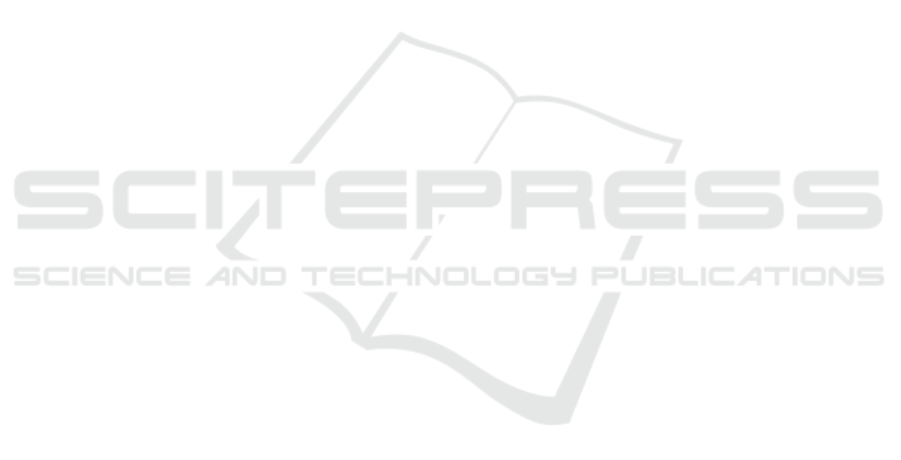
machine learning models and algorithms for open sys-
tem fidelity. Procedia Computer Science, 32:834–841.
Egala, B. S., Pradhan, A. K., Badarla, V. R., and Mohanty,
S. P. (2021). Fortified-chain: A blockchain based
framework for security and privacy assured internet
of medical things with effective access control. IEEE
Internet of Things Journal.
Engels, J. I. (2018). Key Concepts for Critical Infrastruc-
ture Research. Springer.
EU (2008). Council directive 2008/114/ec on the identifi-
cation and designation of european critical infrastruc-
tures and the assessment of the need to improve their
protection. L345, 3:0075–0082.
Frison, C., van Zimmeren, E., and Gils, T. (2020).
Blockchain technology for ip management & gover-
nance: Exploring its potential to restore trust and re-
silience in the plant and biomedical sectors. Current
Opinion in Environmental Sustainability.
Gheorghe, A. V., Vamanu, D. V., Katina, P. F., and Pulfer,
R. (2018). Critical infrastructures, key resources, and
key assets. In Critical Infrastructures, Key Resources,
Key Assets, pages 3–37. Springer.
Gils, T. and Frison, C. (2020). Blockchain technology for
food security? resilience potential and risk identifi-
cation for the multilateral system of the international
treaty on plant genetic resources for food and agri-
culture. Resilience Potential and Risk Identification
for the Multilateral System of the International Treaty
on Plant Genetic Resources for Food and Agriculture
(September 1, 2020). IUCN AEL Proceedings.
Guan, Z., Lu, X., Yang, W., Wu, L., Wang, N., and Zhang,
Z. (2021). Achieving efficient and privacy-preserving
energy trading based on blockchain and abe in smart
grid. Journal of Parallel and Distributed Computing,
147:34–45.
Gupta, R., Tanwar, S., Kumar, N., and Tyagi, S. (2020).
Blockchain-based security attack resilience schemes
for autonomous vehicles in industry 4.0: A system-
atic review. Computers & Electrical Engineering,
86:106717.
Henry, D. and Ramirez-Marquez, J. E. (2012). Generic met-
rics and quantitative approaches for system resilience
as a function of time. Reliability Engineering & Sys-
tem Safety, 99:114–122.
Hossain, M. M., Hasan, R., and Zawoad, S. (2017). Trust-
iov: A trustworthy forensic investigation framework
for the internet of vehicles (iov). In ICIOT, pages 25–
32.
