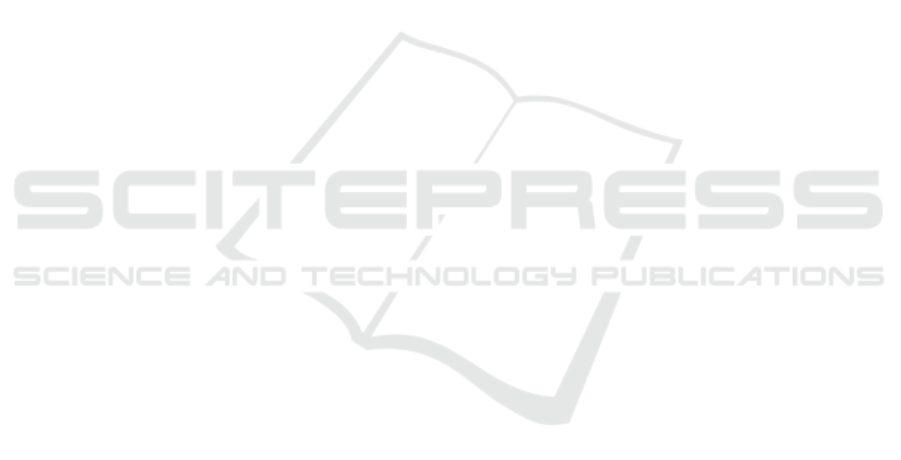
is ”the ability to acquire and apply knowledge and
skills”. This is too broad. Because of this imprecision
in identifying human intelligence, we face the same
dilemma when it comes to machine intelligence, i.e.,
AI. Of course, all are aware that Alan Turing was one
of the first people who asked if machines could think.
Yet, it has been recognized that AI’s goals have often
been debatable, here the points of views are wide and
varied. Experts recognize this inaccuracy and they
do rally for a more formal and accurate definition
(Russell, 2016). This ambiguousness, we believe, is a
source of confusion when AI researchers see the term
used today (Earley, 2016) (Datta, 2017).
If a computer program performs optimization,
is this intelligence? Is prediction the same as in-
telligence? When a computer categorizes correctly
an object, is that intelligence? If something is
automated, is that a demonstration of its capacity
to think? This lack of canonical definition is a
constant problem in AI and it is being brought again
by computer scientists observing the new AI spring
(Datta, 2017).
Carl Sagan said,”You have to know the past to un-
derstand the present” and so let us apply this rule by
studying the history of the AI term so that we may see
why AI is suddenly getting very much publicity these
days.
John McCarthy, the inventor of the LISP program-
ming language, in 1956 introduced the AI term at a
Darthmouth College conference attended by AI per-
sonalities such as Marvin Minsky, Claude Shannon
and Nathaniel Rochester and another seven others
of academic and industrial backgrounds (Russel and
Norwig, 2010), (Buchanan, 2006). The researchers
organized to study if learning or intelligence, ”can be
precisely so described that a machine can be made
to simulate it” (Russel and Norwig, 2010). At that
conference, the thunder came from the work demon-
strated by Allen Newell and Herbert Simon with J.
Clifford Shaw of Carnegie Mellon University on their
Logic Theorist program (Flasinski, 2016) (Russel and
Norwig, 2010). This program was a reasoner and was
able to prove most of the theorems in Chapter 2 of
Principia Mathematica of Bertrand Russell and Al-
fred North Whitehead. Being in the field of foundati-
ons of mathematics, many hoped that all present mat-
hematical theories can be so derived. Ironically they
tried to publish their work at the Journal of Symbolic
Logic but the editors rejected it, not being astounded
that it was a computer that derived and proved the the-
orems.
Though it was in 1956 when the term was used,
the judgment of the community is that as far back
as 1943, the work done by Warren McCulloch and
Walter Pitts in the area of computational neuroscience
is AI (Russel and Norwig, 2010). Their work entitled
A Logical Calculus of Ideas Immanent in Nervous
Activity (McCulloch and Pitts, 1943) (Russel and
Norwig, 2010) (Flasinski, 2016) proposed a model
for artificial neurons as a switch with an ”on” and
”off” states. These states are seen as equivalent to
a proposition for neuron stimulation. McCulloch
and Pitts showed that any computable function can
be computed by some network of neurons. The
interesting part is that they suggested these artificial
neurons could learn. In 1950, Marvin Minsky and
Dean Edmonds inspired by the former’s research on
computational neural networks (NN), built the first
hardware based NN computer. Minsky later would
prove theorems on the limitations of NN (Russel and
Norwig, 2010).
From the above developments we can see that over
optimistic pronouncements emerged right at the in-
ception of AI. Such type of conduct bears upon our
analysis below.
3 AI PARADIGMS AND DEGREES
3.1 Symbolic vs Connectionist
Going back to Section 2, we may observe the follo-
wing. The group gathered by McCarthy proceeded to
work on the use of logic in AI and is consequently
called by some as the Symbolic approach to AI.
Authors have called this view Good Old Fashion
AI (GOFAI). Most of these people apart from Min-
sky, worked on this field and for a while gathered
momentum primarily because it was programming
language based and due to the influence of Newell
and Simon’s results. Those working on NN were
called Connectionists since by the nature of networks,
must be connected. These groups continue to debate
each other on the proper method for addressing the
challenges facing AI (Smolensky, 1987).
This distinction in approaches should come into
play when the AI term is used but hardly is there an
awareness of this in the media and the public.
3.2 Strong or Weak AI
In 1976, Newell and Simon taught that the human
brain is a computer and vice versa. Hence, anything
the human mind can do, the computer should be able
Making AI Great Again: Keeping the AI Spring
145