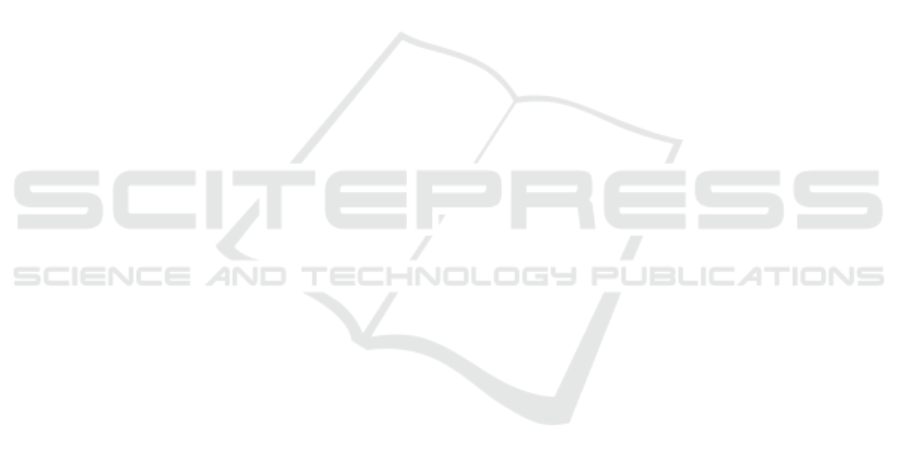
IEEE Journal of Biomedical and Health Informatics,
24(1):160–170.
Laimer, J., Bruckmoser, E., Helten, T., Kofler, B., Zel-
ger, B., Brunner, A., Zelger, B., Huck, C. W., Tap-
pert, M., Rogge, D., Schirmer, M., and Pallua, J. D.
(2021). Hyperspectral imaging as a diagnostic tool to
differentiate between amalgam tattoos and other dark
pigmented intraoral lesions. Journal of Biophotonics,
14(2).
Leavesley, S. J., Walters, M., Lopez, C., Baker, T., Favreau,
P. F., Rich, T. C., Rider, P. F., and Boudreaux, C. W.
(2016). Hyperspectral imaging fluorescence excita-
tion scanning for colon cancer detection. Journal of
Biomedical Optics, 21(10):104003.
Li, H., Liu, W., Dong, B., Kaluzny, J. V., Fawzi, A. A., and
Zhang, H. F. (2017). Snapshot hyperspectral retinal
imaging using compact spectral resolving detector ar-
ray. Journal of Biophotonics, 10(6-7):830–839.
Lin, J., Clancy, N. T., Sun, X., Qi, J., Janatka, M., Stoy-
anov, D., and Elson, D. S. (2016). Probe-Based
Rapid Hybrid Hyperspectral and Tissue Surface Imag-
ing Aided by Fully Convolutional Networks. In
Ourselin, S., Joskowicz, L., Sabuncu, M. R., Unal,
G., and Wells, W., editors, Medical Image Computing
and Computer-Assisted Intervention - MICCAI 2016,
volume 9902, pages 414–422. Springer International
Publishing, Cham. Series Title: Lecture Notes in
Computer Science.
Liu, K., Lin, S., Zhu, S., Chen, Y., Yin, H., Li, Z., and Chen,
Z. (2021). Hyperspectral microscopy combined with
DAPI staining for the identification of hepatic carci-
noma cells. Biomedical Optics Express, 12(1):173.
Liu, N., Chen, Z., and Xing, D. (2020). Integrated photoa-
coustic and hyperspectral dual-modality microscopy
for co-imaging of melanoma and cutaneous squamous
cell carcinoma in vivo. Journal of Biophotonics,
13(8).
Lv, M., Li, W., Tao, R., and Lovell, N. H. (2021). Spatial-
Spectral Density Peaks-Based Discriminant Analysis
for Membranous Nephropathy Classification Using
Microscopic Hyperspectral Images. IEEE Journal of
Biomedical and Health Informatics, pages 1–1.
Mahbub, S. B., Guller, A., Campbell, J. M., Anwer, A. G.,
Gosnell, M. E., Vesey, G., and Goldys, E. M. (2019).
Non-Invasive Monitoring of Functional State of Ar-
ticular Cartilage Tissue with Label-Free Unsupervised
Hyperspectral Imaging. Scientific Reports, 9(1):4398.
Michael, R., Lenferink, A., Vrensen, G. F. J. M., Gelpi, E.,
Barraquer, R. I., and Otto, C. (2017). Hyperspectral
Raman imaging of neuritic plaques and neurofibril-
lary tangles in brain tissue from Alzheimer’s disease
patients. Scientific Reports, 7(1):15603.
