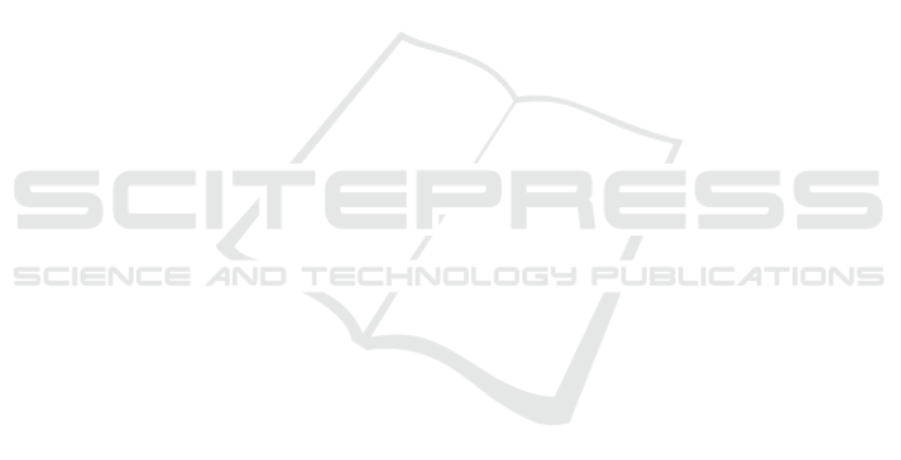
Ekman, P. and Friesen, W. V. (1969). Nonverbal leakage
and clues to deception. Psychiatry, 32(1):88–106.
Ekman, P. and Friesen, W. V. (1971). Constants across cul-
tures in the face and emotion. Journal of personality
and social psychology, 17(2):124.
Endres, J. and Laidlaw, A. (2009). Micro-expression recog-
nition training in medical students: a pilot study. BMC
medical education, 9(1):47.
Frank, M., Herbasz, M., Sinuk, K., Keller, A., and Nolan,
C. (2009). I see how you feel: Training laypeople and
professionals to recognize fleeting emotions. In The
Annual Meeting of the International Communication
Association. Sheraton New York, New York City.
Grobova, J., Colovic, M., Marjanovic, M., Njegus, A., De-
mire, H., and Anbarjafari, G. (2017). Automatic hid-
den sadness detection using micro-expressions. In Au-
tomatic Face & Gesture Recognition (FG 2017), 2017
12th IEEE International Conference on, pages 828–
832. IEEE.
Guo, Y., Xue, C., Wang, Y., and Yu, M. (2015). Micro-
expression recognition based on cbp-top feature with
elm. Optik-International Journal for Light and Elec-
tron Optics, 126(23):4446–4451.
Haggard, E. A. and Isaacs, K. S. (1966). Micromomentary
facial expressions as indicators of ego mechanisms in
psychotherapy. In Methods of research in psychother-
apy, page 154–165. Springer.
He, J., Hu, J.-F., Lu, X., and Zheng, W.-S. (2017). Multi-
task mid-level feature learning for micro-expression
recognition. Pattern Recognition, 66:44–52.
Hus
´
ak, P.,
˘
Cech, J., and Matas, J. (2017). Spotting facial
micro-expressions ”in the wild”. In Proceedings of
the 22nd Computer Vision Winter Workshop. Pattern
Recognition and Image Processing Group (PRIP) and
PRIP club.
LI, J., Soladi
´
e, C., and S
´
eguier, R. (2018). Ltp-ml: Micro-
expression detection by recognition of local temporal
pattern of facial movements. In Automatic Face &
Gesture Recognition (FG 2018), 2018 13th IEEE In-
ternational Conference on, pages 634–641. IEEE.
Li, X., Pfister, T., Huang, X., Zhao, G., and Pietik
¨
ainen,
M. (2013). A spontaneous micro-expression database:
Inducement, collection and baseline, page 1–6. IEEE.
Li, X., Xiaopeng, H., Moilanen, A., Huang, X., Pfister, T.,
Zhao, G., and Pietikainen, M. (2017). Towards rea-
ding hidden emotions: A comparative study of sponta-
neous micro-expression spotting and recognition met-
hods. IEEE Transactions on Affective Computing.
