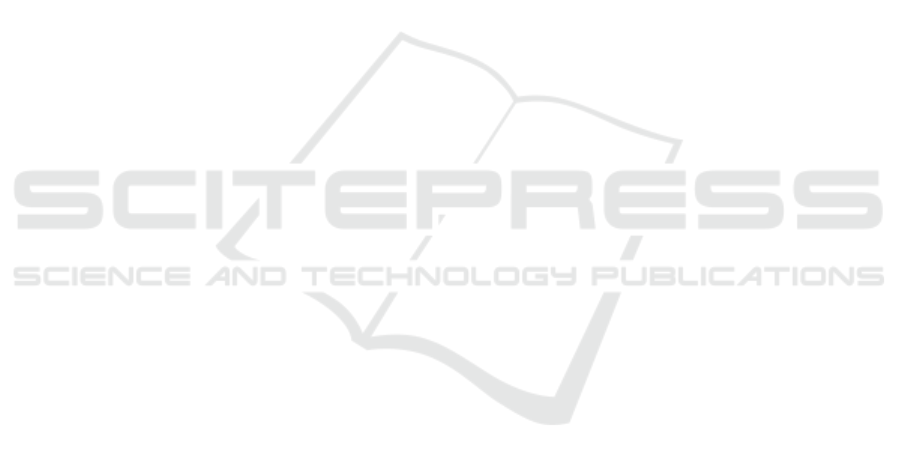
ACKNOWLEDGMENTS
This research was partially supported by the project
that has received funding from the European Union’s
Horizon 2020 Research and Innovation Programme
under Grant Agreement No 739578 and the Govern-
ment of the Republic of Cyprus through the Deputy
Ministry of Research, Innovation and Digital Policy.
REFERENCES
Barbero, l. and Grosse-Wentrup, M. (2010). Biased feed-
back in brain-computer interfaces. Journal of Neuro-
Engineering and Rehabilitation, 7(1):34.
Blanke, O. and Metzinger, T. (2009). Full-body illusions
and minimal phenomenal selfhood. Trends in Cogni-
tive Sciences, 13(1):7–13.
Botvinick, M. and Cohen, J. (1998). Rubber hands’
feel’touch that eyes see. Nature, 391(6669):756.
de Freitas, S. (2011). Technology: Game for change. Na-
ture, 470:330–331.
Deterding, S., Dixon, D., Khaled, R., and Nacke, L. (2011).
From game design elements to gamefulness: defin-
ing” gamification”. In Proceedings of the 15th inter-
national academic MindTrek conference: Envisioning
future media environments, pages 9–15.
Dickhaus, T., Sannelli, C., M
¨
uller, K.-R., Curio, G., and
Blankertz, B. (2009). Predicting BCI performance to
study BCI illiteracy. BMC Neuroscience, 10(Suppl
1):P84.
Faller, J., Vidaurre, C., Solis-Escalante, T., Neuper, C., and
Scherer, R. (2012). Autocalibration and Recurrent
Adaptation: Towards a Plug and Play Online ERD-
BCI. IEEE Transactions on Neural Systems and Re-
habilitation Engineering, 20(3):313–319.
Gallagher, S. (2000). Philosophical conceptions of the self:
implications for cognitive science. Trends in Cogni-
tive Sciences, 4(1):14–21.
Gallagher, S. (2007). The Natural Philosophy of Agency.
Philosophy Compass, 2(2):347–357.
Graimann, B., Allison, B., and Pfurtscheller, G., editors
(2010). Brain-computer interfaces: revolutionizing
human-computer interaction. Frontiers collection.
Springer, Heidelberg. OCLC: ocn707710772.
Hamari, J., Koivisto, J., and Sarsa, H. (2014). Does Gam-
ification Work? – A Literature Review of Empirical
Studies on Gamification. In 2014 47th Hawaii Inter-
national Conference on System Sciences, pages 3025–
3034.
Jeannerod, M. (2003). The mechanism of self-recognition
in humans. Behavioural brain research, 142(1):1–15.
