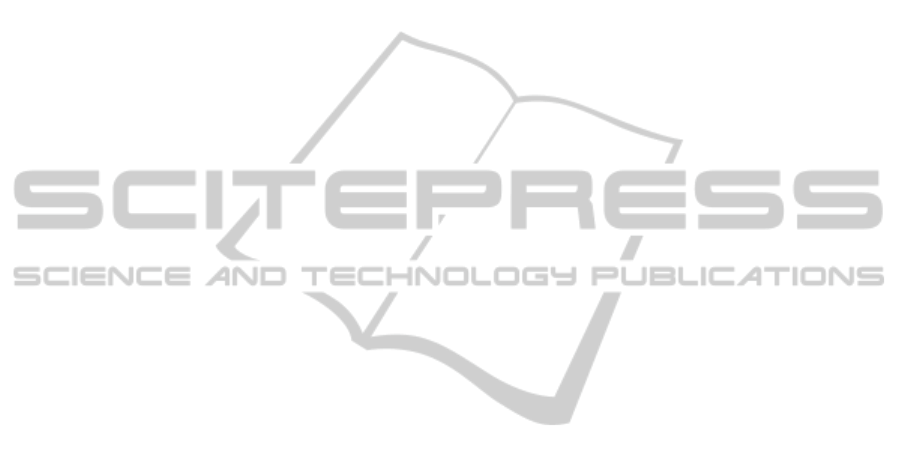
tional near infrared spectroscopy (NIRS) signal im-
provement based on negative correlation between
oxygenated and deoxygenated hemoglobin dynamics.
NeuroImage, 49(4):3039–3046.
Cui, X., Bray, S., and Reiss, A. L. (2010b). Speeded near in-
frared spectroscopy (NIRS) response detection. PLoS
ONE, 5(11).
Heger, D., Mutter, R., Herff, C., Putze, F., and Schultz, T.
(2013). Continuous recognition of affective states by
functional near infrared spectroscopy signals. In Proc.
ACII.
Heger, D., Putze, F., and Schultz, T. (2011). Online recog-
nition of facial actions for natural EEG-based BCI ap-
plications. In Proc. ACII, pages 436–446.
Hoshi, Y. (2011). Functional near-infrared spectroscopy:
current status and future prospects. J. of Biomed. Op.,
12(6).
Hoshi, Y. and Tamura, M. (1997). Near-infrared optical de-
tection of sequential brain activation in the prefrontal
cortex during mental tasks. NeuroImage, 5(4):292–7.
Kawaguchi, Y., Wada, K., Okamoto, M., Tsujii, T., Shibata,
T., and Sakatani, K. (2012). Investigation of brain
activity after interaction with seal robot measured by
fNIRS. In IEEE International Symposium on Robot
and Human Interactive Communication.
Lisetti, C. L. (2011). Believable agents, engagement, and
health interventions. In Proceedings of the Human
Computer Interaction International Conference.
Ochsner, K. N., Silvers, J. A., and Buhle, J. T. (2012). Func-
tional imaging studies of emotion regulation: a syn-
thetic review and evolving model of cognitive control
of emotion. An. of NY Acad. Sci., 1251:1–24.
Rosenthal von der Putten, A. M., Kramer, N. C., Hoffmann,
L., Sobieraj, S., and Eimler, S. C. (2013a). An exper-
imental study on emotional reactions towards a robot.
International Journal of Social Robotics, 5:17–34.
Rosenthal von der Putten, A. M., Schulte, F. P., Eimler,
S. C., Hoffmann, L., Sobieraj, S., Maderwald, S.,
Kramer, N. C., and Brand, M. (2013b). Neural corre-
lates of empathy towards robots. In Proc. HRI, pages
215–216.
Shibata, T. (2012). Therapeutic seal robot as biofeedback
medical device: Qualitative and quantitative evalua-
tions of robot therapy in dementia care. Proceedings
of the IEEE, 100(8):2527 –2538.
Solovey, E. T., Girouard, A., Chauncey, K., Hirshfield,
L. M., Sassaroli, A., Zheng, F., Fantini, S., and Ja-
cob, R. J. K. (2009). Using fNIRS brain sensing in
realistic HCI settings: experiments and guidelines. In
Proc. UIST.
Solovey, E. T., Lalooses, F., Chauncey, K., Weaver, D.,
Parasi, M., Scheutz, M., Sassaroli, A., Fantini, S.,
Schermerhorn, P., Girouard, A., and Jacob, R. J. K.
(2011). Sensing cognitive multitasking for a brain-
based adaptive user interface. In Proc. CHI.
Solovey, E. T., Schermerhorn, P., Scheutz, M., Sassaroli, A.,
Fantini, S., , and Jacob, R. J. K. (2012). Brainput: en-
hancing interactive systems with streaming fnirs brain
input. In Proc. CHI.
Strait, M., Briggs, G., and Scheutz, M. (2013a). Neural
correlates of agency ascription and its role in emo-
tional and non- emotional decision-making. In Affec-
tive Computing and Intelligent Interaction.
Strait, M., Canning, C., and Scheutz, M. (2013b). Limita-
tions of NIRS-based BCI for realistic applications in
human-computer interaction. In 5th International BCI
Meeting, pages 6–7.
Strait, M., Canning, C., and Scheutz, M. (2014). Inves-
tigating the effects of robot communication strategies
in advice-giving situations based on robot appearance,
interaction modality, and distance. In Conference on
Human-Robot Interaction (under review).
Strait, M. and Scheutz, M. (2014). Measuring users‘ re-
sponses to humans, robots, and human-like robots
with functional near infrared spectroscopy. In Confer-
ence on Human Factors in Computing (under review).
Szafir, D. and Mutlu, B. (2012). Pay attention!: designing
adaptive agents that monitor and improve user engage-
ment. In Proceedings of CHI, pages 11–20.
Szafir, D. and Mutlu, B. (2013). Artful: Adaptive review
technology for flipped learning. In Proc. of CHI.
Urry, H. L., van Reekum, C. M., Johnstone, T., Kalin, N. H.,
Thurow, M. E., Schaefer, H. S., Jackson, C. A., Frye,
C. J., Geischar, L. L., Alexander, A. L., and David-
son, R. J. (2006). Amygdala and ventromedial pre-
frontal cortex are inversely coupled during regulation
of negative affect and predict the diurnal pattern of
cortisol secretion among older adults. J. Neurosci.,
26(16):4415–4425.
Zander, T. O. (2009). Detecting affective covert user states
with passive brain-computer interfaces. In Proceeding
of Affective Computing and Intelligent Interaction.
Zander, T. O. and Kothe, C. (2011). Towards passive brain-
computer interfaces: applying brain-computer inter-
face technology to human-machine systems in gen-
eral. Journal of Neural Engineering, 8(2).
PhyCS2014-InternationalConferenceonPhysiologicalComputingSystems
392