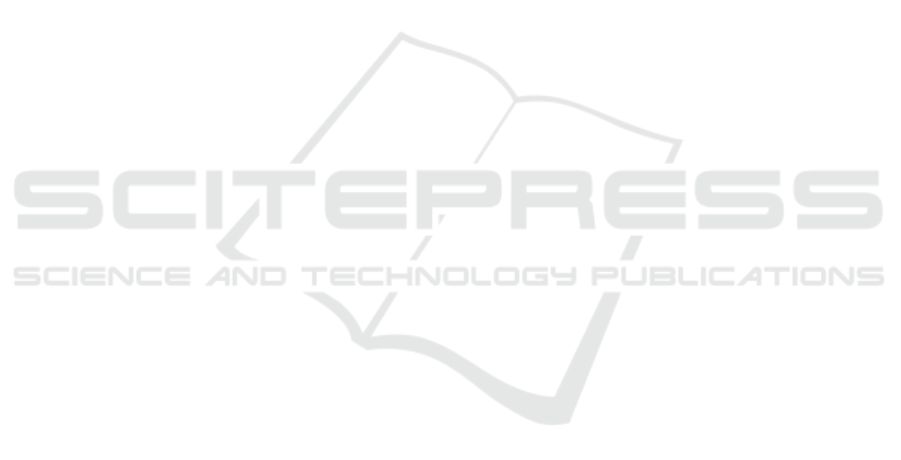
4 CONCLUSIONS
The presented approach of the course ”Artificial Intel-
ligence for Climate Change” was new into the faculty
and for the students, but the feedback and the interest
from both students and specialists invited show that
the idea can be of success also in the next years.
To the best of our knowledge, this approach is
unique among the universities of Romania, in par-
ticular, computer science faculties. Globally how-
ever, this program is implemented across several uni-
versities such as: AI for Social Good from Stan-
ford, Climate Change and AI from Oxford and AI for
the study of Environmental Risks from University of
Cambridge.
In the scientific community there is a great interest
for this topic, ”AI for Good” being the largest confer-
ence to date (https://aiforgood.itu.int/). There are also
working groups consisting of researchers and special-
ists to support the fight against climate change (Fo-
cus Group on Environmental Efficiency for Artificial
Intelligence and other Emerging Technologies, Focus
Group on AI for Natural Disaster Management, Focus
Group on AI for Health, etc.).
As future work directions, our intention is to ex-
tend the list of specialists from different areas, in-
crease the numbers of AI teachers and to organise
some workshop during the laboratory work activity -
engaging also experts from other fields to offer feed-
back in real-time for a new implemented feature.
ACKNOWLEDGEMENTS
The work presented became real with the help of sup-
portive colleagues, extremely passionate guests, and
highly involved students. Therefore we would like to
express our gratitude to Professor Motogna Simona,
Lecturer Alexandru Stermin and specialists Mihai
Cuibus (Romanian Society for Cultural Astronomy),
Veronica Tulpan and Raluca Nicolae (Agent Green),
Sorina Avadanei (Durham University), Daniel Rusen
and Lucian Ungureanu (Microsoft Romania).
The publication of this paper was supported by the
2021 Development Fund of the Babes¸-Bolyai Univer-
sity (UBB).
REFERENCES
Anderson, A. (2012). Climate change education for mitiga-
tion and adaptation. Journal of Education for Sustain-
able Development, 6(2):191–206.
Baeten, M., Kyndt, E., Struyven, K., and Dochy, F. (2010).
Using student-centred learning environments to stim-
ulate deep approaches to learning: Factors encourag-
ing or discouraging their effectiveness. Educational
Research Review, 5(3):243–260.
Briguglio, L. and Moncada, S. (2019). The benefits and
downsides of multidisciplinary education relating to
climate change. Climate Change and the Role of Ed-
ucation, pages 169–187.
Dey, A. (2016). Machine learning algorithms: a review.
International Journal of Computer Science and Infor-
mation Technologies, 7(3):1174–1179.
Dias Canedo, E., Santos, G. A., and Andrade de Fre-
itas, S. A. (2017). Analysis of the teaching-learning
methodology adopted in the introduction to computer
science classes. In 2017 IEEE Frontiers in Education
Conference (FIE), pages 1–8.
Falchi, F., Cinzano, P., Duriscoe, D., Kyba, C. C., Elvidge,
C. D., Baugh, K., Portnov, B. A., Rybnikova, N. A.,
and Furgoni, R. (2016). The new world atlas of
artificial night sky brightness. Science advances,
2(6):e1600377.
Famili, A., Shen, W.-M., Weber, R., and Simoudis, E.
(1997). Data preprocessing and intelligent data anal-
ysis. Intelligent data analysis, 1(1):3–23.
Griffiths, G., Oates, B. J., and Lockyer, M. (2007). Evolving
a facilitation process towards student centred learning:
A case study in computing. Journal of Information
Systems Education, 18(4):459.
Lu, Z., Hou, L., and Huang, X. (2010). A research on a
student-centred teaching model in an ict-based english
audio-video speaking class. International Journal
of Education and Development Using ICT, 6(3):101–
123.
Microsoft (2021). Light pollution maps.
Morris, H. (2014). Socioscientific issues and multidisci-
plinarity in school science textbooks. International
Journal of Science Education, 36(7):1137–1158.
Perez, F. and Granger, B. E. (2015). Project jupyter: Com-
putational narratives as the engine of collaborative
data science. Retrieved September, 11(207):108.
Perez, L. and Wang, J. (2017). The effectiveness of data
augmentation in image classification using deep learn-
ing. arXiv preprint arXiv:1712.04621.
Ruiter, D. J., van Kesteren, M. T., and Fernandez, G. (2012).
How to achieve synergy between medical education
and cognitive neuroscience? an exercise on prior
knowledge in understanding. Advances in health sci-
ences education, 17(2):225–240.
Xu, Y. and Goodacre, R. (2018). On splitting training
and validation set: A comparative study of cross-
validation, bootstrap and systematic sampling for es-
timating the generalization performance of supervised
learning. Journal of Analysis and Testing, 2.
Collaborative Transdisciplinary Educational Approaches in AI
267