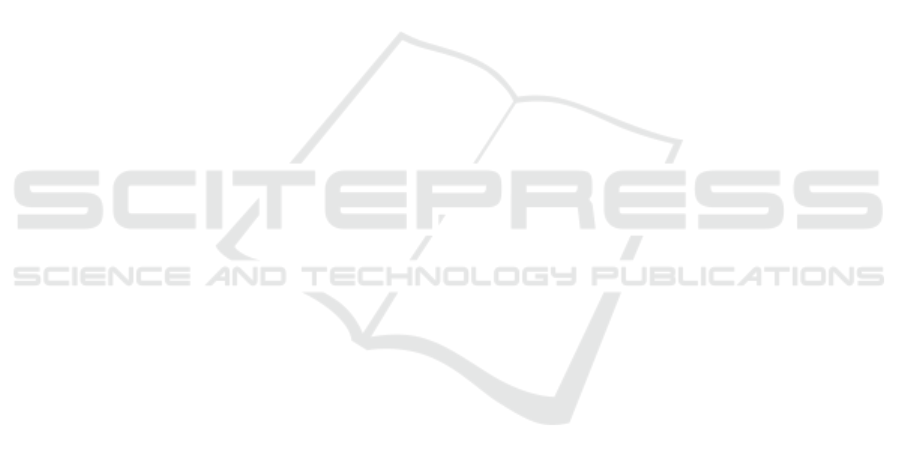
data do not differentiate the portion bought from
online or conventional channels. Moreover, the
generalization of the finding is limited to Indonesian
provinces. Further studies for countries with growing
online food delivery services could generalize.
Managers of OFD platforms might use the finding
to decide which provinces to focus on for their
marketing strategy. Similarly, as a prominent actor
for ICT development, the government might use the
result to formulate a better plan to improve ICT
access. The growth of OFD, especially the Platform-
to-Consumer segment, will give a big multiplier
economic impact, at least for food vendors and
delivery people. In addition, increasing food
consumption could heighten obesity or diet-related
disease in society. Therefore, the government and
OFD platforms should promote healthy food eating to
improve public health.
REFERENCES
Alalwan, A. A. (2020). Mobile food ordering apps: An
empirical study of the factors affecting customer e-
satisfaction and continued intention to reuse.
International Journal of Information Management, 50,
28–44.
Antonio Fernández-Portillo, Almodóvar-González, M., &
Hernández-Mogollón, R. (2020). Impact of ICT
development on economic growth. A study of OECD
European union countries. Technology in Society,
63(101420).
Appiah-Otoo, I., & Song, N. (2021). The impact of ICT on
economic growth: Comparing rich and poor countries.
Telecommunications Policy, 45(2), 102082.
Bahrini, R., & Qaffas, A. A. (2019). Impact of information
and communication technology on economic growth:
Evidence from developing countries. Economies, 7(1).
Bates, S., Bates, S., Reeve, B., & Trevena, H. (2020). A
narrative review of online food delivery in Australia:
Challenges and opportunities for public health nutrition
policy. Public Health Nutrition, 5.
Du, Z., Fan, Z. P., & Gao, G. X. (2021). Choice of O2O
Food Delivery Mode: Self-built Platform or Third-
Party Platform? Self-Delivery or Third-Party Delivery?
IEEE Transactions on Engineering Management, May.
Gavilan, D., Balderas-Cejudo, A., Fernández-Lores, S., &
Martinez-Navarro, G. (2021). Innovation in online food
delivery: Learnings from COVID-19. International
Journal of Gastronomy and Food Science,
24(February).
ITU. (2017). The ICT Development Index (IDI): conceptual
framework and methodology. International
Telecommunication Union.
Kaur, P., Dhir, A., Talwar, S., & Ghuman, K. (2020). The
value proposition of food delivery apps from the
perspective of theory of consumption value.
International Journal of Contemporary Hospitality
Management, 33(4), 1129–1159.
Keeble, M., Adams, J., Sacks, G., Vanderlee, L., White, C.
M., Hammond, D., & Burgoine, T. (2020). Use of
online food delivery services to order food prepared
away-from-home and associated sociodemographic
characteristics: A cross-sectional, multi-country
analysis. International Journal of Environmental
Research and Public Health, 17(14), 1–17.
Li, C., Mirosa, M., & Bremer, P. (2020). Review of online
food delivery platforms and their impacts on
sustainability. Sustainability (Switzerland), 12(14), 1–
17.
Maimaiti, M., Zhao, X., Jia, M., Ru, Y., & Zhu, S. (2018).
How we eat determines what we become: opportunities
and challenges brought by food delivery industry in a
changing world in China. European Journal of Clinical
Nutrition, 72(9), 1282–1286.
Martinez-Plumed, F., Contreras-Ochando, L., Ferri, C.,
Hernandez Orallo, J., Kull, M., Lachiche, N., Ramirez
Quintana, M. J., & Flach, P. A. (2019). CRISP-DM
Twenty Years Later: From Data Mining Processes to
Data Science Trajectories. IEEE Transactions on
Knowledge and Data Engineering, 33(8), 3048–3061.
Mokhtarian, P. L., Salomon, I., & Handy, S. L. (2006). The
impacts of ICT on leisure activities and travel: A
conceptual exploration. Transportation, 33(3), 263–
289.
Statista. (2021a). Online Food Delivery - Worldwide.
Statista.com.
https://www.statista.com/outlook/dmo/eservices/onlin
e-food-delivery/worldwide?currency=usd
Statista. (2021b). Most used food delivery app orders
Indonesia 2021. https://www.statista.com/statistics/
1149349/indonesia-favorite-food-delivery-apps/
Statista. (2021c). Online Food Delivery - Indonesia.
https://www.statista.com/outlook/dmo/eservices/onlin
e-food-delivery/indonesia
Suhartanto, D., Ali, M. H., Tan, K. H., Sjahroeddin, F., &
Kusdibyo, L. (2019). Loyalty toward online food
delivery service: the role of e-service quality and food
quality. Journal of Foodservice Business Research,
22(1), 2–3.
Suhartanto, D., Dean, D., Leo, G., & Triyuni, N. N. (2019).
Millennial Experience With Online Food Home
Delivery. Interdisciplinary Journal of Information,
Knowledge, and Management, 14, 277–279.
Vu, K., Hanafizadeh, P., & Bohlin, E. (2020). ICT as a
driver of economic growth: A survey of the literature
and directions for future research. Telecommunications
Policy, 44(2), 101922.
Xie, J., Xu, Y., & Li, H. (2021). Environmental impact of
express food delivery in China: the role of personal
consumption choice. Environment, Development, and
Sustainability, 23(6), 8234–8251.
Zhang, S., Pauwels, K., & Peng, C. (2019). The Impact of
Adding Online-to-Offline Service Platform Channels
on Firms' Offline and Total Sales and Profits. Journal
of Interactive Marketing, 47, 115–128.
ICEIS 2022 - 24th International Conference on Enterprise Information Systems
178