicting tie strength in a new medium.
In CSCW ’12, page 1047. ACM.
Gilbert, E. and Karahalios, K. (2009). Predicting tie
strength with social media. In CHI 09, page 211.
ACM.
Granovetter, M. S. (1973). The Strength of Weak Ties.
American Journal of Sociology, 78(6):1360–1380.
Gupta, J. P., Menon, K., K
¨
arkk
¨
ainen, H., Huhtam
¨
aki, J.,
Mukkamala, R. R., Hussain, A., Vatrapu, R., Jussila,
J., and Pirkkalainen, H. (2016). Identifying weak ties
from publicly available social media data in an event.
In AcademicMindtrek ’16, pages 11–19. ACM.
Hogan, B. (2018). Social media giveth, social media taketh
away: Facebook, friendships, and APIs. International
Journal of Communication, 12(0):592–611.
Huang, H., Tang, J., Liu, L., Luo, J., and Fu, X. (2015). Tri-
adic Closure Pattern Analysis and Prediction in Social
Networks. IEEE TKDE, 27(12):3374–3389.
Huhtam
¨
aki, J. and Olsson, T. (2018). Digital Social Match-
ing Ecosystem for Knowledge Work. In KMIS 10,
pages 194–199. SCITEPRESS.
Jones, J. J., Settle, J. E., Bond, R. M., Fariss, C. J., Mar-
low, C., and Fowler, J. H. (2013). Inferring Tie
Strength from Online Directed Behavior. PLoS ONE,
8(1):e52168.
Kahanda, I. and Neville, J. (2009). Using transactional in-
formation to predict link strength in online social net-
works. ICWSM09, pages 74–81.
Liberatore, F. and Quijano-Sanchez, L. (2017). What do we
really need to compute the Tie Strength? An empirical
study applied to Social Networks. Comput Commun,
110:59–74.
Mattie, H., Engø-Monsen, K., Ling, R., and Onnela, J.-P.
(2018). Understanding tie strength in social networks
using a local “bow tie” framework. Scientific Reports,
8(1):9349.
Onnela, J.-P., Saram
¨
aki, J., Hyv
¨
onen, J., Szab
´
o, G., Lazer,
D., Kaski, K., Kert
´
esz, J., and Barab
´
asi, A.-L. (2007).
Structure and tie strengths in mobile communication
networks. PNAS, 104(18):7332–6.
Quijano-S
´
anchez, L., D
´
ıaz-Agudo, B., and Recio-Garc
´
ıa,
J. A. (2014). Development of a group recommender
application in a Social Network. Knowledge-Based
Systems, 71:72–85.
Xiang, R., Neville, J., and Rogati, M. (2010). Modeling re-
lationship strength in online social networks. In WWW
’10, page 981.
Zhang, B., K
¨
arkk
¨
ainen, H., Gupta, J. P., and Menon, K.
(2017). The role of weak ties in enhancing knowl-
edge work. In AcademicMindtrek ’17, pages 210–215.
ACM.
KMIS 2019 - 11th International Conference on Knowledge Management and Information Systems
194