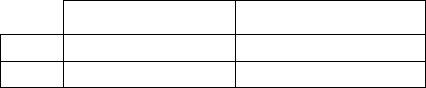
Table 5 below. Thus, for high-magnitude war only
defensive strategies generate bounded results.
Table 5: Pseudo q-values for log-gamma and log-normal
exceedance probabilities based on indicated COW deaths.
Attacker(s) Defender(s)
Win q=0.83,0.8Mtotal q=1.2,17Mtotal
Lose q=0.5,5.4Mtotal q=0.78,6.Mtotal
5 CONCLUSION
Risk of military attack, in terms of combat fatalities,
can be minimized using a Bayesian detection criteria
based on prior probability distributions derived from
COW Inter-State war data. Use of the power-law in
this context is invalid and should be abandoned. A
game-theoretic model with two Nash Equilibrium
points help explain why combatant fatalities follow a
log-gamma or log-normal probability distribution
depending on if a state is offensive or defensive. De-
correlating combat fatalities from alliance effects
exposes the log-gamma structure of the defend-lose
case and enables a calculation of attack risk per year
for ranges of deaths in powers of ten. Further, the data
indicate that war occurs with predictable temporal
frequency where the likelihood of one or more wars
in a year follows the Poisson distribution. After being
initiated, a war escalates or deescalates proportional
to the combat losses already incurred. The data also
shows that the risk of nuclear war level fatalities
increases despite decreasing in probability. Taking
into account detection and false-alarm probabilities,
an LRT advises that it is rational to escalate only
when the consequence of inaction and action are
about equal in magnitude, corresponding to nominal
false-alarm maxima. A corollary is that act-on-
warning is justified only if the detection system
indicates an upper limit of impending fatalities.
Lastly, only defensive strategies have a convergent
mean for wars having fatalities greater 10
8
.
In future work, a Bayesian detection criteria could
be applied to automated detection of cyber-attack,
informed by the correct prior and taking into account
both positive and negative consequences.
REFERENCES
Advisory Committee on Reactor Safeguards, 2000.
Proceedings of the United States Nuclear Regulatory
Commission Advisory Committee on Reactor
Safeguards.
Benguigui, L. & Marinov, M., 2015. A Classification of
Natural and Social Distributions Part one: The
Descriptions. arXiv:1607.008556v1 [physics.soc-ph]
Cederman, L., 2003. Modeling the Size of Wars: From
Billiard Balls to Sandpiles. The American Political
Science Review, 97.1 (April 2015), p. 135
Freedman, L., 2017. The Future of War: A History. New
York, NY. Hachette Book Group.
Halliwell, L. J., 2018. The Log-Gamma Distribution and
Non-Normal Error. Variance (Accepted for
Publication).
Jackson, M. O. & Nei, S., 2015. Networks of Military
Allliances, Wars, and International Trade. PNAS,
112(50), pp. 15277 - 15284.
Li, J., 1994. Synthetic Aperature Radar Target Detection,
Feature Extraction, and Image Formation Techniques,
Gainesville, Florida. NASA.
Nash, J. F., 1950. Equilibrium Points in N-Person Games.
Proceedings of the National Academy of Sciences,
36(1), pp. 48-49.
Ott, W. R., 1990. A Physical Explanation of the
Lognormality of Pollutant Concentrations. Air and
Waste Management Association, 40(10), pp. 1378 -
1383.
Richardson, L. F., 1960. The Statistics of Deadly Quarrells.
The Boxwood Press, Pittsburgh.
Sarkees, M. R. a. F. W., 2010. Resort to War: 1816 - 2007.
Washington DC. CQ Press.
Sornette, D., 2007. Probability Distributions in Complex
Systems. Review article for the Encyclopedia of
Complexity and System Science ed. Zurich. Springer
Science.
Sox, H. C., Higgins, M. C. & Owens, D. K., 2013. Medical
Decision Making. 2nd ed. The Atrium, Southern Gate,
Chichester, West Susssex, UK. Wiley-Blackwell.
U.S. Dept. of Defense, 2018. U.S. Nuclear Posture Review.
Washington D.C.: U.S. Government.
Clausewitz, C., 1976. On War. Suffolk, Great Britain.
Princeton University Press.
DISCLAIMER
The opinions, conclusions, and recommendations
expressed or implied are the authors’ and do not
necessarily reflect the views of the Department of
Defense or any other agency of the U.S. Federal
Government, or any other organization.