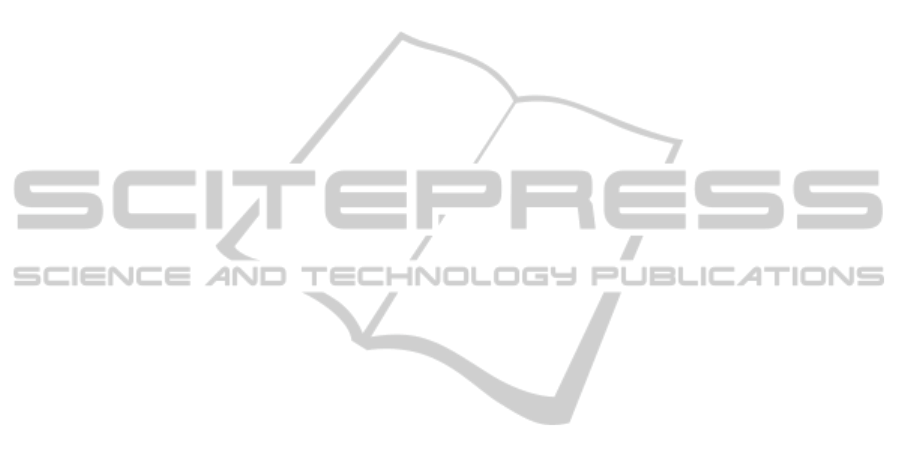
tailers that sell products with prices above the $5 − 6
mark, that carry products with relatively long life-
cycles, that operate with relatively low service levels,
and that plan his sales well or even “buy into mark-
down” should expect higher returns from inventory
visibility.
Our findings suggest further extensions, especially
regarding the retailer’s planning methodology and the
chain structure. One wonders how value of visibility
would change if the retailer adopted a dynamic ap-
proach and updated his forecast and inventory targets
based on observed sales, or if the stores in the chain
were not identical. One would also wonder the in-
teraction of inventory visibility with price promotion
decisions for a retailer. These settings could further
help characterize the value of inventory visibility in a
perishable/fashion retail environment.
REFERENCES
Ashton, K. (2011). Whither the Five-Cent Tag?
www.rfidjournal.com (retrieved: August 1, 2014).
C¸ akıcı, O. E., Groenevelt, H., and Seidmann, A. (2011).
Using RFID for the Management of Pharmaceutical
Inventory - System Optimization and Shrinkage Con-
trol. Decision Support Systems, 51.
Collins, J. (2006). RFID Goes Underground in London.
www.rfidjournal.com (retrieved: August 1, 2014).
de Kok, A. G., Donselaar, K. H. V., and Woensel, T. V.
(2008). A Break-even Analysis of RFID Technology
for Inventory Sensitive to Shrinkage. International
Journal of Production Economics, 112.
DeHoratius, N., Merserau, A. J., and Schrage, L. (2008).
Retail Inventory Management When Records are In-
accurate. Manufacturing & Service Operations Man-
agement, 10(2):257–277.
DeHoratius, N. and Raman, A. (2008). Inventory Record
Inaccuracy: An Empirical Analysis. Management Sci-
ence, 54.
Fleisch, E. and Tellkamp, C. (2005). Inventory Inaccuracy
and Supply Chain Performance: A Simulation Study
of a Retail Supply Chain. International Journal of
Production Economics, 95.
Gaukler, G. M.,
¨
Ozer, O., and Hausman, W. H. (2008). Or-
der Progress Information: Improved Dynamic Emer-
gency Ordering Policies. Production and Operations
Management, 17(6):599–613.
Kang, Y. and Gershwin, S. B. (2005). Information Inaccu-
racy in Inventory Systems: Stock Loss and Stockout.
IIE Transactions, 37.
Karaer, O. and Lee, H. L. (2007). Managing the Reverse
Channel with RFID-Enabled Negative Demand In-
formation. Production and Operations Management,
16(5):625–645.
K
¨
ok, A. G. and Shang, K. H. (2007). Inspection and
Replenishment Policies for Systems with Inventory
Record Inaccuracy. Manufacturing & Service Oper-
ations Management, 9(2):185–205.
Lee, H. L. and
¨
Ozer, O. (2007). Unlocking the Value
of RFID. Production and Operations Management,
16(1):40–64.
Merserau, A. J. (2013). Information-Sensitive Replenish-
ment when Inventory Records are Inaccurate. Pro-
duction and Operations Management, 22(4).
Pisello, T. (2006). The ROI of RFID in the Supply Chain.
www.rfidjournal.com (retrieved: August 1, 2014).
Prince, P. (2013). Hospitals in Japan, China Seek to Save
Lives via Pocketsize Reader. www.rfidjournal.com
(retrieved: August 1, 2014).
Reda, S. (2010). Ready (Finally) For Item Level Deploy-
ment. Stores.org (retrieved: August 1, 2014).
Rekik, Y., Jemai, Z., Sahin, E., and Dallery, Y. (2007). Im-
proving the Performance of Retail Stores Subject to
Execution Errors: Coordination versus RFID Tech-
nology. OR Spektrum, 29.
Rekik, Y., Sahin, E., and Dallery, Y. (2008). Analysis of
the Impact of RFID Technology on Reducing Product
Misplacement Errors at Retail Stores. International
Journal of Production Economics, 112.
Rekik, Y., Sahin, E., and Dallery, Y. (2009). Inventory In-
accuracy in Retail Stores due to Theft: An Analysis of
the Benefits of RFID. International Journal of Pro-
duction Economics, 118.
Roberti, M. (2010). Wal-Mart Relaunches EPC RFID
Effort, Starting With Men’s Jeans and Basics.
www.rfidjournal.com (retrieved: August 1, 2014).
Sahin, E., Buzacott, J., and Dallery, Y. (2008). Analysis
of a Newsvendor Which Has Errors in Inventory Data
Records. European Journal of Operational Research,
188.
Sarac¸, A., Absi, N., and Dauzere-Peres, S. (2010). A Liter-
ature Review on the Impact of RFID Technologies on
Supply Chain Management. International Journal of
Production Economics, 128.
Staff (2003). The best thing since the bar-code.
www.economist.com (retrieved: August 1, 2014).
Staff (2011). Macy’s pushing to get RFID technology into
stores. www.businessweek.com (retrieved: August 1,
2014).
Swedberg, C. (2013). Russian Tag Company Reaches for
a Worldwide Audience. www.rfidjournal.com (re-
trieved: August 1, 2014).
ICORES2015-InternationalConferenceonOperationsResearchandEnterpriseSystems
184