Jeunet, C., Jahanpour, E., and Lotte, F. (2016a). Why stan-
dard brain-computer interface (BCI) training proto-
cols should be changed: an experimental study. Jour-
nal of neural engineering, 13(3):036024.
Jeunet, C., N’Kaoua, B., and Lotte, F. (2016b). Advances in
user-training for mental-imagery-based BCI control:
Psychological and cognitive factors and their neural
correlates. Progress in brain research, 228:3–35.
Kaiser, V., Bauernfeind, G., Kreilinger, A., Kaufmann, T.,
K
¨
ubler, A., Neuper, C., and M
¨
uller-Putz, G. R. (2014).
Cortical effects of user training in a motor imagery
based brain–computer interface measured by fNIRS
and EEG. NeuroImage, 85:432–444.
Kerous, B., Skola, F., and Liarokapis, F. (2017). EEG-based
BCI and video games: a progress report. Virtual Re-
ality.
Kilteni, K., Groten, R., and Slater, M. (2012). The Sense of
Embodiment in Virtual Reality. Presence, 21(4):373–
387.
ˇ
Skola, F. and Liarokapis, F. (2018). Embodied VR environ-
ment facilitates motor imagery brain–computer inter-
face training. Computers & Graphics, 75:59–71.
ˇ
Skola, F., Tinkov
´
a, S., and Liarokapis, F. (2019). Progres-
sive Training for Motor Imagery Brain-Computer In-
terfaces Using Gamification and Virtual Reality Em-
bodiment. Frontiers in Human Neuroscience, 13:329.
Kondo, T., Saeki, M., Hayashi, Y., Nakayashiki, K., and
Takata, Y. (2015). Effect of instructive visual stimuli
on neurofeedback training for motor imagery-based
brain-computer interface. Human Movement Science,
43:239–249.
Kosmyna, N. and L
´
ecuyer, A. (2017). Designing Guiding
Systems for Brain-Computer Interfaces. Frontiers in
Human Neuroscience, 11.
Longo, M. R., Sch
¨
u
¨
ur, F., Kammers, M. P. M., Tsakiris,
M., and Haggard, P. (2008). What is embodiment? A
psychometric approach. Cognition, 107(3):978–998.
Lotte, F., Bougrain, L., and Clerc, M. (2015). Electroen-
cephalography (EEG)-Based Brain–Computer Inter-
faces. Wiley Encyclopedia of Electrical and Electron-
ics Engineering.
Lotte, F., Larrue, F., and Muehl, C. (2013). Flaws in cur-
rent human training protocols for spontaneous Brain-
Computer Interfaces: lessons learned from instruc-
tional design. Frontiers in Human Neuroscience,
7:568.
Meehan, M., Insko, B., Whitton, M., and Brooks, Jr., F. P.
(2002). Physiological Measures of Presence in Stress-
ful Virtual Environments. In Proceedings of the 29th
Annual Conference on Computer Graphics and Inter-
active Techniques, SIGGRAPH ’02, pages 645–652,
New York, NY, USA. ACM.
M
¨
uhl, C., Allison, B., Nijholt, A., and Chanel, G. (2014). A
survey of affective brain computer interfaces: princi-
ples, state-of-the-art, and challenges. Brain-Computer
Interfaces, 1(2):66–84.
Salisbury, D. B., Dahdah, M., Driver, S., Parsons, T. D.,
and Richter, K. M. (2016). Virtual reality and brain
computer interface in neurorehabilitation. Proceed-
ings (Baylor University. Medical Center), 29(2):124–
127.
ˇ
Skola, F. (2020). Reducing the motor imagery brain-
computer interface training time with embodiment in
virtual reality.
Slater, M. (2009). Place illusion and plausibility can lead to
realistic behaviour in immersive virtual environments.
VISIGRAPP 2022 - 17th International Joint Conference on Computer Vision, Imaging and Computer Graphics Theory and Applications
16