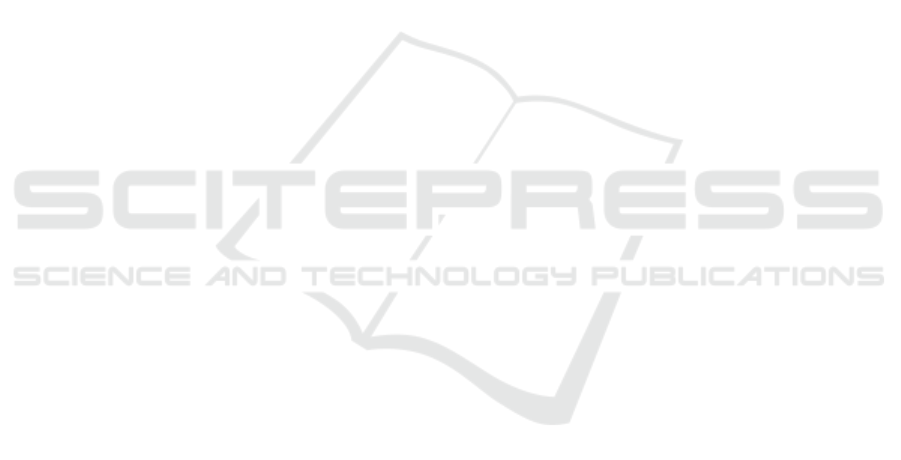
demonstrate the applicability of the novel approach in
assisting the purchasing manager at company A to
produce a green and resilient purchasing strategy
through supplier evaluation.
The developed methodology can be applied to
other companies as a tool to measure the healthiness
of their supply chain in terms of resilience and green
performance. Furthermore, it mediates the
uncertainty in experts’ opinions through the use of
fuzzy evaluation.
Ongoing work includes the incorporation of social
criteria to those already studied here. Finally, the
authors are developing a multi-objective optimization
model to help decision makers in solving order
allocation problem with respect to TGR performance
of suppliers.
REFERENCES
Akman, G., 2015. Evaluating suppliers to include green
supplier development programs via fuzzy c-means and
VIKOR methods. Comput. Ind. Eng. 86, 69–82.
BBC News, 18 Mar 2011. Japan disaster: Supply shortages
in three months. BBC News. (accessed 11.02.17).
Bing, X., Bloemhof-Ruwaard, J., Chaabane, A., van der
Vorst, J., 2015. Global reverse supply chain redesign
for household plastic waste under the emission trading
scheme. J. Clean. Prod. 103, 28-39. Aissaoui, N.,
Haouari, M., Hassini, E., 2007. Supplier selection and
order lot sizing modeling: a review. Com. Oper. Res.
34, 3516–3540.
Burnson, P., 30 October 2012. Nation’s Supply Chains
Disrupted by Hurricane Sandy. Logistics Management.
(accessed 14.06.17).
Büyüközkan and Çifçi, 2010 G. Büyüközkan, G. Çifçi.
Evaluation of the green supply chain management
practices: a fuzzy ANP approach. Production Planning
& Control, iFirst (2010), pp. 1-14
Chen, C.T., Lin, C.T., Huang, S.F., 2006. A fuzzy approach
for supplier evaluation and selection in supply chain
management. Int. J. Prod. Econ. 102, 289–301.
Dickson, G., 1966. An analysis of vendor selection systems
and decisions, J. Purch. 2(1), 5–17.
Govindan, K., Rajendran, S., Sarkis, J., Murugesan, P.,
2015. Multi criteria decision making approaches for
green supplier evaluation and selection: a literature
review. J. Clean. Prod. 98, 66–83.
Govindan, K., Sivakumar, R., 2016. Green supplier
selection and order allocation in a low-carbon paper
industry: integrated multi-criteria heterogeneous
decision making and multi-objective linear
programming approaches. Ann. Oper. Res. 238, 243–
276.
Ha, S.H., Krishnan, R., 2008. A hybrid approach to supplier
selection for the maintenance of a competitive supply
chain. Expe. Sys. with Appl. 34, 1303–1311.
Haldar, A., Ray, A., Banerjee, D., Ghosh, S., 2014. Resi-
lient supplier selection under a fuzzy environment. Int.
J. Manage. Sci. Eng. Manage. 9 (2), 147–156.
Hall, J., 16 Apr 2010. Volcanic Ash Cloud Leaves Shops
Facing Shortages of Fruit, Vegetables and Medicine.
The Daily Telegraph.
Hwang, C.L, Yoon, K., 1981. Multiple attribute decision
making: methods and applications. New York:
Springer-Verlag.
Kannan, D., Govindan, K., Rajendran, S., 2015. Fuzzy
axiomatic design approach based green supplier
selection: a case study from singapore. J. Clean. Prod.
96, 194–208.
Klibi, W.; Martel, A. Scenario-based Supply Chain
Network risk modeling. Eur. J. Oper. Res. 2012, 223,
644–658.
Konur, D., Campbell, J.F., Monfared, J.A., 2016. Economic
and environmental considerations in a stochastic
inventory control model with order splitting under
different delivery schedules among suppliers. Omega,
71, 46–65.
Kuo, R.J., Y.J. Lin. Supplier selection using analytic
network process and data envelopment analysis.
International. Journal of Production Research (2011),
pp. 1-12iFirst.
Mitra, K.; Gudi, R.D.; Patwardhan, S.C.; Sardar, G.
Towards resilient supply chains: Uncertainty analysis
using fuzzy mathematical programming. Chem. Eng.
Res. Des. 2009, 87, 967–981.
Mohammed A. and Wang Q. 2015. Integrity of an RFID-
enabled HMSC Network. Proceedings of the Third
International Conference on Digital Enterprise and
Information Systems, Shenzhen, China, 2015a, 79-86.
Mohammed A. and Wang Q. and Li X. 2017a. Developing
a meat supply chain network design using a multi-
objective possibilistic programming approach. British
Food Journal, 119, 3, 690-706.
Mohammed, A. and Wang, Q., 2017b. The fuzzy multi-
objective distribution planner for a green meat supply
chain. International J. Pro Eco., 184, 47–58
Mohammed, A., Wang, Q., Alyahya, S. and Binnette, N.
2017c. Design and optimization of an RFID-enabled
automated warehousing system under uncertainties: A
multi-criterion fuzzy programming approach. The
International Journal of Advanced Manufacturing
Technology. 91 (5), 1661-1670.
Pramanik, D., Subhash, N., Haldar, A., Mondal, S.C.,
Naskar, S.N., Ray. A., 2017. Resilient supplier
selection using AHP-TOPSIS-QFD under a fuzzy
environment. International Journal of Management
Science and Engineering Management, 12 (1), 45-54.
Purvis, L., Spall, S., Naim, M. and Spiegler, V. 2016.
Developing a resilient supply chain strategy during
‘boom’ and ‘bust’, Production Planning & Control,
27:7-8, 579-590.
Rajesh, R.; Ravi, V. Supplier selection in resilient supply
chains: A grey relational analysis approach. J. Clean.
Prod. 2015, 86, 343–359.
Saaty, T. L. (1977). A scaling method for priorities in
hierarchical structures. Journal of Mathematical
Psychology, 15(3), 234–281.
Evaluating Green and Resilient Supplier Performance: AHP-Fuzzy Topsis Decision-Making Approach
215