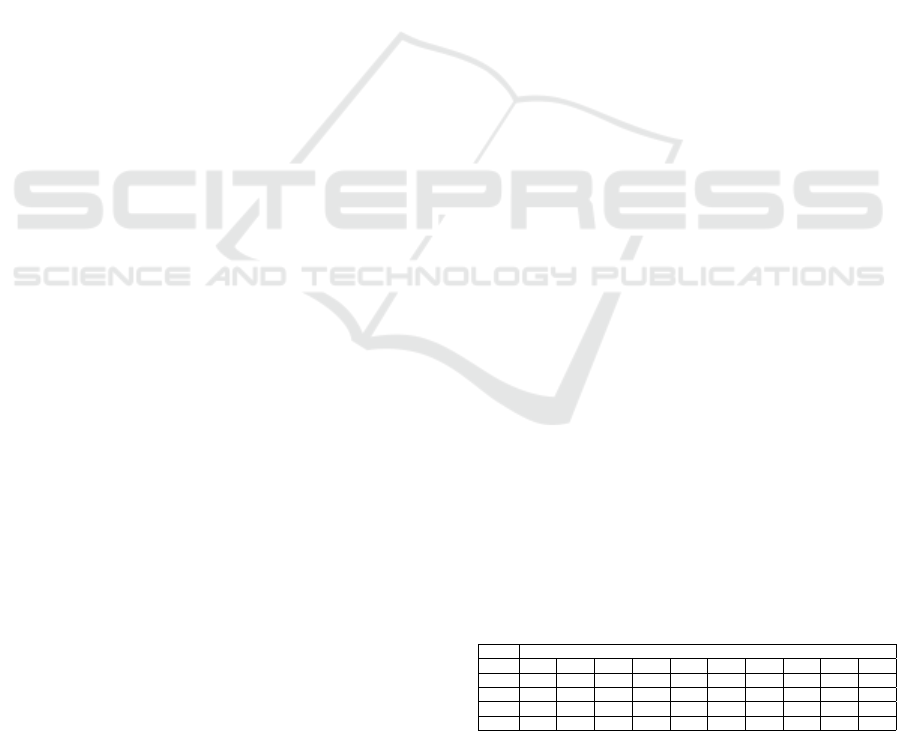
”household grouping heuristic” that allows household
members to seats near to each other without respect-
ing social distancing, and show that the proposed ap-
proach increases the capacity of the transit vehicles
(e.g., airplanes, school buses, and trains) without in-
creasing the risk of infection. The ongoing pandemic
of COVID-19 and the constant change of rules to ar-
rest the spread of the virus have been a high impact
on transportation sector. Providing flexible models
to manage the capacity during these sudden events is
crucial for avoiding the service disruption. The ma-
jority of the reviewed works, focused on social dis-
tancing, hence on the reduction of the capacity of the
vehicles. However, only one work, i.e., (Moore et al.,
2021), considers the possibility to allocate household
groups on adjacent seats. In our work we focus on
this particular strategy, currently adopted in bus pas-
senger transportation. For this type of transport, in
fact, the current Italian rule establishes that the use of
adjacent seats is limited exclusively to cohabiting pas-
sengers in the same housing unit, as well as between
relatives and people who have stable interpersonal re-
lationships. It is clear that this possibility could lead
to a potential increase in the capacity of the vehicle
(i.e., number of allocable seats), and consequently an
increase in the number of sold tickets, hence to obtain
additional revenues. Our work present some similar-
ities with (Moore et al., 2021), in fact, they consider
the allocation of groups of people on a bus. How-
ever, the main difference is that (Moore et al., 2021)
consider a single-destination transportation service,
in our work, instead, we consider more lines and a
multi origin-destination scenario. In addition, (Moore
et al., 2021) propose a heuristic approach to allocate
the groups, while in our work we propose a mathe-
matical model to handle the problem.
3 A LINEAR PROGRAMMING
MODEL FOR GROUPS
ASSIGNMENT PROBLEM
UNDER COVID-19
RESTRICTIONS
We propose a mathematical model for managing dif-
ferent sized kin groups of passengers on the buses, re-
specting the social distancing limitations imposed by
the Italian government rule reported in the previous
section. The proposed model is an extension of the
BSAP (Bus Seat Assignment Problem) formulation
defined by (Guerriero et al., 2020). As in (Guerriero
et al., 2020), we consider a passenger transportation
company that offers a transportation service from a
given set of origins to a given set of destinations. The
company sells a set of products to several groups of
customers on a given time horizon. Each product is
an origin-destination (OD) transportation service per-
formed by a bus. At each time of the planning hori-
zon, the company has to decide how to manage the
overall capacity in the most profitable way.
Let E = {e
1
, . . . , e
n
} denote the set of n origins
and F = { f
1
, . . . , f
q
} the set of q destinations. A
generic product is denoted as the pair {(e, f ) : f >
e, e ∈ E, f ∈ F} and represents the OD transportation
service from the bus station e to the bus station f .
All the products are sorted in increasing order of the
origin bus station and stored in the set (EF), that is
(EF) = (e
1
, f
1
), . . . , (e
1
, f
q
), (e
2
, f
1
), . . . , (e
n
, f
q
). The
products offered by the company can be indexed as
p = (1, . . . , |(EF)|). We assume that the company per-
forms the transportation service using a set of buses,
each of them characterized by a given number of rows
i = 1, . . . m, each row is composed by a certain num-
ber of seats denoted by l. In addition, we also assume
that every bus has the same number of seats on each
row. The number of seats per each row multiplied by
the number of rows of the bus represents the seating
capacity of the bus. The buses have t lines, t = 1, . . . T
and C
t
is the capacity of line t that is the seating ca-
pacity of the bus, which runs on the line t. Each line t
consists of a given number of stops denoted as S
t
+ 1
including the starting and the terminal bus stations
and S
t
legs between each two bus stations. All the
products (i.e., OD transportation service) produced by
each line t, t = 1, . . . , T are stored in a sequence ac-
cording to the incremental order of f and e, that is
(EF)
t
= (1, 2)
t
, . . . , (1, S
t
+ 1)
t
, (2, 3), . . . , (S
t
, S
t
+ 1).
After numbering all the products in (EF)
t
from left to
right, we can get the product sequence indexed by the
serial number p
t
= (1, . . . , |(EF)
t
|). Let H
t
= h
t
sp
t
, s =
1, . . . , S
t
, p
t
= 1 . . . , |(EF)
t
| denote a binary matrix,
each element being equal to 1 if product p
t
generated
by the line t uses leg s and zero otherwise. Each col-
umn of matrix H
t
contains all the information related
to the legs involved in the OD transportation services
provided by the line t. An example of matrix H
t
for a
line with S
t
= 5 stops and 10 products is reported in
Figure 2.
Product
Leg (1,2) (1,3) (1,4) (1,5) (2,3) (2,4) (2,5) (3,4) (3,5) (4,5)
(1, 2) 1 1 1 1 0 0 0 0 0 0
(2, 3) 0 1 1 1 1 1 1 0 0 0
(3, 4) 0 0 1 1 0 1 1 1 1 0
(4, 5) 0 0 0 1 0 0 1 0 1 1
Figure 2: Representation of of the matrix B, for a line with
5 stops and 10 products.
We assume that different lines can deliver the
same OD transportation service from e to f , that is
ICORES 2022 - 11th International Conference on Operations Research and Enterprise Systems
70