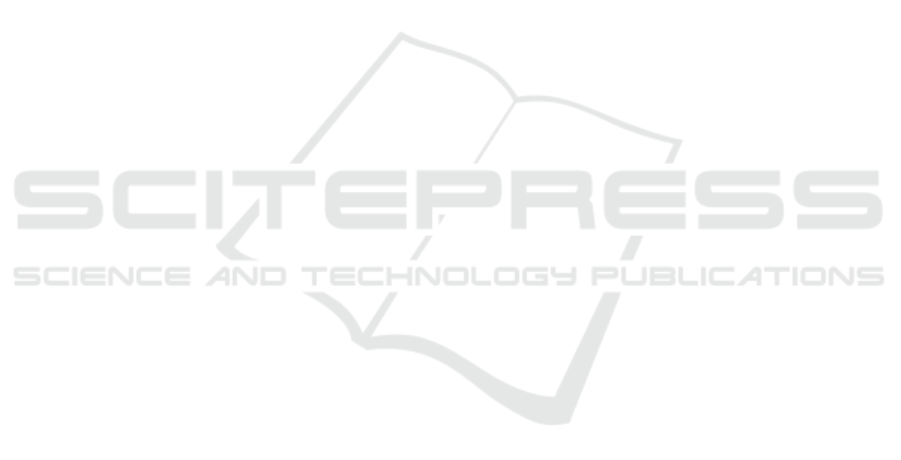
other words, every Indonesian citizen is considered
not poor if the income per capita per day of them is
Rp. 13,366 for urban-dweller and Rp. 12,363 for
rural areas-dweller (BPS, 2018). The national
average poverty line determined by BPS is higher
than the World Bank Purchasing Power Parity
standard (Novalia, 2018).
The calculation of poverty using the World
Bank’s PPP standard of US$ 1.9 per day is measured
using 2011 as its base year. The estimated
conversion of 1 US dollar in 2011 is
Rp11,157/capita/day, which then shifted to
Rp13,162/capita/day in 2018 (International
Monetary Fund, 2018).
Even Enny Sri Hartati, the Director of the
Institute for Development of Economics and Finance
(INDEF) stated: “The poverty rate of Indonesia
number could be doubled to 70 million people if the
USD 1.9/capita/day standard is used” (Suryowati
06/03/2018, https://www.jawapos.com/jpg-
today/06/03/2018/).
The statement comes from the result of
calculation using the applicable international
exchange rate. Using the international exchange rate
as of March 2018 (Rp. 13,761), the monthly poverty
standard is Rp. 784,377/capita/month, equivalent to
(Rp. 26,146/ capita/ day). It doubles the standard of
Purchasing Power Parity (PPP) used by BPS for
both urban and rural poverty standards (Suryowati,
Tuesday (6/3) JawaPos.com,
https://www.jawapos.com/jpg-today/06/03/2018/).
South Sumatera Province ranked the third in the
largest poor residents number in Sumatera, and
ranked the seventh in all Indonesia (BPS, 2018). The
number of poor people in South Sumatra Province is
beyond the percentage of the average number of
poor people in Indonesia. BPS reported in Semester
1 of 2018, the percentage of the poor population of
South Sumatra Province was 12.80 percent, or
exceed the average number of poor people in
Indonesia (9.82 percent). The condition has been
continuously occuring for the past four years, where
the percentage target of the number of poor people
in South Sumatra Province is always above the
national average of 7.5 percent. Therefore, further
research is needed in determining the factors
affecting the level of poverty in the regencies/cities
in South Sumatera Province. The results are
expected to provide as a reference in determining the
direction of poverty alleviating policies.
Research on the factors determining the number
of poor people has been widely carried out, both in
national and provincial scale. (Fajriyah & Rahayu,
2016) has conducted a modeling analysis of the
factors that influence poverty in the regencies/cities
in East Java Province with panel data regression,
which then revealed that the significant predictor
variables included literacy, labor force participation,
number of population working in the agricultural
sector, as well as GDRP per capita. Meanwhile,
predictor variables with no effect on the response
variable are residents without health access.
Furthermore, research related to poverty modeling is
carried out by (Zuhdiyati & Kaluge, 2015) with the
results showing that the HDI has a significant
negative effect on poverty and the open
unemployment rate has no significant effect.
However, previous research conducted by Yacoub
(2012) shows that the open unemployment rate has a
significant effect on poverty.
2 LITERATURE REVIEW
2.1 Poverty Definition
Poverty can be illustrated as a situation where
there is a lack of common things related with the life
quality, such as food, clothing, shelter and clean
water. Economically, poverty can be indicated by
the level of lack of resources in fulfilling the needs
of life and improve the welfare of a group of people.
According to (Suryawati, 2005) poverty can be
divided into four forms : 1) absolute poverty, where
the income is below the poverty line or not enough
to fulfill the standard needs of food, clothing, health,
shelter and education needed to maintain live and
gain job; 2) relative poverty, which caused by the
failure of development policies in reaching all the
layer of communities, causing inequality in income
among residents; 3) cultural poverty, refers to the
attitude matter of a person or community caused by
cultural factors, such as the reluctancy to improve
the level of life, wasteful, and not creative; and 4)
structural poverty, a poverty caused by minimum
access to resources that occur in socio-cultural and
political systems that do not support poverty
alleviation.
2.2 Measurement of Poverty
According to BPS, in 2018, the level of poverty
is based on the amount of rupiah consumption spent
for food, precisely 2.100 calories per person per day
(from 52 types of commodities representing the
consumption patterns of residents of lower-class),
and non-food consumption of 45 types foods
commodities in accordance with national agreements
Economic Growth, Social Expenditure, Unemployment, and Inflation: The Impact on Poverty in South Sumatera
627