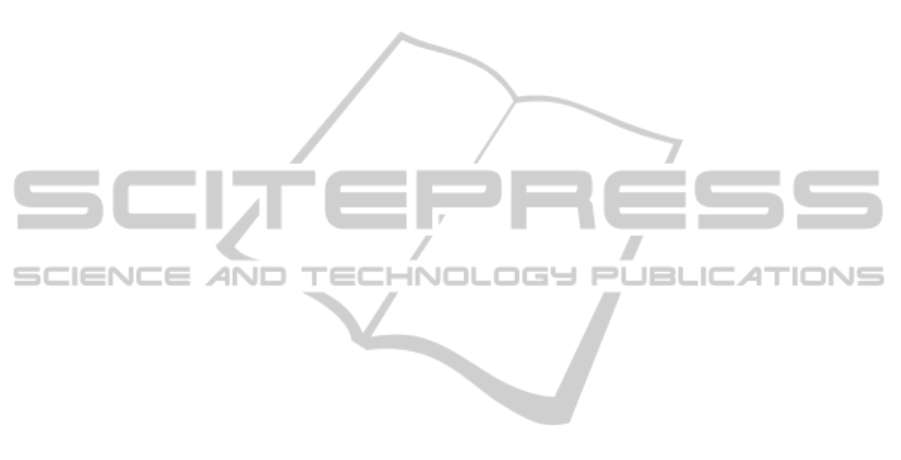
2011) using FES and a spinal cord injured person,
we have also found that the mean frequency
decreases during the periods of extension
maintenance. Therefore, the results presented in this
paper are consistent with studies already published
and show that this technique can be useful in
following the evolution of FES-elicited muscle
contraction. Convex hull area, despise its limitations,
can be a promising candidate as a parameter
indicating muscle fatigue in closed-loop FES control
systems.
5 CONCLUSIONS
In this study, we proposed the use of a computational
geometry technique known as the convex hull of a
finite set of planar points in the analysis of triaxial
mechanomyography signals. During an open chain
contraction and maximum knee extension evoked by
functional electrical stimulation, the convex hull
showed that its area reduced in all planes in a space
defined by the three axes of the mechanomyography
sensor. This reduction in the convex hull area was
related to a decrease in force production due to sup-
pressive performance phenomena like muscle fa-
tigue.
ACKNOWLEDGEMENTS
The authors thank CNPq, SETI-PR, CAPES e
FINEP for the financial support.
REFERENCES
Akataki, K., Mita, K. & Itoh, Y. 1999. Relationship Be-
tween Mechanomyogram And Force During Voluntary
Contractions Reinvestigated Using Spectral Decompo-
sition. European Journal Of Applied Physiology, 80,
173-9.
Bajd, T., Kralj, A., Sega, J., Turk, R., Benko, H. & Stro-
jnik, P. 1981. Use Of A Two-Channel Functional Elec-
trical Stimulator To Stand Paraplegic Patients. Physi-
cal Therapy, 61, 526-27.
Beck, T. W., Housh, T. J., Johnson, G. O., Weir, J. P.,
Cramer, J. T., Coburn, J. W. & Malek, M. H. 2005.
Comparison Of Fourier And Wavelet Transform Pro-
cedures For Examining The Mechanomyographic And
Electromyographic Frequency Domain Responses
During Fatiguing Isokinetic Muscle Actions Of The
Biceps Brachii. Journal Of Electromyography And Ki-
nesiology, 15, 190-9.
Chapin, J., Moxon, K., Markowitz, R. & Nicolelis, M.
1999. Real-Time Control Of A Robot Arm Using Sim-
ultaneously Recorded Neurons In The Motor Cortex.
Nature Neuroscience, 2, 664-70.
Fong, S., Hang, Y., Mohammed, S. & Fiaidhi, J. 2011.
Stream-Based Biomedical Classification Algorithms
For Analyzing Biosignals. Journal Of Information
Processing Systems, 7, 717-32.
Gobbo, M., Cè, E., Diemont, B., Esposito, F. & Orizio, C.
2006. Torque And Surface Mechanomyogram Parallel
Reduction During Fatiguing Stimulation In Human
Muscles. European Journal Of Applied Physiology,
97, 9-15.
Graham, R. L. 1972. An Efficient Algorithm For Deter-
mining The Convex Hull Of A Finite Planar Set. In-
formation Processing Letters, 1, 132-3.
Gregory, C. M. & Bickel, C. S. 2005. Recruitment Patterns
In Human Skeletal Muscle During Electrical Stimula-
tion. Physical Therapy, 85, 358-64.
Hubert, M. & Rousseeuw, P. J. 2005. Robpca: A New
Approach To Robust Principal Component Analysis.
Technometrics, 47, 64-79.
Jailani, R. & Tokhi, M. O. 2012. The Effect Of Functional
Electrical Stimulation (Fes) On Paraplegic Muscle Fa-
tigue. Ieee 8th International Colloquium On Signal
Processing And Its Applications (Cspa). Shah Alam,
Selangor, Malaysia: Ieee.
Langlois, P. J., Demosthenous, A., Pachnis, I. & Donald-
son, N. 2010. High-Power Integrated Stimulator Out-
put Stages With Floating Discharge Over A Wide Volt-
age Range For Nerve Stimulation. Ieee Transactions
On Biomedical Circuits And Systems, 4, 39-48.
Nogueira-Neto, G. N., Krueger, E., Scheeren, E. M., No-
hama, P. & Button, V. L. S. 2011. Estimulação Elétrica
Funcional Aplicada Em Cadeia Aberta - Um Estudo
De Caso Com Monitoração Mecanomiográfica. 5th
Latin American Congress Of Biomedical Engineering.
Havana: Ifmbe.
Osborne, J. W. & Costello, A. B. 2004. Sample Size And
Subject To Item Ratio In Principal Components Anal-
ysis. Practical Assessment, Research & Evaluation, 9,
8.
Peng, C.-W., Chen, S.-C., Lai, C.-H., Chen, C.-J., Chen,
C.-C., Mizrahi, J. & Handa, Y. 2011. Review: Clinical
Benefits Of Functional Electrical Stimulation Cycling
Exercise For Subjects With Central Neurological Im-
pairments. Journal Of Medical And Biological Engi-
neering, 31, 1-11.
Petitjean, M., Maton, B. & Fourment, A. 1998. Summation
Of Elementary Phonomyograms During Isometric
Twitches In Humans. European Journal Of Applied
Physiology, 77, 527-35.
Petrofsky, J. S. 2004. Electrical Stimulation: Neurophysio-
logical Basis And Application. Basic And Applied My-
ology, 14, 205-13.
Qi, L., Wakeling, J. M., Green, A., Lambrecht, K. & Fer-
guson-Pell, M. 2011. Spectral Properties Of Electro-
myographic And Mechanomyographic Signals During
Isometric Ramp And Step Contractions In Biceps Bra-
ConvexHullAreainTriaxialMechanomyographyduringFunctionalElectricalStimulation
255