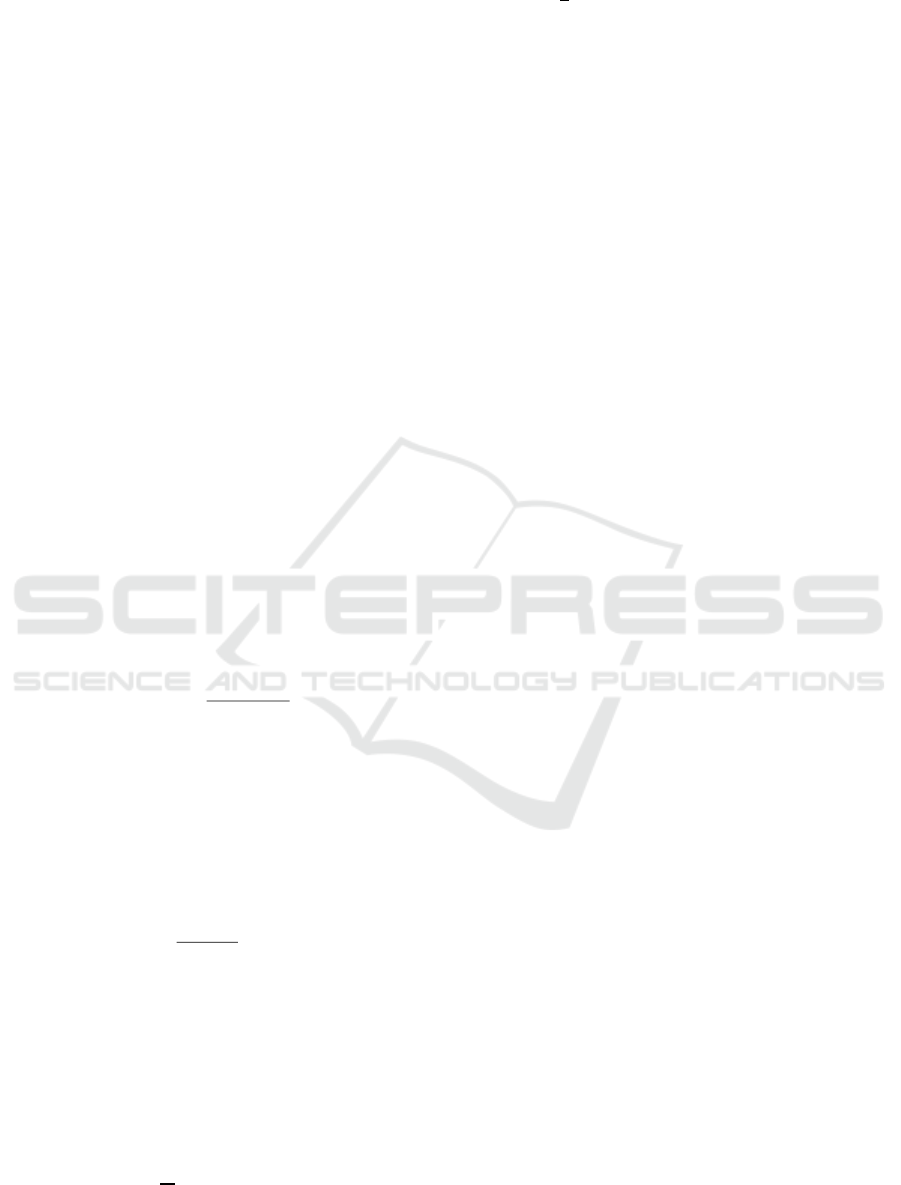
xxg
10
(x) - 1
(x)
ln )(
+=
=
lecturer are State Civil Apparatus (SCA), Internal
Lecturer Not SCA (NSCA), and External Lecturer
(EL), assessment components of LPI consist of
Department’s Assessment and Student’s
Assessment.
A data set also lists categories of lecturers in
IAIN Purwokerto that number of State Civil
Apparatus (SCA) are 131 lecturers included 26 no
fine and 105 fine, Internal Lecturer Not SCA
(NSCA) are 50 lecturers included 7 no fine and 43
fine, and External Lecturer (EL) are 39 lecturers
included 22 no fine and 17 fine. As for data of 201
lecturers for the department’s assessment and
student’s assessment that each includes 22 lecturers
in no fine category and 179 are fine.
Finally, this paper organize by the second section
is the basic theories and later, analyze the data set by
binary logistic regression. Data representation and
visualization are presented, also the interpretation of
data analysis is done. Conclusion will finish in the
analysis of the IAIN Purwokerto lecturer’s data.
2 THE BASIC THEORIES
2.1 Logistic Regression
The specific form of the logistic regression model
uses as below
(1)
where π(x) = E(Y|x) represent the conditional mean
of Y given x when the logistic distribution is used
(Hyeoun-Ae, 2013).
A transformation of π(x) that is central to study
logistic regression is the logit transformation. This
transformation is defined in terms of π(x) as
(2)
The importance of this transformation is that
has many of the desirable properties of a linear
regression model (Tranmer and Elliot, 2008;
Thomsen and Lundbeck, n.d.). The endpoints of a
100(1 - α)% confidence interval for slope coefficient
are
(3)
where
is upper 100(1-α/2)% point from the
standard normal distribution.
The reason to use the logistic regression in this
data analysis are logistic regression do not require a
linear relationship between independent and
dependent variables, the independent variable does
not require the multivariate normality assumptions,
the independent variable can be in terms of continue
and category data, dependent variable must be
dichotomy that is two categories, and samples
required in relatively large quantities, minimum
required up to 50 data samples for an independent
variable. In this research use more than 50 data to be
analyzed.
2.2 Performance
The person’s performance can be influenced by
internal and external factors. Internal factors
included psychic and physical. Physical component
for example health, gender, age, and external factors
included salary, working conditions, work
relationship, position (Handoko, 2002). IAIN
Purwokerto has done an assessment of LPI five
times from 2015 until 2018, only once in 2018. IAIN
Purwokerto also declares that LPI < 3.00 is no fine
and LPI ≥ 3.00 is fine. IAIN Purwokerto has three
categories of lecturer since 2017 that is State Civil
Apparatus (SCA), Internal Lecturer Not SCA
(NSCA), and External Lecturer (EL) (Tim, 2016).
Department’s Assessment includes four components
in IAIN Purwokerto. A way to evaluate based on the
time interval, when 1) lecturer give the planning of
learning, 2) lecturer give the question of final exam
of the semester, 3) lecturer give the final exam score,
and 4) how many lecturers teach students in one
semester. Department’s assessment score is made in
scale 0 - 4 (Tim, 2016). Student’s assessment is
obtained from the evaluation of the lecturer learning
in each course class by students. A way to evaluate
based on the results of a questionnaire given to
students. The score of the student’s assessments is
also made in scale 0 - 4 too.
3 RESULTS OF THE DATA
ANALYSIS
When faced with a set of data, a person will play
around with them, an activity called (explanatory)
data analysis. The activity is elementary calculation
will be made, simple graphs will be plotted, and also
table will be made (Dennis, 2001). Based on the
ICMIs 2018 - International Conference on Mathematics and Islam
266