Mavadati, S. M., Mahoor, M. H., Bartlett, K., Trinh, P.,
and Cohn, J. F. (2013). Disfa: A spontaneous facial
action intensity database. IEEE Transactions on Af-
fective Computing, 4(2):151–160.
Oh, Y.-H., See, J., Le Ngo, A. C., Phan, R. C.-W., and Bas-
karan, V. M. (2018). A survey of automatic facial
micro-expression analysis: Databases, methods and
challenges. Frontiers in Psychology, 9:1128.
Pfister, T., Li, X., Zhao, G., and Pietik
¨
ainen, M. (2011). Re-
cognising spontaneous facial micro-expressions. In
Computer Vision (ICCV), 2011 IEEE International
Conference on, pages 1449–1456. IEEE.
Polikovsky, S. (2009). Facial micro-expressions recognition
using high speed camera and 3d-gradients descriptor.
In Conference on Imaging for Crime Detection and
Prevention, 2009, volume 6.
Qu, F., Wang, S.-J., Yan, W.-J., Li, H., Wu, S., and Fu,
X. (2017). Cas (me)ˆ 2: a database for spontaneous
macro-expression and micro-expression spotting and
recognition. IEEE Transactions on Affective Compu-
ting.
Radlak, K., Bozek, M., and Smolka, B. (2015). Silesian de-
ception database: Presentation and analysis. In Pro-
ceedings of the 2015 ACM on Workshop on Multimo-
dal Deception Detection, pages 29–35. ACM.
Shreve, M., Godavarthy, S., Goldgof, D., and Sarkar,
S. (2011). Macro-and micro-expression spotting in
long videos using spatio-temporal strain, page 51–56.
IEEE.
Vinciarelli, A., Dielmann, A., Favre, S., and Salamin, H.
(2009). Canal9: A database of political debates for
analysis of social interactions. In Affective Compu-
ting and Intelligent Interaction and Workshops, 2009.
ACII 2009. 3rd International Conference on, pages 1–
4. IEEE.
Wang, S.-J., Yan, W.-J., Sun, T., Zhao, G., and Fu, X.
(2016). Sparse tensor canonical correlation analysis
for micro-expression recognition. Neurocomputing,
214:218–232.
Warren, G., Schertler, E., and Bull, P. (2009). Detecting de-
ception from emotional and unemotional cues. Jour-
nal of Nonverbal Behavior, 33(1):59–69.
Weber, R., Soladi
´
e, C., and S
´
eguier, R. (2018). A survey
on databases for facial expression analysis. In Pro-
ceedings of the 13th International Joint Conference
on Computer Vision, Imaging and Computer Graphics
Theory and Applications - Volume 5: VISAPP,, pages
73–84. INSTICC, SciTePress.
Xiaohua, H., Wang, S.-J., Liu, X., Zhao, G., Feng, X., and
Pietikainen, M. (2017). Discriminative spatiotemporal
local binary pattern with revisited integral projection
for spontaneous facial micro-expression recognition.
IEEE Transactions on Affective Computing.
Yan, W.-J., Li, X., Wang, S.-J., Zhao, G., Liu, Y.-J., Chen,
Y.-H., and Fu, X. (2014). Casme ii: An improved
spontaneous micro-expression database and the base-
line evaluation. PloS one, 9(1):e86041.
Yan, W.-J., Wu, Q., Liu, Y.-J., Wang, S.-J., and Fu, X.
(2013). CASME database: a dataset of spontane-
ous micro-expressions collected from neutralized fa-
ces, page 1–7. IEEE.
Yap, M. H., See, J., Hong, X., and Wang, S.-J. (2018).
Facial micro-expressions grand challenge 2018 sum-
mary. In Automatic Face & Gesture Recognition (FG
2018), 2018 13th IEEE International Conference on,
pages 675–678. IEEE.
Zhang, X., Yin, L., Cohn, J. F., Canavan, S., Reale, M.,
Horowitz, A., Liu, P., and Girard, J. M. (2014). Bp4d-
spontaneous: a high-resolution spontaneous 3d dyn-
amic facial expression database. Image and Vision
Computing, 32(10):692–706.
VISAPP 2019 - 14th International Conference on Computer Vision Theory and Applications
248