Misra, S. K., Ostadhossein, F., Daza, E., Johnson, E. V.,
and Pan, D. (2016). Hyperspectral Imaging Offers Vi-
sual and Quantitative Evidence of Drug Release from
Zwitterionic-Phospholipid-Nanocarbon When Con-
currently Tracked in 3D Intracellular Space. Advanced
Functional Materials, 26(44):8031–8041.
Nahmad-Rohen, A., Regan, D., Masia, F., McPhee, C.,
Pope, I., Langbein, W., and Borri, P. (2020). Quanti-
tative Label-Free Imaging of Lipid Domains in Single
Bilayers by Hyperspectral Coherent Raman Scatter-
ing. Analytical Chemistry, 92(21):14657–14666.
Nystr
¨
om, S., B
¨
ack, M., Nilsson, K. P. R., and Ham-
marstr
¨
om, P. (2017). Imaging Amyloid Tissues
Stained with Luminescent Conjugated Oligothio-
phenes by Hyperspectral Confocal Microscopy and
Fluorescence Lifetime Imaging. Journal of Visualized
Experiments, (128):56279.
Ogi, H., Moriwaki, S., Kokubo, M., Hikida, Y., and Itoh, K.
(2019). Label-free classification of neurons and glia in
neural stem cell cultures using a hyperspectral imag-
ing microscopy combined with machine learning. Sci-
entific Reports, 9(1):633.
Ortega, S., Guerra, R., Diaz, M., Fabelo, H., Lopez, S., Cal-
lico, G. M., and Sarmiento, R. (2019). Hyperspec-
tral Push-Broom Microscope Development and Char-
acterization. IEEE Access, 7:122473–122491.
Palombo, F., Masia, F., Mattana, S., Tamagnini, F., Borri, P.,
Langbein, W., and Fioretto, D. (2018). Hyperspectral
analysis applied to micro-Brillouin maps of amyloid-
beta plaques in Alzheimer’s disease brains. The Ana-
lyst, 143(24):6095–6102.
Paugh, J. R., Alfonso-Garcia, A., Nguyen, A. L., Suhalim,
J. L., Farid, M., Garg, S., Tao, J., Brown, D. J., Potma,
E. O., and Jester, J. V. (2019). Characterization of
expressed human meibum using hyperspectral stimu-
lated Raman scattering microscopy. The Ocular Sur-
face, 17(1):151–159.
Pichette, J., Laurence, A., Angulo, L., Lesage, F.,
Bouthillier, A., Nguyen, D. K., and Leblond, F.
(2016). Intraoperative video-rate hemodynamic re-
sponse assessment in human cortex using snapshot hy-
perspectral optical imaging. Neurophotonics, 3(04):1.
Rebner, K., Ostertag, E., and Kessler, R. W. (2016). Hyper-
spectral backscatter imaging: a label-free approach to
cytogenetics. Analytical and Bioanalytical Chemistry,
408(21):5701–5709.
Schultz, R. A., Nielsen, T., Zavaleta, J. R., Ruch, R., Wyatt,
R., and Garner, H. R. (2001). Hyperspectral imaging:
A novel approach for microscopic analysis. Cytome-
try, 43(4):239–247.
Semwogerere, D. and Weeks, E. R. (2005). G. Wnek,
G. Bowlin (Eds.), Encyclopedia of Biomaterials and
Biomedical Engineerin. Taylor and Francis, New
York.
Sen, D., SoRelle, E. D., Liba, O., Dalal, R., Paulus, Y. M.,
Kim, T.-W., Moshfeghi, D. M., and de la Zerda,
A. (2016). High-resolution contrast-enhanced opti-
cal coherence tomography in mice retinae. Journal
of Biomedical Optics, 21(06):1.
Seo, Y., Park, B., Hinton, A., Yoon, S.-C., and Lawrence,
K. C. (2016). Identification of Staphylococcus species
with hyperspectral microscope imaging and classifi-
cation algorithms. Journal of Food Measurement and
Characterization, 10(2):253–263.
Septiana, L., Suzuki, H., Ishikawa, M., Obi, T., Kobayashi,
N., Ohyama, N., Ichimura, T., Sasaki, A., Wihardjo,
E., and Andiani, D. (2019). Elastic and collagen fibers
discriminant analysis using H&E stained hyperspec-
tral images. Optical Review, 26(4):369–379.
Song, J., Hu, M., Wang, J., Zhou, M., Sun, L., Qiu, S., Li,
Q., Sun, Z., and Wang, Y. (2019). ALK positive lung
SIGMAP 2021 - 18th International Conference on Signal Processing and Multimedia Applications
118