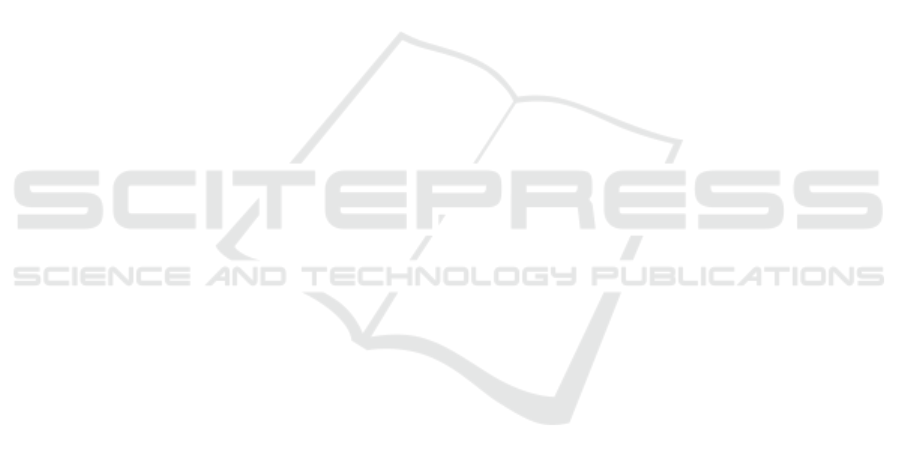
vides letters with phrases and emotions that reflect the
users’ experience. On the other hand, users also com-
mented it limits users to adapt their perception to the
available cards.
From our point of view, we believe that the meth-
ods complemented each other. AttrakDiff measures
UX through hedonic and pragmatic chatbot, compar-
ing users’ expectations and experience. However, be-
cause it is a scale-type questionnaire, users cannot ef-
fectively point out UX problems since this can only
be identified during interaction with the chatbot. The
Thinking Aloud method helped us to get explicit feed-
back from users with suggestions that can help de-
signers improve the chatbot. In AttrakDiff, the user
only chose among adjectives, and in Think Aloud,
the user was concerned with reporting their actions.
Complementarily, MAX helped users express them-
selves more openly about their user emotions, how
easy and helpful it was to use the chatbot, and their
intention to use it again. Therefore, we believe that
the three methods were essential to capture the whole
experience of users when using the chatbot. A limi-
tation of this research was that we evaluated from the
perspective of only one chatbot, and the usability of
UX methods depended only on this artifact.
Overall, we hope that the results of this study will
help promote and improve current practice and re-
search on UX in chatbots. In addition, we hope that
suggestions for improvements can contribute to the
evolving ANA chatbot. This work opens the possi-
bility for different relevant results: What are the main
factors that positively and negatively influence the ex-
perience of chatbot users? How can UX methods be
designed to capture the UX better? Is it better to adopt
quantitative, qualitative, or mixed metrics to evaluate
UX? How can we adopt machine learning to automate
UX assessments? In this sense, as future work, we
intend to carry out new UX evaluations with differ-
ent types of chatbots to verify a divergence between
the generated UX, adopting other UX methods, with
a larger sample, and in other domains (educational,
health, commerce).
REFERENCES
Alhadreti, O. and Mayhew, P. (2018). Rethinking thinking
aloud: A comparison of three think-aloud protocols.
In Proceedings of the 2018 CHI Conference on Hu-
man Factors in Computing Systems, pages 1–12.
Baravalle, A. and Lanfranchi, V. (2003). Remote web us-
ability testing. Behavior Research Methods, Instru-
ments, & Computers, 35(3):364–368.
Cavalcante, E., Rivero, L., and Conte, T. (2015). Max:
A method for evaluating the post-use user experience
through cards and a board. In 27th International Con-
ference on Software Engineering and Knowledge En-
gineering (SEKE 2015), pages 495–500.
Chagas, B. A., Ferreguetti, K., Ferreira, T. C., Prates, R. O.,
Ribeiro, L. B., Pagano, A. S., Reis, Z. S., and Meira Jr,
W. (2021). Chatbot as a telehealth intervention strat-
egy in the covid-19 pandemic: Lessons learned from
an action research approach. CLEI electronic journal,
24(3).
da Silva Franco, R. Y., Santos do Amor Divino Lima, R.,
Paixão, M., Resque dos Santos, C. G., Serique Meigu-
ins, B., et al. (2019). Uxmood—a sentiment analysis
and information visualization tool to support the eval-
uation of usability and user experience. Information,
10(12):366.
Fernandes, U. d. S., Prates, R. O., Chagas, B. A., and Bar-
bosa, G. A. (2021). Analyzing molic’s applicability
to model the interaction of conversational agents: A
case study on ana chatbot. In Proceedings of the XX
Brazilian Symposium on Human Factors in Comput-
ing Systems, pages 1–7.
Fiore, D., Baldauf, M., and Thiel, C. (2019). “forgot your
password again?" acceptance and user experience of
a chatbot for in-company it support. In Proceedings
of the 18th International Conference on Mobile and
Ubiquitous Multimedia, pages 1–11.
Følstad, A., Nordheim, C. B., and Bjørkli, C. A. (2018).
What makes users trust a chatbot for customer ser-
vice? an exploratory interview study. In Interna-
tional conference on internet science, pages 194–208.
Springer.
Følstad, A. and Skjuve, M. (2019). Chatbots for customer
service: user experience and motivation. In Proceed-
ings of the 1st international conference on conversa-
tional user interfaces, pages 1–9.
Guerino, G. C., Silva, W. A. F., Coleti, T. A., and Valentim,
N. M. C. (2021). Assessing a technology for usabil-
ity and user experience evaluation of conversational
systems: An exploratory study. In Proceedings of the
23rd International Conference on Enterprise Informa-
tion Systems (ICEIS 2021), volume 2, pages 461–471.
Hassenzahl, M., Burmester, M., and Koller, F.
(2003). Attrakdiff: Ein fragebogen zur messung
wahrgenommener hedonischer und pragmatischer
qualität. In Mensch & computer 2003, pages 187–196.
Springer.
ISO9241-210 (2011). Iso / iec 9241-210: Ergonomics of
human-system interaction – part 210: Human-centred
design for interactive systems.
Jain, M., Kumar, P., Kota, R., and Patel, S. N. (2018). Eval-
uating and informing the design of chatbots. In Pro-
ceedings of the 2018 Designing Interactive Systems
Conference, pages 895–906.
Lewis, J. R. and Sauro, J. (2021). Usability and user expe-
rience: Design and evaluation. Handbook of Human
Factors and Ergonomics, pages 972–1015.
Luger, E. and Sellen, A. (2016). " like having a really bad
pa" the gulf between user expectation and experience
of conversational agents. In Proceedings of the 2016
CHI conference on human factors in computing sys-
tems, pages 5286–5297.
ICEIS 2022 - 24th International Conference on Enterprise Information Systems
362