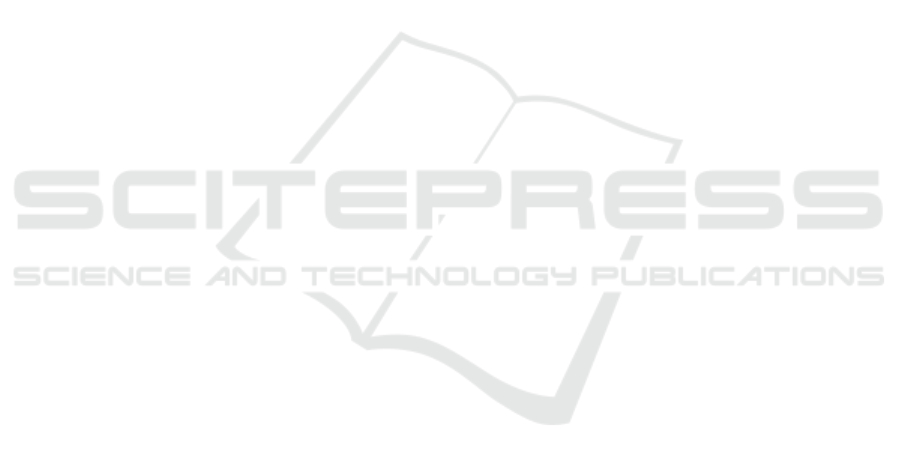
Sources of data within the classroom:
We think that all lecturers live for the discussions they
have with students in class, we certainly do. As good
as they can be, there are two potential problems with
class discussions. First, often only a small part
of the class will participate, and often the same
students each time. This can lead to a somewhat
limited number of perspectives in the discussion.
Second, even if the discussions are documented in a
lecture recording, the students participating are not
wearing microphones, so viewers of the recording
will not be able to hear the student part of the
discussion. In lectures that are not recorded, the
discussions will be unavailable for those students not
attending the physical lecture, and at best very hard to
recall for those attending and participating. (We
suspect that most of these will have forgotten the
whole discussion within days.)
If there were a way to structure and document
those discussions, there would be two obvious
benefits. For the student, the documentation would be
useful for repetition. For the lecturer, the
documentation would be available, and useful, when
preparing for the next time doing the same course. It
would be easier to assess if the questions were any
good for making the intended point during the lecture
and improving the structure of the lecture if
necessary. We propose the following ways to gather
data within the classroom:
1. Working on Assignments on a Shared
Document
We have several years' experience using MS
OneNote for this purpose. The platform is in no way
perfect or without its technical flaws; in fact we have
considered changing it several times. Still it is good
enough to both document the discussions and capture
more perspectives from the students. It is easy to
access without logging into a service, but as many
institutions now have Office365 for their students and
staff, logging in is also an option that will make it
easier to trace each student’s activity.
Practically, students are given an assignment for
discussion during the lecture, and a timeframe for
solving and writing down the answer, usually 5 or 10
minutes. In OneNote they will create a tab (in
OneNote this is called a page) with their names as
a heading and write down the answer on the page.
This answer will be available for all the other
students, and the lecturer, to see. The answers then
make the basis for the discussion, making it possible
for the lecturer to reach out to specific students by
name to start the discussion.
This way of data collection has proven to
constitute a valuable archive for both lecturer and
students.
2. Micro-surveys at the End of Each Lecture
Overwhelmingly long, non-compulsory evaluations
are not very appealing to anyone. This also applies to
students, and as mentioned before there are a lot of
sources of bias and error that affect the outcome of
those evaluations. The timing is one of them, right
before exams is a stressful time for
students that might affect the evaluation, and after the
exam, the grading can affect the evaluations both
positively and negatively. It is also hard to remember
specific classes and topics, so what is measured is
more a general impression. Thus, end-of-semester
evaluation results might not be the best data for
optimizing the learning and the environment in which
it occurs. At best, the next class will reap the benefits,
not the class doing the evaluations. We propose the
following:
We designed a framework for micro-surveys to serve
two purposes. First, to give the students a couple of
minutes for reflection at the end of the lecture.
Second, and most importantly for learning analytics,
give the students a chance to evaluate the lecture in
two quick ways: A 1 - 5 -star rating on how much
understanding they have for central topics presented
during the lecture, usually 4 or 5 topics, and a text
field where they can write down what is most unclear
to them after the lecture. In addition to this there is
also a field where the students can give general
comments to the lecture.
Many times, one or two of the topics will get a
lower rating than the others. Repetition of these
topics will be a good starting point for the next
lecture, and the same goes for discussing some
answers from the text field of unclear topics after the
lecture. The answers in the text field will help
uncover other topics for repetition than the pre-
defined topics that are star-rated.
Practically, this short survey is done with Forms
in Office365, and the students are given a link to the
form in the last picture for the lecture notes, where it
is also possible to access the survey on a mobile
device via a QR-code. The results from the previous
lecture will be presented in the beginning of the
lecture notes for the next lecture. Again, the data
collected makes it possible to improve the following
Can Digital Footprints Save the Physical Lecture?
463