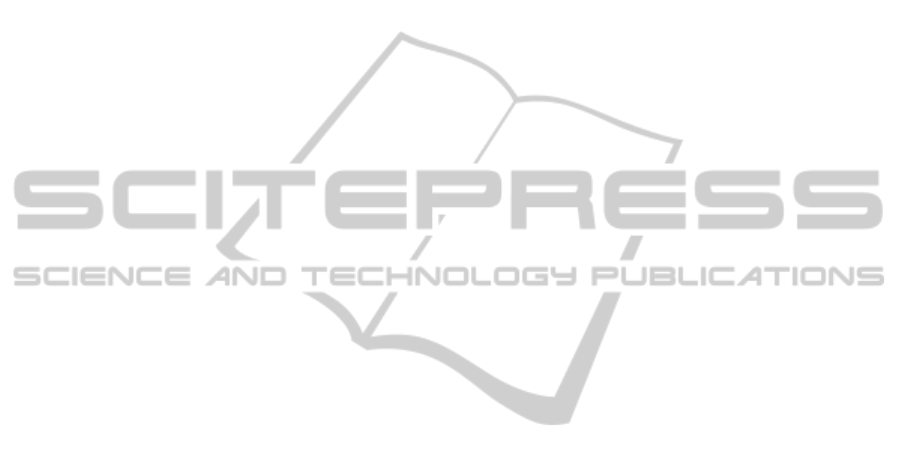
synthesis of such algorithms in fixed-point arithmetic,
like matrix inversion, for these particular hardware
and the adaptation of the techniques presented here
to such particular problems.
ACKNOWLEDGEMENTS
The work was supported by the ANR project DE-
FIS (Ing
´
enierie Num
´
erique et S
´
ecurit
´
e 2011, ANR-
11-INSE-0008).
REFERENCES
ALshebeili, S. A. (2001). Computation of higher-order
cross moments based on matrix multiplication. Jour-
nal of the Franklin Institute, 338(7):811–816.
Campbell, S. J. and Khatri, S. P. (2006). Resource and de-
lay efficient matrix multiplication using newer FPGA
devices. In Proceedings of the 16th ACM Great Lakes
Symposium on VLSI, GLSVLSI ’06, pages 308–311,
New York, NY, USA. ACM.
Cormen, T. H., Leiserson, C. E., Rivest, R. L., and Stein,
C. (2009). Introduction to Algorithms (3. ed.). MIT
Press.
Golub, G. and Mitchell, I. (1998). Matrix factorizations in
Fixed Point on the C6x VLIW architecture. Techni-
cal report, Stanford University, Standford, California,
USA.
Irturk, A., Benson, B., Mirzaei, S., and Kastner, R. (2010).
GUSTO: An automatic generation and optimization
tool for matrix inversion architectures. ACM Trans.
Embed. Comput. Syst., 9(4):32:1–32:21.
Kim, S., il Kum, K., and Sung, W. (1998). Fixed-point
optimization utility for C and C++ based digital sig-
nal processing programs. In IEEE Trans. Circuits and
Systems II, pages 1455–146.
Lee, D.-U., Gaffar, A., Cheung, R. C. C., Mencer, O.,
Luk, W., and Constantinides, G. (2006). Accuracy-
guaranteed bit-width optimization. Computer-Aided
Design of Integrated Circuits and Systems, IEEE
Transactions on, 25(10):1990–2000.
Lee, D.-U. and Villasenor, J. D. (2009). Optimized Custom
Precision Function Evaluation for Embedded Proces-
sors. IEEE Transactions on Computers, 58(1):46–59.
Mehlhose, M. and Schifferm
¨
uller, S. (2009). Efficient
Fixed-Point Implementation of Linear Equalization
for Cooperative MIMO Systems. 17th European Sig-
nal Processing Conference (EUSIPCO 2009).
Melquiond, G. (2006). De l’arithm
´
etique d’intervalles
`
a la
certification de programmes. PhD thesis,
´
ENS Lyon.
Moore, R. E., Kearfott, R. B., and Cloud, M. J. (2009). In-
troduction to Interval Analysis. SIAM.
Mouilleron, C. (2011). Efficient computation with struc-
tured matrices and arithmetic expressions. PhD the-
sis, Univ. de Lyon - ENS de Lyon.
Mouilleron, C., Najahi, A., and Revy, G. (2013). Auto-
mated Synthesis of Target-Dependent Programs for
Polynomial Evaluation in Fixed-Point Arithmetic.
Technical Report 13006.
Mouilleron, C. and Revy, G. (2011). Automatic Generation
of Fast and Certified Code for Polynomial Evaluation.
In Proc. of the 20th IEEE Symposium on Computer
Arithmetic (ARITH’20), Tuebingen, Germany.
Nikolic, Z., Nguyen, H. T., and Frantz, G. (2007). Design
and Implementation of Numerical Linear Algebra Al-
gorithms on Fixed-Point DSPs. EURASIP J. Adv. Sig.
Proc., 2007.
Ogita, T., Rump, S. M., and Oishi, S. (2005). Accurate Sum
and Dot Product. SIAM J. Sci. Comput., 26(6):1955–
1988.
Qasim, S. M., Abbasi, S., Alshebeili, S., Almashary, B., and
Khan, A. A. (2008). FPGA Based Parallel Architec-
ture for the Computation of Third-Order Cross Mo-
ments. International Journal of Computer, Informa-
tion and Systems Science, and Engineering, 2(3):216–
220.
Rump, S. M. (2009). Ultimately Fast Accurate Summation.
SIAM J. Sci. Comput., 31(5):3466–3502.
Shamos, M. I. and Hoey, D. (1975). Closest-point problems.
In FOCS, pages 151–162.
Sotiropoulos, I. and Papaefstathiou, I. (2009). A fast par-
allel matrix multiplication reconfigurable unit utilized
in face recognitions systems. In Field Programmable
Logic and Applications, 2009. FPL 2009. Interna-
tional Conference on, pages 276–281.
Syed M. Qasim, Ahmed A. Telba, A. Y. A. (2010). FPGA
Design and Implementation of Matrix Multiplier Ar-
chitectures for Image and Signal Processing Applica-
tions. International Journal of Computer Science and
Network Security, 10(2):168–176.
Yates, R. (2009). Fixed-Point Arithmetic: An Introduction.
Digital Signal Labs.
PECCS2014-InternationalConferenceonPervasiveandEmbeddedComputingandCommunicationSystems
214