Ibitoye, O., Abou-Khamis, R., Matrawy, A., and Shafiq,
M. O. (2019). The threat of adversarial attacks on
machine learning in network security–a survey. arXiv
preprint arXiv:1911.02621.
Jarmon, J. A. (2019). The New Era in US National Secu-
rity: Challenges of the Information Age. Rowman &
Littlefield Publishers.
Jetley, H. (2019). Blockchain implementation for smart grid
resilience. In Proceedings of the International Annual
Conference of the American Society for Engineering
Management., pages 1–9. American Society for Engi-
neering Management (ASEM).
Jian, X., Leng, P., Wang, Y., Alrashoud, M., and Hossain,
M. S. (2021). Blockchain-empowered trusted net-
working for unmanned aerial vehicles in the b5g era.
IEEE Network, 35(1):72–77.
Johnson, J. and Gheorghe, A. V. (2013). Antifragility anal-
ysis and measurement framework for systems of sys-
tems. International Journal of Disaster Risk Science,
4(4):159–168.
Kahan, J. H., Allen, A. C., and George, J. K. (2009). An op-
erational framework for resilience. Journal of Home-
land Security and Emergency Management, 6(1).
Karadimas, A., Hewig, E., Behera, S., and Kotisi, T. (2014).
Case study of black swans and antifragility. Semantic
Scholar, pages 1–17.
Kendzierskyj, S. and Jahankhani, H. (2019). The role of
blockchain in supporting critical national infrastruc-
ture. In 2019 IEEE 12th International Conference on
Global Security, Safety and Sustainability (ICGS3),
pages 208–212. IEEE.
Klein, L. (2020). Governing critical infrastructure in digi-
tal futures. In Sustainable Development and Resource
Productivity, pages 182–192. Routledge.
Kotsiuba, I., Velykzhanin, A., Biloborodov, O., Skarga-
Bandurova, I., Biloborodova, T., Yanovich, Y., and
Zhygulin, V. (2018). Blockchain evolution: from bit-
coin to forensic in smart grids. In 2018 IEEE Inter-
national Conference on Big Data (Big Data), pages
3100–3106. IEEE.
Liu, Y., Yuan, X., Xiong, Z., Kang, J., Wang, X., and Niy-
ato, D. (2020). Federated learning for 6g communi-
cations: Challenges, methods, and future directions.
China Communications, 17(9):105–118.
Lusetti, M., Salsi, L., and Dallatana, A. (2020). A
blockchain based solution for the custody of digital
files in forensic medicine. Forensic Science Interna-
tional: Digital Investigation, 35:301017.
Mahzarnia, M., Moghaddam, M. P., Baboli, P. T., and Siano,
P. (2020). A review of the measures to enhance power
systems resilience. IEEE Systems Journal.
Malamas, V., Dasaklis, T., Kotzanikolaou, P., Burmester,
M., and Katsikas, S. (2019). A forensics-by-design
management framework for medical devices based on
blockchain. In 2019 IEEE World Congress on Services
(SERVICES), volume 2642, pages 35–40. IEEE.
Martinetti, A., Chatzimichailidou, M. M., Maida, L., and
van Dongen, L. (2019). Safety i–ii, resilience and an-
tifragility engineering: a debate explained through an
accident occurring on a mobile elevating work plat-
form. International journal of occupational safety and
ergonomics, 25(1):66–75.
Mbarek, B., Chren, S., Rossi, B., Pitner, T., et al. (2020). An
enhanced blockchain-based data management scheme
for microgrids. In AINA Workshops, pages 766–775.
Miller, K. W., Morell, L. J., Noonan, R. E., Park, S. K.,
Nicol, D. M., Murrill, B. W., and Voas, M. (1992). Es-
timating the probability of failure when testing reveals
no failures. IEEE transactions on Software Engineer-
ing, 18(1):33.
Mohay, G. (2005). Technical challenges and directions for
digital forensics. In First International Workshop on
IoTBDS 2022 - 7th International Conference on Internet of Things, Big Data and Security
86