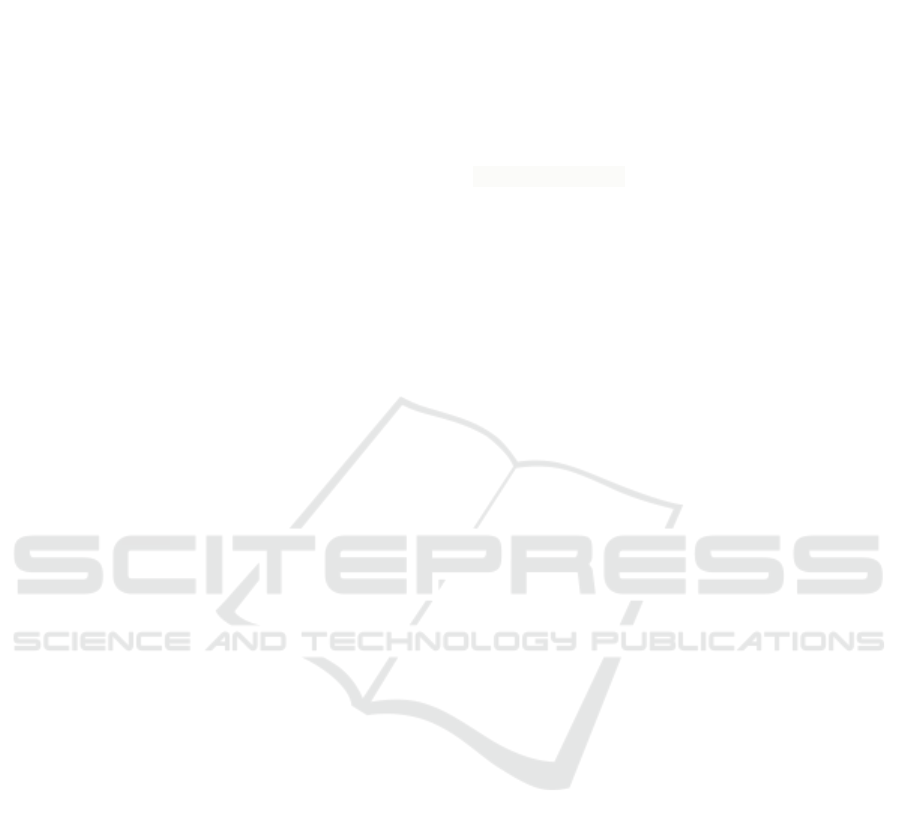
4 CONCLUSIONS
The result of modelling of sample BOD level using
cokriging method obtained by the best model based
on the smallest RSS value of 2838 is Gaussian model.
The value of
is very high of 91.5% and small MSE
value of 0.7057. This shows that the interpolation
results are accurate with the Gaussian model. The
estimation result of BOD level in Surabaya River
shows that BOD level leading downstream of the
river is lower. This is because the source of pollutants
from the upstream of the river that leads downstream
of the river is less have an effect. The results show
that BOD levels at new locationaroundPT. A, namely
location 11 of 7.5 mg / l. This value is far exceeding
class II river water quality standard that has been set
that is 3 mg / l so it can be said that the location has
been contaminated status.Several factors cause high
levels of BOD in the location that is because the river
flow that still brings the influence of waste from the
previous location and also can be expected because
the location is close to PT. A which is the industry
with the dominant waste that is hydrargyrum (Hg),
cadmium (Cd), chromium (Cr), lead (Pb), and copper
(Cu).Then the estimation of BOD levels in the new
locationaroundPT. A, namely location 12 of 9.9 mg /
l. This value far exceeding class II river water quality
standard that has been set that is 3 mg / l so it can be
said that the location has been contaminated status.
Several factors cause high levels of BOD in that
location, which is due to river currents that still carry
the effect of waste from the previous location
including the waste of PT. A and may also be
suspected because the location of location 12 is close
to PT. B which is an industry with dominant waste,
namely organic matter, suspended solids (SS),
dissolved solids (DS) and Cd.
REFERENCES
Ahmadi, S. H., and Sedghamiz, A., 2008. Application and
Evaluation of Kriging and Cokriging Methods on
Groundwater Depth Mapping. Journal Science, 138:
357-368, DOI 10.1007/s10661-007-9803-2.
Atima, W., 2014. BOD dan COD sebagai Parameter
Pencemaran Air dan Baku Mutu Air Limbah. Jurnal
Biology Science and Education, 3(2), ISSN 2252-858X.
Fardiaz, S., 1992. POLUSI AIR & UDARA, Penerbit
KANISIUS, Yogyakarta.
Haq, A. Z., 2017. Awas, Jangan Sembarangan Makan Ikan
Wader dan Mujaer dari Sungai Surabaya, SURYA,
March 8, 2017.
Isaaks, H. E. and Srivastava, R. M., 1989. Applied
Geostatistics. Oxford University Press, New York
Kelkar, M. and Perez, G., 2002. Applied Geostatistics for
Reservoir Characterization. Society of Petroleum
Engineers, USA.
Kis, I. M., 2016. Comparison of Ordinary and Universal
Kriging interpolation techniques on a depth variable (a
case of linear spatial trend), the case study of the
Sandrovac Field. Rudenko – Geolosko – Naftni Zbornik,
31(2), 41.
LeMay, N. E., 1995.Variogram Modeling and Estimation.
Thesis Master of Science Applied Mathematics,
University of Colorado, Denver.
Tchobanoglous, G., 1991. Wastewater Engineering
Treatment, Disposal, and Reuse, McGraw-Hill Book
Company, New Delhi.
Priyono, T. S. C., Yuliani, E. and Sayekti, R. W., 2013.
Studi Penentuan Mutu Air Sungai Surabaya untuk
Keperluan Bahan Baku Air Minum. Jurnal Teknik
Pengairan, 4(1): 53-60.
Razif, M. and Masduqi, A., 1996. Penelitian Korelasi COD
dan BOD Limbah Cair untuk Monitoring Pencemaran
Kali Surabaya. Majalah IPTEK, Mei.
Suwari, 2010. Model Pengendalian Pencemaran Air Pada
Wilayah Kali Surabaya. Dissertation, Institut Pertanian
Bogor.
Trisnawati, A. and Masduqi, A., 2013. Analisis Kualitas
dan Strategi Pengendalian Pencemaran Air Kali
Surabaya. Jurnal Purifikasi, 14(2): 90-98.
Biochemical Oxygen Demand Level Modeling in Surabaya River using Approach of Cokriging Method
59