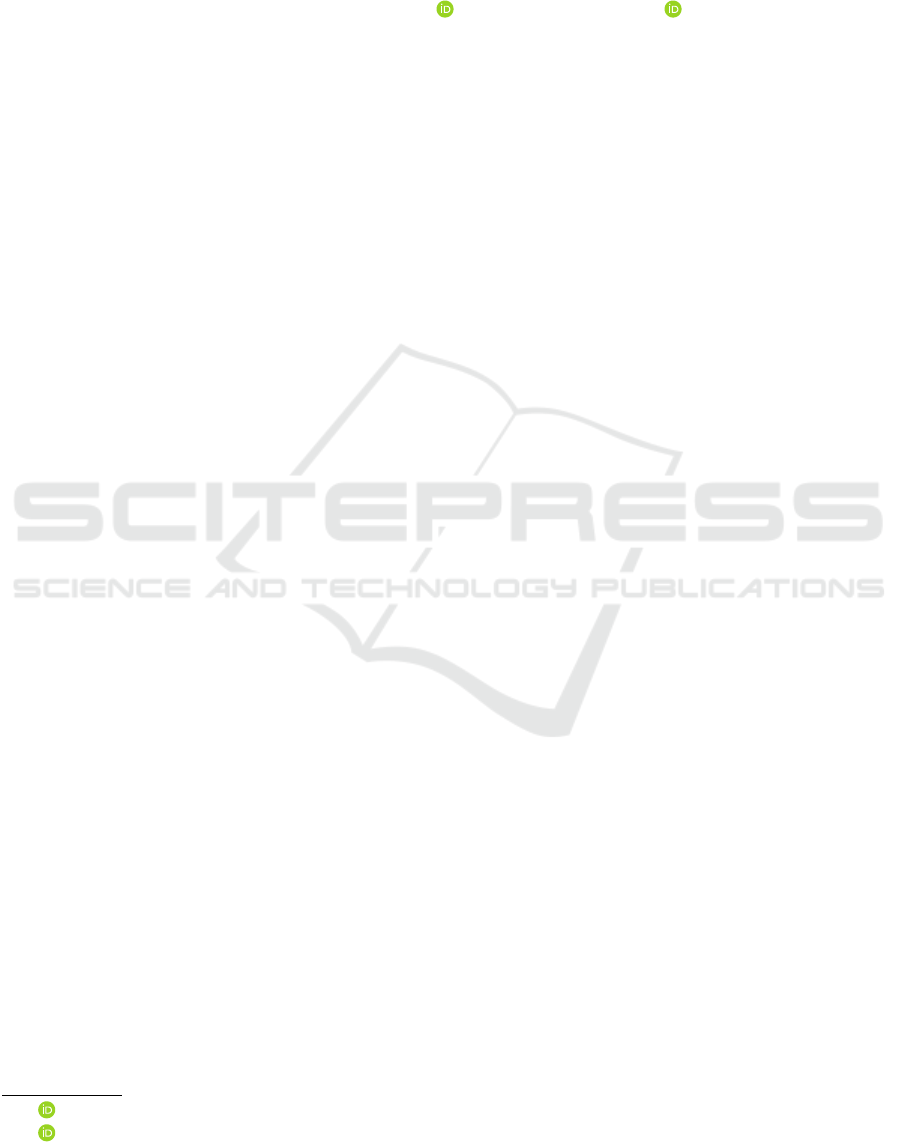
Optimal Social Limitation Reduction under Vaccination and Booster
Doses
Paolo Di Giamberardino
a
and Daniela Iacoviello
b
Department of Computer, Control and Management Engineering, Sapienza University of Rome, Rome, Italy
Keywords:
Epidemic Modeling, COVID-19, Vaccination, Optimal Control, Social Behaviour.
Abstract:
In the paper an optimal control solution is provided for the containment of the number of infected individuals
in COVID-19 pandemic under vaccination campaign. The possibility to dynamically change the cost of the
controls according to the ongoing evolution within the design procedure allows to get great efforts in presence
of very serious disease conditions, saving resources otherwise. The different contribution of vaccinated and
unvaccinated individuals to the epidemic spread is investigated, optimising the controls which describe the
individual contact restrictions separately for the two classes and showing that it would have been possible
to reduce all the social limitations introduced by many governments for the vaccinated individuals since the
beginning of the vaccination campaign.
1 INTRODUCTION
COVID-19 is a sanitary emergency since January
2020 and the scientific community has started to
study the evolution and the spread of the infection
by means of mathematical models which had to fol-
low the changes and the novelties in the knowledge
of the illness, updated day by day, and in the pos-
sibility to fight the virus, as for examples (Di Gi-
amberardino et al., 2021),(Giordano et al., 2020),
(Tang et al., 2020), (Di Giamberardino and Iacoviello,
2021), (Radulescu et al., 2020). In particular, since
the end of 2020, the availability of an effective vac-
cine makes it possible to introduce in all the models a
new important control input, especially for the initial
problem of scheduling the vaccinations among popu-
lation categories. There is for example (Diagne et al.,
2021), with the study of the efficacy of the vaccination
with respect to the amount of population immunized.
There is a great relationship between the level of
immunization of the population and the relax of the
individual containment limitations. The necessity of
a booster dose after the two doses cycle of vaccina-
tions highly increased the people immunization, al-
lowing to strongly reduce or even eliminate social re-
strictions in many places. Different control actions
have been proposed in literature to reduce the num-
ber of infected patients; in particular, optimal control
a
https://orcid.org/0000-0002-9113-8608
b
https://orcid.org/0000-0003-3506-1455
allows to allocate efficiently the available limited re-
sources, as in (Silva et al., 2021) referred in particular
to the Portugal situation, where an optimal strategy is
proposed to maximize the number of people that can
return to normal life minimizing the number of in-
fected patients, taking into account the level of hospi-
talization. In (Olivares and Staffetti, 2021) the effects
of various vaccination strategies are studied consid-
ering realistic scenarios in which constraints on vac-
cine administration are introduced. Optimal solutions
for vaccination strategies are also proposed improv-
ing existing models by the addition of compartments
which take into account new knowledge on epidemic
behaviour, like in (Liu et al., 2021) with the introduc-
tion of class of people for which the vaccine is inef-
fective.
In this paper, following the approach described in
(Di Giamberardino and Iacoviello, 2017), it is pro-
posed an optimal control obtained minimizing a suit-
able cost index in which the controls are weighted
on the basis of the severity of the pandemic situa-
tion. In particular, this approach is used to act on the
most invasive containment measure represented by
the individual contact limitations with consequences
on the everyday life and economy. A new mathemat-
ical model, which takes into account the possibility
of a periodic reiteration with respect to the vaccina-
tion procedure, is here proposed as an improvement of
previously adopted ones to better fit the present epi-
demic conditions.
38
Di Giamberardino, P. and Iacoviello, D.
Optimal Social Limitation Reduction under Vaccination and Booster Doses.
DOI: 10.5220/0011277500003271
In Proceedings of the 19th International Conference on Informatics in Control, Automation and Robotics (ICINCO 2022), pages 38-48
ISBN: 978-989-758-585-2; ISSN: 2184-2809
Copyright
c
2022 by SCITEPRESS – Science and Technology Publications, Lda. All rights reserved