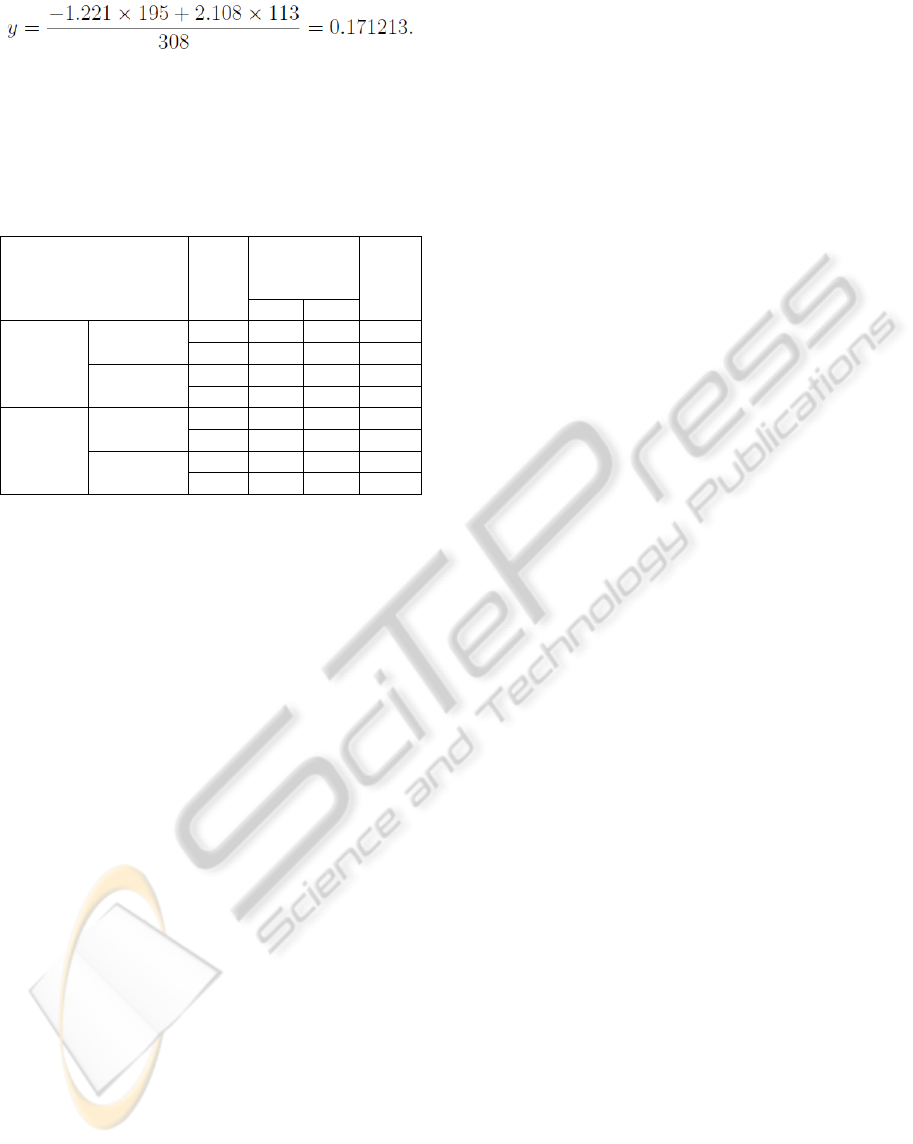
(8)
We judged whether a participant suffered from
mental illnesses by comparing the values of f and y:
the participant was classified as mentally ill if f < y
and as mentally healthy if f > y.
Table 6: Classification results.
V
Predicted
group
membership
Total
0 1
Original Count 0 190 5 195
1 10 103 113
Percentage 0 97.4 2.6 100.0
1 8.8 91.2 100.0
Cross-
validated
Count 0 189 6 195
1 10 103 113
Percentage 0 96.9 3.1 100.0
1 8.8 91.2 100.0
Table 6 presents the classification results for the
participants. We were able to correctly classify
97.4% of the mentally ill patients and 91.2% of the
mentally healthy students in our sample.
4 CONCLUSIONS AND REMARK
To conclude, this study has identified characteristic
physiological patterns of mentally ill patients using
pulse waves measurement. From the results of the
analysis, we obtained a significant difference
between these groups in LLE and ANB.
Notably, this system can display the activity of
sympathetic nerves, parasympathetic nerves, and
LLE at the same time, which enables us to assess the
mental status of patients when measuring their
fingertip pulse waves. Furthermore, the
methodology is simple and the operation is
economical. It can be used for early detection of
mental illnesses.
We hope that this system can contribute to
promoting better medical care. We will strive to
collect and analyze more data of mentally illness
sufferers, and intend to continue relevant studies in
the future.
ACKNOWLEDGEMENTS
We are deeply thankful to Dr. Oshima, president and
psychological counsellor at the Insight Counselling
Corporation (Tokyo, Japan) who helped provide the
pulse waves measurements of the mentally ill
patients and diagnoses of their mental illnesses. We
thank Dr. Shomura who provided the English names
of the mental illnesses.
REFERENCES
Abarbanel, H. D. I., Brown, R., Sidorowich, J. J.,
Tsimring, L. S. (1993). The Analysis of Observed
Chaotic Data in Physical Systems. Rev. Mod. Phys.,
65, 1331-1992.
Imanishi, A. and Oyama-Higa, M. (2006). The Relation
Between Observers’ Psychophysiological Conditions
and Human Errors During Monitoring Task. 2006
IEEE Conference on Systems, Man, and Cybernetics,
2035–2039.
Ministry and Health, Labour and Welfare (Japan). (n.d.).
The patient investigation. Retrieved March 26, 2012,
from http://www.mhlw.go.jp/toukei/list/10-20.html
Oyama-Higa, M. and Miao, T. (2006). Discovery and
Application of New Index for Cognitive Psychology.
2006 IEEE Conference on Systems, Man, and
Cybernetics, 2040–2044.
Oyama-Higa, M., Miao, T. and Mizuno-Matsumoto, Y.
(2006). Analysis of Dementia in Aged Subjects
Through Chaos Analysis of Fingertip Pulse Waves.
2006 IEEE Conference on Systems, Man, and
Cybernetics, 2863–2867.
Sano, M. and Sawada, Y. (1985). Measurement of the
Lyapunov Spectrum From a Chaotic Time Series.
Phys. Rev. Lett., 55, 1082-1085.
Sumida, T., Arimitu, Y., Tahara, T. and Iwanaga, H.
(2000). Mental Conditions Reflected by the Chaos of
Pulsation in Capillary Vessels. Int. J. Bifurcation and
Chaos, 10, 2245–2255.
Tsuda, I., Tahara, T. and Iwanaga, I. (1992). Chaotic
Pulsation in Capillary Vessels and Its Dependence on
Mental and Physical Conditions. Int. J. Bifurcation
and Chaos, 2, 313–324.
Identifying Characteristic Physiological Patterns of Mentally Ill Patients using Nonlinear Analysis of Plethysmograms
73