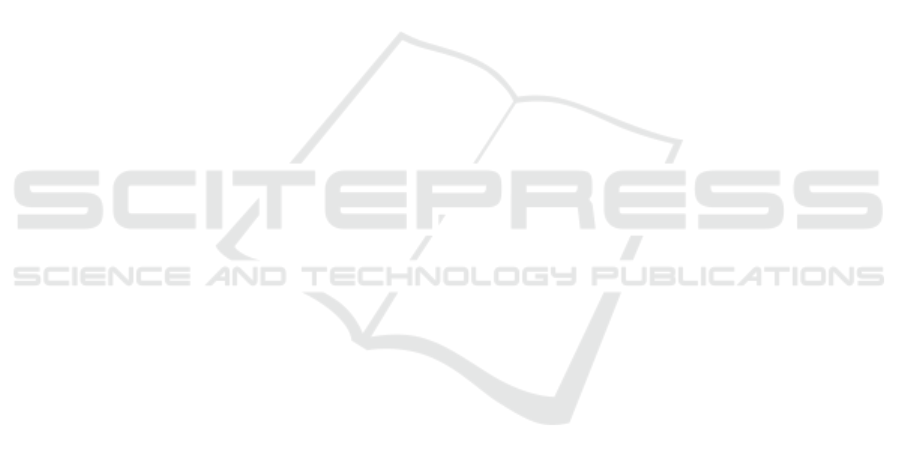
be an appropriate measurement to describe the qual-
ity ratings of instructional videos. Other investigated
methods are still missing. Currently, however, eval-
uating a video’s quality is still best carried out by
hand and with various measurement tools that target
the content of the instructional video. It is, therefore,
necessary to take into account the content orientation
of the instructional video. For this purpose, there are
already various evaluation approaches from profes-
sional disciplines (Hartung, 2020; Kulgemeyer, 2020;
Okagbue et al., 2020; Uebing, 2019). The question is
whether it would be possible to develop a framework
that is either so modular that it can be adapted depend-
ing on the subject area or so abstract that different
subject areas are thereby taken into account. How-
ever, what features reliably make up a good instruc-
tional video remains to be examined. Many things
can be studied for this purpose (e.g., eye tracking).
Moreover, the click behavior when searching for and
watching the videos (Fyfield et al., 2021) could be in-
vestigated. One of the most promising approaches
seems to be comments. In this regard, Kulgemeyer
and Peters (2016) already noted connections between
physical instructional videos found to be good and the
number of content-related comments.
In general, a current development is important to
note. Youtube recently abolished the possibility to see
the number of dislikes for a video. This means that
further research on possible influences of dislikes for
the quality perception of instructional videos can no
longer be conducted directly. In principle, however,
there is a great need for research in order to be able to
describe the quality perception of instructional videos
in more detail.
REFERENCES
Alphabet Investor Relations. Alphabet q1 2021 earnings
call.
Bernickel, R. Mobilit
¨
at - Arbeit - Gesundheit: Zahlen,
Daten, Fakten [Mobility - Work - Health: Fig-
ures, Data, Facts]. Number 2020 in BKK Gesund-
heitsreport. Medizinisch Wissenschaftliche Verlags-
gesellschaft.
Cetin, A. Evaluation of YouTube video content related to
the management of hypoglycemia. 13(1):7.
Czysz, A. Zahl der arztbesuche und h
¨
aufigste krankheiten
in deutschland [number of doctor visits and most com-
mon diseases in germany].
Erdem, H. and Sisik, A. The reliability of bariatric surgery
videos in YouTube platform. 28(3):712–716.
Fyfield, M., Henderson, M., and Phillips, M. Navigating
four billion videos: teacher search strategies and the
YouTube algorithm. 46(1):47–59. Publisher: Taylor
& Francis.
Giffey, F. BMFSFJ - Ministerin Giffey: Einsamkeit ernst
nehmen [Minister Giffey: Taking loneliness seri-
ously].
Hartung, T. Geschichtsdarstellungen auf YouTube –
geschichtsdidaktische Prinzipien f
¨
ur die Beurteilung
von Erkl
¨
arvideos [Representations of history on
YouTube - historical didactic principles for the assess-
ment of explainer videos]. page 99.
Heidemann, C., Scheidt-Nave, C., Beyer, A.-K., Baumert,
J., Thamm, R., Maier, B., Neuhauser, H., Fuchs, J.,
Kuhnert, R., and Hapke, U. Gesundheitliche lage
von erwachsenen in deutschland – ergebnisse zu aus-
gew
¨
ahlten indikatoren der studie GEDA 2019/2020-
EHIS [health situation of adults in germany - results
on selected indicators of the study GEDA 2019/2020-
EHIS].
Kahane, K., Sherman, J., and Patella, S. Public health’s call
to the people: Media misinformation matters. (28).
Khan, M. L. Social media engagement: What motivates
user participation and consumption on YouTube?
66:236–247.
Kulgemeyer, C. Erkl
¨
aren im physikunterricht [explaining
in physics classes]. In Kircher, E., Girwidz, R., and
Fischer, H. E., editors, Physikdidaktik | Grundlagen,
pages 403–426. Springer.
Kulgemeyer, C. A framework of effective science explana-
tion videos informed by criteria for instructional ex-
planations. 50(6):2441–2462.
Kulgemeyer, C. and Peters, C. Exploring the explaining
quality of physics online explanatory videos. 37:1–
14.
Leopoldina, acatech – Deutsche Akademie der Technik-
wissenschaften, and Union der deutschen Akademien
der Wissenschaften. Public health in deutschland
– strukturen, entwicklungen und globale heraus-
forderungen [Public Health in Germany - Structures,
Developments and Global Challenges].
Long, P. and Siemens, G. Penetrating the fog: Analytics in
learning and education.
Mayer, R. The Cambridge Handbook of Multimedia Learn-
ing. Cambridge University Press. Google-Books-ID:
SSLdo1MLIywC.
Okagbue, H., Oguntunde, P. E., Bishop, S., Obasi, E. C. M.,
Opanuga, A., and Ogundile, O. P. Review on the reli-
ability of medical contents on YouTube.
Papamitsiou, Z. and Economides, A. A. Learning analytics
and educational data mining in practice: A systematic
literature review of empirical evidence. 17(4):49–64.
Publisher: International Forum of Educational Tech-
nology & Society.
Schwartz, F., Walter, U., Johannes, S., Kolip, P., Leidl, R.,
Dierks, M.-L., Busse, R., Schneider, and Nils (2012).
Public Health: Gesundheit und Gesundheitswesen
[Public health : Health and health care].
Shoufan, A. Estimating the cognitive value of YouTube’s
educational videos: A learning analytics approach.
92:450–458.
Thurston, M. Key Themes in Public Health. Routledge.
Uebing, J. Geschichte in 10 Minuten – Wie geht das? Ein
Vorschlag zur Analyse von historischen Erkl
¨
arvideos
CSEDU 2022 - 14th International Conference on Computer Supported Education
240