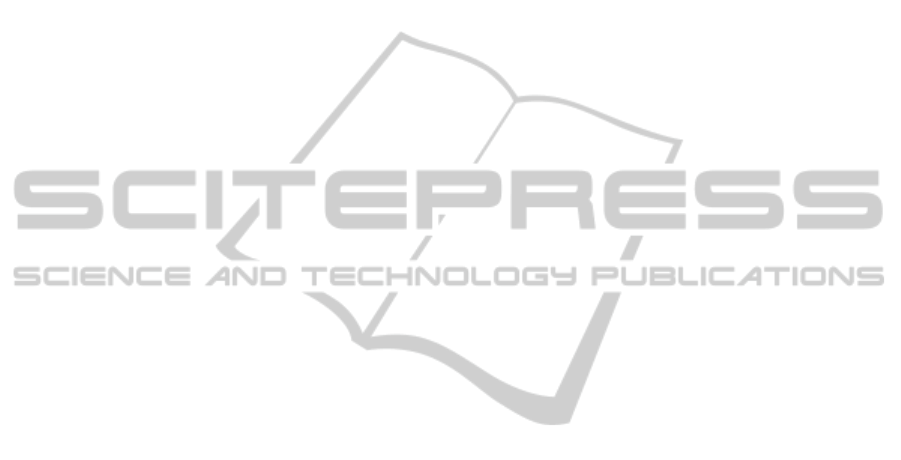
The tree formation measurements were
performed on trees of the Ficus species, where the
directional spectrum was recorded at four specific
positions inside the forest medium. The experiment
geometry is depicted in Figure 1 b). The directional
spectrum was recorded by rotating the receiver
around its own axis, in the azimuth plane, over a
range of 360º with an angular resolution of 2º. The
transmitter was placed conveniently to guarantee the
illumination of 90º of the air-to-vegetation interface.
The 10 and 20 dBi antennas used in the single trees
measurements, were also used in these
measurements, on the transmitter and receiver side,
respectively. The received time-series were recorded
over a period of 10s, per angle, with a sampling rate
of 1 kHz. Once again the wind induced effects were
simulated with a household fan, placed in four
distinct locations around the tree, as seen in Figure 1
b). The fan produces a wind front at the air-to-
vegetation interface with relative narrow width
illumination (around 2 trees), in comparison to wide
uniform wind illumination observed in outdoor
forest geometries. However, given the small
dimensions of the indoor measurement geometries,
the employed method to generate artificial wind is
found to be suitable.
2.2 Statistical Analysis
A statistical analysis was performed on the single
tree and tree formation measurements. The ensuing
results are presented. The single tree results focus on
the effects of different wind incidences on the
scattered radio signals. The tree formation results
aim to investigate the effect of vegetation depth on
the received signal, as wind induced vegetation
movement causes channel dynamics across the
foliage medium, from different incidences.
The analysis of the single tree results is done
through the appreciation of the re-radiation pattern
through a skewed box plot based on a Lognormal
distribution, and second order statistics Average
Fade Duration (AFD) and Level Crossing Rate
(LCR). Concerning the box plot depictions, on each
blue box the central mark is the median, the edges of
the box are the 25
th
and 75
th
percentiles, the whiskers
(dotted line) extend to the most extreme data points,
and the outliers (red dots) are plotted individually.
The box plot enables the analysis of the following
information about the data: position, spread,
skewness and tails. The measured radio signal
scattered from the vegetation volume is shown to be
influenced by the direction of the artificially
generated wind. As wind is blown on to a single tree
from a specific direction, two areas of vegetation
motion need to be considered. One area of the tree,
where the wind incises directly on the foliage (active
area), and another area opposite to the first (quiet
area). The foliage dynamics in the quiet area are
observed to be reduced in comparison to the active
area, as a result of wind speed decay through the
vegetation media. A comparison between measured
re-radiation patterns obtained with opposite wind
directions is depicted in Figure 2. The active area is
considered to be from Φ=-120º to Φ=-50º and
Φ=50º to Φ=120º, for wind directions B and F,
respectively. The quiet area may be defined as the
opposite, in reference to the wind direction. For both
wind incidences in analysis, the received signal in
the active area presents an increased standard
deviation in comparison to the quiet area. This is
indicated by the lack of outliers in the active area
below the mean level. The increased number of
outliers in the quiet area below the mean level shows
that the received signal seldom falls into signal
levels as low as the ones observed in the active area.
In addition, analysis of the AFD and LCR statistics,
depicted in Figure 3 and Figure 4, show that the
deep fades in the active area as well as the fast-
fading signal variation are greater compared to the
quiet area results. Furthermore, analysis of the
results in Figure 5, show that a distinct
differentiation can be made on whether the wind
incidence is on the transmitter or receiver side. The
depicted results relate to AFD and LCR statistics,
obtained at Φ=-90º and Φ=90º, for wind incidences
of B and C. These show lower signal fades and
signal crossing rates observed in results from wind
direction B, against wind direction C. When the
direction of wind illuminates the tree canopy in the
same direction of the propagating radio wave, the
received signal presents smaller signal fades and
lower signal variation compared to wind
illumination from the receiver (opposite) side.
The investigation of the tree formation results is
done through the analysis of the re-radiation pattern
and its corresponding boxplot. The measured
directional spectrum inside a forest medium is
shown to be influenced not only by the direction of
wind illumination, but also the amount of vegetation
between the source of wind and position of the
receiver. Analysis of the results depicted in Figure 6,
show that the origin of measured scatter dynamics
varies according to the wind source of illumination.
For instance, Figure 6 a) shows the re-radiation
pattern relative to wind direction B. In this case the
measured dynamics observed from Φ=-100º to
Φ=50º (except in the main lobe region where the
InvestigationofaRadioPropagationModelforVegetationScatterDynamicChannelsatBFWAFrequencies
331