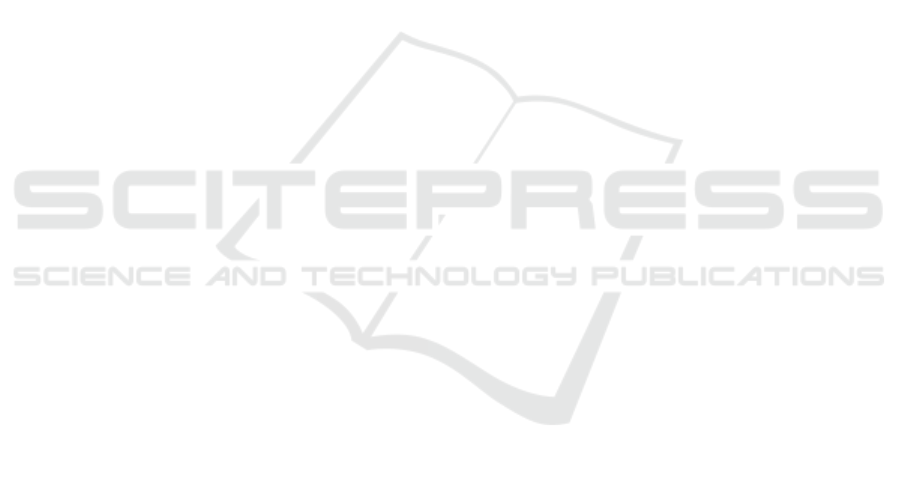
knowledge base with training data, respectively,
providing qualitative and quantitative assessments.
(ii) Reasoning and Knowledge Base (KB) is
composed of all available knowledge over which the
reasoning is performed. The Task FB input is used as
a trigger to start the inference process, which is based
on SWRL rules whereas the output of that process is
given as a series of axioms, representing detailed
results with practical, human readable data. (iii) Task
Rules comprises all SWRL rules created to infer
knowledge from training related instances. These
rules may be created or updated by several experts
from different domains. (iv) Domain Knowledge Base
refers to the application-independent axioms, which
can be updated to better cope with improvements in
the understanding of applicable fields. Knowledge
bases are implemented as ontologies, which were
divided into assertion axioms (i.e., Fact base; FB)
and terminological axioms (i.e., Concepts and
Attributes; CA) to illustrate the interaction of both
areas in the global architecture. Each KB and
respective rules were created using Protégé and its
plug-ins.
2.2 Modelling and Design of the
Weightlifting Domain Ontology
To obtain a deep understanding of aspects and concrete
entities comprising the weightlifting TDC cycle, repe-
titive collaboration meetings were organized between
athletes, coaches, biomechanist and nutritionist along
with electronics and software engineers. The following
design artefacts express ontologies in the weightlifting
TDC-cycle knowledge -based system i.e., TDC-
Ontology = (CA, CV, FB, R, A):
2.2.1 Concepts and Attributes (CA)
Different concepts in the TDC-Ontology have been
divided into four main knowledge sets: training,
biomechanics, nutrition, and problem solving,
complemented with an athlete profile concept as
nearly all observation and measurement are around
athlete's activities. The first three sets correspond to
domain ontology which identifies general concepts
and their relations in the field of weightlifting, while
the fourth one is part of the task ontology.
Training-or coaching-related ontology subset
refers to classes modelling exercises performed by
athlete, with each exercise consisting of several
phases. Basically, these concepts are used to promote
a qualitative weightlifting analysis and are mainly
represented by abstract values regarding observable
lifting performance by a coach.
Biomechanics-related ontology subset is used to
leverage a quantitative weightlifting analysis and are
represented by the ExerciseProperty concept. The
main purpose is complementing qualitative lifting
performance values with biomechanics ground values
measured during a lifting exercise phase, using
biomechanics equipment.
Nutrition-related ontology subset is also used to
leverage a quantitative weightlifting analysis and it is
modelled by the following subclasses. The Dietary
Protocol related to each workout period, the
respective NutrientPortions, and the Consumable
having nutrients. Nutritional ground values are
measured for a lifting exercise phase, using a
combination of energy expenditure measurement
equipment, prediction equations, and methods of
analysis. The DietaryProtocol concept prescribes the
receipt of nutrient portions for a specified workout
phase, the NutrientPortions concept identifies a
specific nutrient and its amount in terms of macro-
and micro-nutrients and the Consumable concept
represents the food and drink that are sources of
nutrients. In this prototype, Consumable concept are
adopted from our previous work (Tumnark et al.,
2013). However, in the future, we may consider
adopting the food concept from other available
literature in order to cover all available menus items.
2.2.2 The Controlled Vocabulary (CV)
Horizontal to concepts defined in CA, there is a list of
authorized keywords, used across both domain and
task ontology. The list contains nine subclasses and
under each of them, authorized keywords are used to
provide reference and indexing for communication
with other concepts and instances. Subclasses are the
WorkoutPhase concept defining periods for which a
dietary protocol is prescribed, which is instantiated as
authorized keywords Preworkout, Duringworkout,
and Postworkout. The DayPart concept represents
day time prescribed for weightlifting training and
dietary intake which is instantiated as authorized
keywords Morning, Afternoon, and Evening. The
Acquisition Method concept establishes methods used
to collect quantitative ground values, e.g., heart rate
monitor, motion analysis, electromyography (EMG),
or force measurement; Muscle concept defines
muscles where activity should be measured, e.g.,
VastusLateralis, Biceps Femoris, PectineusGracilis.
The AnalysisMethod concept establishes analysis
methods used for the assessment of energy
expenditure and biomechanics features from several
kinds of collected data, such as kinetics, kinematics,
and physiological. The Calibration Method concept
KEOD 2018 - 10th International Conference on Knowledge Engineering and Ontology Development
210