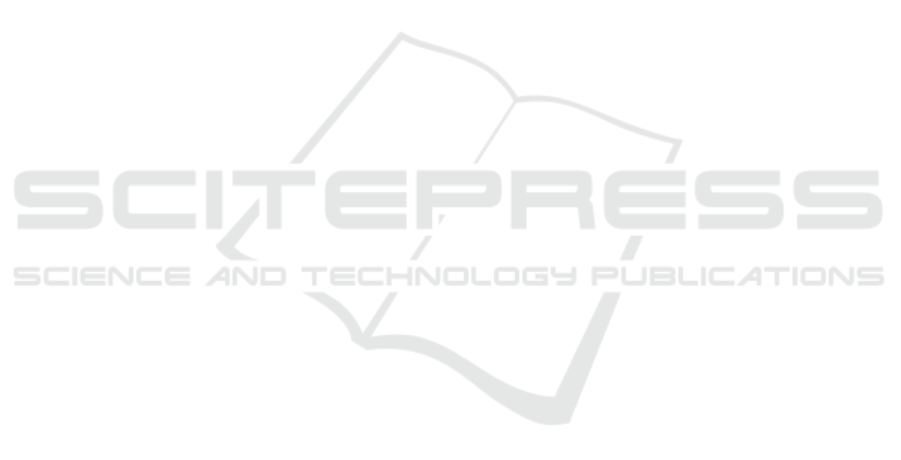
with larger participant samples and possibly lower
dropout rates in longitudinal testing to strengthen the
evidence when answering questions regarding long-
term effects.
6 CONCLUSION
In this paper, we present the results of a compar-
ative user study of an anti-phishing learning game
and its personalized version as well as an analysis
of in-game behavior to understand how personaliza-
tion influences the participants’ gameplay and perfor-
mance. We find, that users interact differently when
confronted with URLs based on services they are not
familiar with, both during gameplay and in the URL
tests of our user study. While we did not find signif-
icant differences in the classification performance of
participants of the personalized and non-personalized
versions of the game, we find some indications that
personalization might potentially have positive effects
on the players’ awareness. Our work therefore moti-
vates further analyses of learning games with person-
alized content and how it affects players during and
after playing the game. Furthermore, we performed
longitudinal testing three months after the game was
played and find, that while the participants’ perfor-
mance seems to drop compared to the post-test, it
is still significantly higher than the pre-test. These
results indicate, that general knowledge about the
URL structure and possible manipulation techniques
can help users detect malicious URLs even several
months after the intervention.
ACKNOWLEDGEMENTS
This research was supported by the research train-
ing group “Human Centered Systems Security” spon-
sored by the state of North Rhine-Westphalia.
REFERENCES
Aleroud, A. and Zhou, L. (2017). Phishing environments,
techniques, and countermeasures: A survey. Comput-
ers & Security, 68:160–196.
APWG (2021). APWG Phishing Activity Trends Report,
3rd Quarter 2021. Technical report, Anti-Phishing
Working Group.
Bull, S. (2004). Supporting learning with open learner mod-
els. Planning, 29(14):1.
Canova, G., Volkamer, M., Bergmann, C., and Reinheimer,
B. (2015). NoPhish app evaluation: Lab and retention
study. In NDSS Workshop on Usable Security 2015,
USEC ’15, San Diego, California. Internet Society.
Dey, R. and Konert, J. (2016). Content Generation for Se-
rious Games. In D
¨
orner, R., G
¨
obel, S., Kickmeier-
Rust, M., Masuch, M., and Zweig, K., editors, En-
tertainment Computing and Serious Games: Interna-
tional GI-Dagstuhl Seminar 15283, Revised Selected
Papers, pages 174–188. Springer, Cham.
Drury, V., Roepke, R., Schroeder, U., and Meyer, U. (2022).
Analyzing and Creating Malicious URLs: A Compar-
ative Study on Anti-Phishing Learning Games. In Us-
able Security and Privacy Symposium 2022, USEC
’22, pages 1–13, San Diego, USA. IEEE. [in publi-
cation].
Kaspersky (2021). Spam and phishing in Q3 2021. Techni-
cal report, Kaspersky.
Kickmeier-Rust, M. D. and Albert, D. (2010). Micro-
adaptivity: Protecting immersion in didactically adap-
tive digital educational games. Journal of Computer
Assisted Learning, 26(2):95–105.
Kumaraguru, P., Sheng, S., Acquisti, A., Cranor, L. F., and
Hong, J. (2008). Lessons from a real world evaluation
of anti-phishing training. In 2008 eCrime Researchers
Summit, pages 1–12.
Lastdrager, E. E. (2014). Achieving a consensual defini-
tion of phishing based on a systematic review of the
literature. Crime Science, 3(1):1–10.
Law, E. L.-C. and Rust-Kickmeier, M. (2008). 80Days:
Immersive Digital Educational Games with Adaptive
Storytelling. In Proceedings of the 1st International
Workshop on Story-Telling and Educational Games,
STEG ’08, pages 56–62, Maastricht, Netherlands.
CEUR.
Roepke, R., Drury, V., Meyer, U., and Schroeder, U.
(2021a). Exploring Different Game Mechanics for
Anti-Phishing Learning Games. In Games and Learn-
ing Alliance, GaLA ’21, Cham. Springer.
Roepke, R., Drury, V., Schroeder, U., and Meyer, U.
(2021b). A Modular Architecture for Personalized
Learning Content in Anti-Phishing Learning Games.
In Software Engineering 2021 Satellite Events, SE-SE
’21, Braunschweig, Germany. CEUR.
Roepke, R., Koehler, K., Drury, V., Schroeder, U., Wolf,
M. R., and Meyer, U. (2020a). A Pond Full of Phish-
ing Games - Analysis of Learning Games for Anti-
Phishing Education. In Hatzivasilis, G. and Ioanni-
dis, S., editors, Model-Driven Simulation and Train-
ing Environments for Cybersecurity, Lecture Notes in
Computer Science, pages 41–60, Cham. Springer.
Roepke, R., Schroeder, U., Drury, V., and Meyer, U.
(2020b). Towards Personalized Game-Based Learn-
ing in Anti-Phishing Education. In 20th Interna-
tional Conference on Advanced Learning Technolo-
gies, ICALT ’20, pages 65–66, Tartu, Estonia. IEEE.
Sheng, S., Magnien, B., Kumaraguru, P., Acquisti, A., Cra-
nor, L. F., Hong, J., and Nunge, E. (2007). Anti-
Phishing Phil: The Design and Evaluation of a Game
That Teaches People Not to Fall for Phish. In Pro-
ceedings of the 3rd Symposium on Usable Privacy and
Security, SOUPS ’07, pages 88–99, New York, USA.
ACM.
CSEDU 2022 - 14th International Conference on Computer Supported Education
466