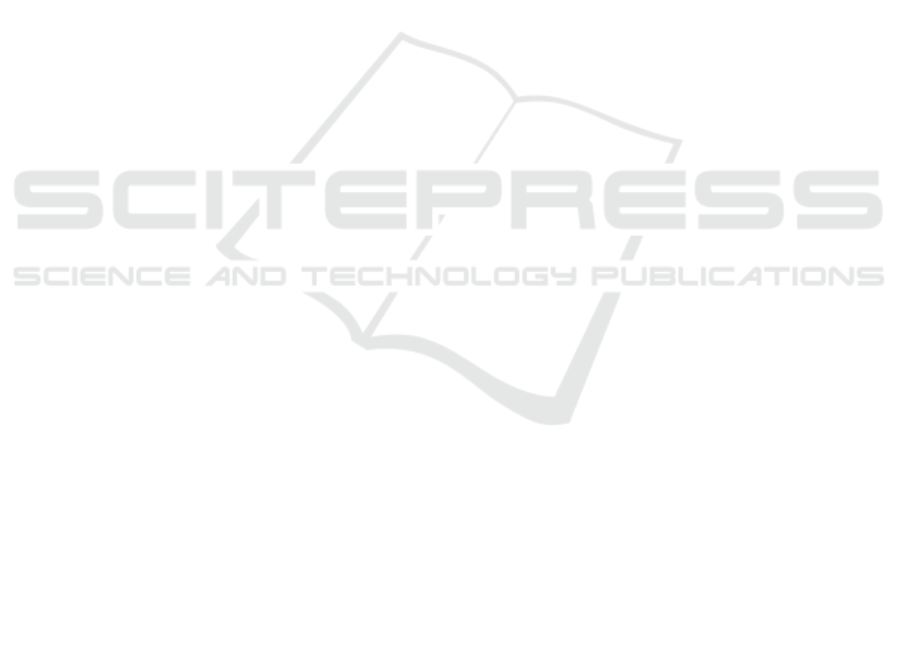
Bangladesh. Palgrave Communications, 7(2), 222–239.
https://doi.org/10.1057/s41599-019-0302-1
Manzi, L. (International O. for M. (2016). Migration from
rural areas to cities: challenges and opportunities |
Regional Office for Central America, North America
and the Caribbean. Retrieved March 8, 2021, from http
s://rosanjose.iom.int/SITE/en/blog/migration-rural-are
as-cities-challenges-and-opportunities
National Institute of Standards and Technology. (2018).
Framework for improving critical infrastructure
cybersecurity. Proceedings of the Annual ISA Analysis
Division Symposium, 535, 9–25.
Open Geospatial Consortium. (2015). Open Geospatial
Consortium OGC Smart Cities Spatial Information
Framework. OGC Doc. No. 14-115, 1–32. Retrieved
from https://portal.opengeospatial.org/files/61188
Paskaleva, K. & Evans, J. & Martin, C. & Linjordet, T. &
Yang, D & Karvonen, A. (2017). Data Governance in
the Sustainable Smart City. Informatics. 4. 41.
10.3390/informatics4040041.
Pérez-Delhoyo, R., García-Mayor, C., Mora-Mora, H.,
Gilart-Iglesias, V., & Andújar-Montoya, M. D. (2016).
Making smart and accessible cities: An urban model
based on the design of intelligent environments.
SMARTGREENS 2016 - Proceedings of the 5th
International Conf. on Smart Cities and Green ICT
Systems, (Smartgreens), 63–70. https://doi.org/1
0.5220/0005798100630070
Plale, B., & Kouper, I. (2017). The Centrality of Data: Data
Lifecycle and Data Pipelines. In Data Analytics for
Intelligent Transportation Systems (pp. 91–111).
https://doi.org/10.1016/B978-0-12-809715-1.00004-3
Pouchard, L. (2015). Revisiting the Data Lifecycle with Big
Data Curation. International Journal of Digital Curation,
10(2), 176–192. https://doi.org/10.2218/ijdc.v10i2.342
Rabelo, A. C. S., Oliveira, I. L., & Lisboa-Filho, J. (2017).
An architectural model for intelligent cities using
collaborative spatial data infrastructures.
