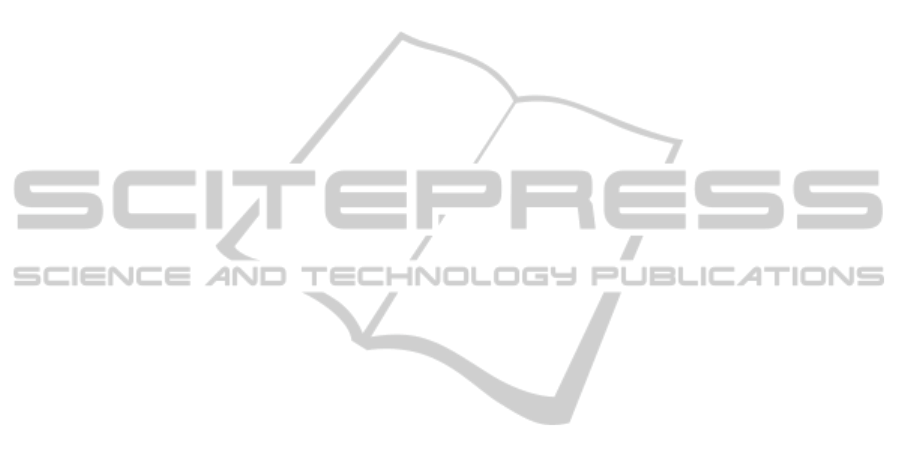
sensor signal was available to the classifier, the
performance usually decreased. The most worthy
contribution to high performance gas sensing came
from the dynamic exposure conditions, causing fast
change of the sensor signal both, when rising (Fig. 3
and Fig. 7) and when decreasing (Fig. 5 and Fig. 9).
In this approach the static exposure conditions were
least informative (Fig. 4 and Fig. 8). The obtained
results promise that the measurement period in the
single sensor portable devise may be shorter than we
originally thought. It may be achieved by
eliminating the middle part of the operation mode,
i.e. stopping the gas flow.
Based on our studies, the single sensor gas
sensing may be effectively performed with low
energy consumption sensors. Overall, the best sensor
of this group for recognizing the gas type was TGS
2620 (Fig. 2). The concentration categories were
best delimited by with TGS 2600 (Fig. 6). The
heaters of both sensors consume 210 mW each. It
proves the technical feasibility of the concept of the
battery powered portable gas sensing device.
5 CONCLUSIONS
The concept of the portable gas sensing device was
presented in this work. It was assumed that the
semiconductor gas sensors shall be applied in our
solution, as this technology guaranties the best
reliability. However, due to high power consumption
required for maintaining the adequate temperature of
the sensing layer, more than one sensor of this kind
is not allowed in the instrument. The portable
character of the device imposes strict power
consumption restrictions. Therefore, a prerequisite
for the feasibility of the concept was the successful
identification of gases and/or the concentration
categories determination using the single sensor
approach.
We showed that the prerequisite may be
satisfied. The crucial factor for achieving this goal
was to apply the active sampling approach in the gas
sensing device. It allows for altering sensor exposure
conditions in time. As a result of variable exposure
conditions the sensor signal may be considered as a
response of the “virtual sensor array”. We showed
that the information content of this data was
sufficient to recognize different volatile organic
compounds and to determine the concentration
categories for the gas samples. The achieved
assessment error was less than 5 %. The portable
sensor device with such performance characteristics
well fits the existing market niche.
ACKNOWLEDGEMENTS
This contribution was supported by the project: "The
variability of physical and chemical parameters in
time as the source of comprehensive information
about indoor air quality". The project is financially
supported by the National Science Center, Poland,
under the contract No. UMO-2012/07/B/ST8/03031.
REFERENCES
Alippi C., Pelosi G., Roveri M., 2006. Computational
intelligence techniques to detect toxic gas presence,
IEEE international Conference on Computational
Intelligence for Measurement Systems and
Applications (CIMSA), La Coruna, Spain.
Conner L., Chin S., Furton K.G., 2006. Evaluation of field
sampling techniques including electronic noses and a
dynamic headspace sampler for use in fire
investigations, Sensors and Actuators B, 116(1–2),
121129.
Gutierrez-Osuna R., Korah S., Perera A., 2001. Multi-
frequency temperature modulation for metal oxide gas
sensors, The 8
th
International Symposium on Olfaction
and the Electronic Nose, Washington, DC.
Kimon I., Bârsan N., Bauer M., Weimar U., 2001.
Micromachined metal oxide gas sensors: opportunities
to improve sensor performance, Sensors and Actuators
B, 73(1), 1–26.
Lee A. P., Reedy B. J., 1999. Temperature modulation in
semiconductor gas sensing, Sensors and Actuators B,
60, 3542.
Lodge J. P, 1989. Methods of air sampling and analysis,
Lewis Publishers Inc., 3742.
Maciejewska M., Szczurek A., Bodzoj Ł., Flisowska-
Wiercik B., 2010. Sensor array and stop-flow mode
applied to discrimination and quantification of gas
mixtures, Sensors and Actuators B, 150, 93–98.
Nakata S., Takemura K., Neya K., 2001. Non-linear
dynamic responses of a semiconductor gas sensor:
Evaluation of kinetic parameters and competition
effect on the sensor response, Sensors and Actuators
B, 76(1–3), 436441.
Perera A., Sundic T., Pardo A., Gutierrez-Osuna R.,
Marco S., 2002. A portable electronic nose based on
embedded PC technology and GNU/Linuxhardware,
software and applications, IEEE Sensors Journal, 2(3),
235246.
Polikar R., Shinar R., Udpa L., Porter M.D., 2001.
Artificial intelligence methods for selection of an
optimized sensor array for identification of volatile
organic compounds, Sensors and Actuators B, 80,
243254.
Postolache O., Pereira M., Girão P., 2005. Smart Sensor
Network for Air Quality Monitoring Applications,
IMTC 2005 – Instrumentation and Measurement
PortableSensingofOrganicVapoursbasedonaSingleSemiconductorSensor
321