SMARTGREENS 2017 - Proceedings of the 6th
International Conf. on Smart Cities and Green ICT
Systems, (Smartgreens), 242–249. https://doi.org/10.
5220/0006306102420249
Siddiqa, A., Hashem, I. A. T., Yaqoob, I., Marjani, M.,
Shamshirband, S., Gani, A., & Nasaruddin, F. (2016).
A survey of big data management: Taxonomy and state-
of-the-art. Journal of Network and Computer
Applications, 71, 151–166. https://doi.org/10.1016/
j.jnca.2016.04.008
Sinaeepourfard, A., Masip-Bruin, X., Garcia, J. and Marín-
Tordera, E., (2015). A Survey on Data Lifecycle
Models: Discussions toward the 6Vs Challenges.
Technical Report (UPC-DAC-RR-2015–18).
Sinaeepourfard, A., Garcia, J., Masip-Bruin, X., & Marín-
Tordera, E. (2016). A comprehensive scenario agnostic
Data LifeCycle model for an efficient data complexity
management. Proceedings of the 2016 IEEE 12th
International Conf. on E-Science, e-Science 2016, 276–
281. https://doi.org/10.1109/eScience.2016.7870909
SmartCities. (2011). Creating Municipal ICT
Architectures. A Reference Guide from Smart Cities.
Retrieved from www.smartcities.info/ict-architecture
Urbaczewski, L., & Mrdalj, S. (2006). A Comparison of
Enterprise Architecture Frameworks. Issues in
Information Systems, 7(2), 18–23.
Webster, J., & Watson, R. T. (2002). Analyzing the past to
prepare for the future: writing a literature review. MIS
Quarterly, 26(2).
APPENDIX A
Ali, I. M. et al. (2019) Measuring the Performance of Big
Data Analytics Process, 97(14), pp. 3796–3808.
Alshammari, M & Simpson, A. (2018). Personal Data
Management: An Abstract Personal Data Lifecycle
Model. 10.1007/978-3-319-74030-0_55.
Attard J., Orlandi F., Auer S. (2016) Data Driven
Governments: Creating Value Through Open
Government Data. In: Hameurlain A. et al. (eds)
Transactions on Large-Scale Data- and Knowledge-
Centered Systems XXVII. Lecture Notes in Computer
Science, vol 9860. Springer, Berlin, Heidelberg
Ball, A., (2012) Review of Data Management Lifecycle
Models. University of Bath, Bath, UK.
Barnaghi, P. & Bermúdez-Edo, M. & Tönjes, R. (2015).
Challenges for Quality of Data in Smart Cities. Journal
of Data and Information Quality. 6. 1-4.
10.1145/2747881.
Carlson, J., (2014) The Use of Life Cycle Models in
Developing and Supporting Data Services. In J. M. Ray
(Ed.), Research Data Management. Practical
Strategies for Information Professionals. West
Lafayette: Purdue University Press., 63-86.
Coleman, S.L, (2013). Measuring Data Quality for Ongoing
Improvement. A datta quality assessment framework.
In MK Series on Business Intelligence, Morgan
Kaufmann.
Cox, A. M., & Tam, W. W. T. , (2018) A critical analysis
of lifecycle models of the research process and research
data management. Aslib Journal of Information
Management, 70(2), 142–157. https://doi.org/
10.1108/AJIM-11-2017-0251.
DAMA International, (2017). The DAMA Guide to the
Data Management Body of Knowledge – DAMA-
DMBOK. Technics Publications.
Elmekki, H., Chiadmi, D. and Lamharhar, H., (2019) Open
Government Data: Towards a comparison of Data
Lifecycle models. In Proceedings of the ArabWIC 6th
Annual International Conference Research Track
(ArabWIC 2019). ACM, New York, NY, USA, Article
15, 6 pages. DOI: https://doi.org/10.1145/33331
65.3333180.
El Arass, M. Tikito, I. and Souissi, N. (2017). Data
lifecycles analysis: Towards intelligent cycle,
Intelligent Systems and Computer Vision (ISCV), Fez,
pp. 1-8.
SMARTGREENS 2021 - 10th International Conference on Smart Cities and Green ICT Systems
218