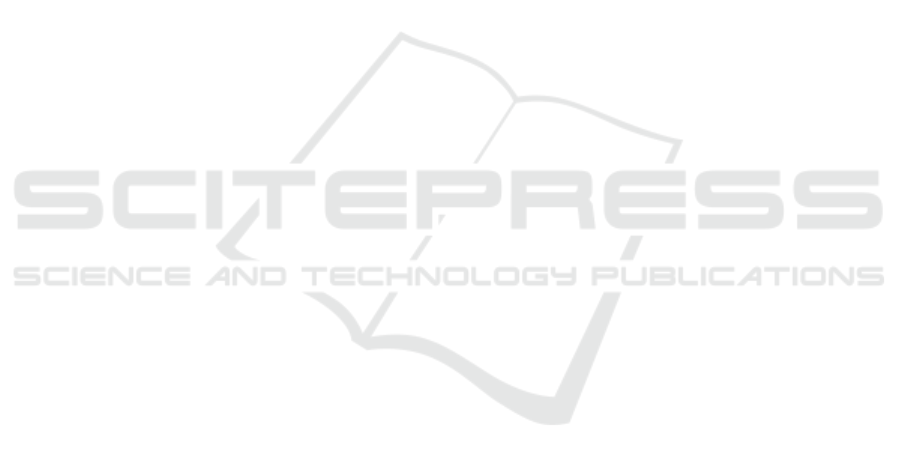
Haiyan, W., Haomin, Y., and Xueming, Li abd Haijun, R.
(2015). Semi-supervised autoencoder: A joint ap-
proach of representation and classification. In Interna-
tional Conference on Computational Intelligence and
Communication Networks, pages 1424–1430.
Hinton, G. E. and Salakhutdinov, R. (2006). Reducing the
dimensionality of data with neural networks. Science,
313(5786):504–507.
Hinton, G. E. and Zemel, R. S. (1993). Autoencoders, min-
imum description length and helmholtz free energy.
In Proceedings of the 6th International Conference on
Neural Information Processing Systems, pages 3–10.
K
¨
achele, M., Amirian, M., Thiam, P., Werner, P., Walter, S.,
Palm, G., and Schwenker, F. (2016a). Adaptive confi-
dence learning for the personalization of pain intensity
estimation systems. Evolving Systems, 8(1):1–13.
K
¨
achele, M., Thiam, P., Amirian, M., Schwenker, F.,
and Palm, G. (2016b). Methods for person-
centered continuous pain intensity assessment from
bio-physiological channels. IEEE Journal of Selected
Topics in Signal Processing, 10(5):854–864.
Kessler, V., Thiam, P., Amirian, M., and Schwenker, F.
(2017). Pain recognition with camera photoplethys-
mography. In Seventh International Conference on
Image Processing Theory, Tools and Applications
(IPTA), pages 1–5.
Khattar, D., Goud, J. S., Gupta, M., and Varma, V. (2019).
MVAE: Multimodal Variational Autoencoder for fake
news detection. In The World Wide Web Conference,
pages 2915–2921.
Kingma, D. P. and Ba, J. (2015). Adam: A method for
stochastic optimization. In Proceedings of the 3rd In-
ternational Conference on Learning Representations
(ICLR).
Le, L., Patterson, A., and White, M. (2018). Supervised
autoencoders: Improving generalization performance
with unsupervised regularizers. In Advances in Neural
Information Processing Systems, number 31, pages
107–117. Curran Associates, Inc.
Lim, H., Kim, B., Noh, G.-J., and Yoo, S. K. (2019). A
deep neural network-based pain classifier using a pho-
toplethysmography signal. Sensors, 2(384).
Liu, X., Wang, M., Zha, Z.-J., and Hong, R. (2019a). Cross-
modality feature learning via convolutional autoen-
coder. ACM Transactions on Multimedia Computing,
Communications, and Applications, 15(1s):7:1–7:20.
Liu, X., Zhou, Q., Zhao, J., Shen, H., and Xiong, X.
(2019b). Fault diagnosis of rotating machinery un-
der noisy environment conditions based on a 1-d con-
volutional autoencoder and 1-d convolutional neural
network. Sensors, 19(972).
Lopes, N. and Ribeiro, B. (2015). Machine Learning
for Adaptive Many-Core Machines - A Practical Ap-
proach, chapter Deep Belief Networks (DBNs), pages
155–186. Springer International Publishing.
Lopez-Martinez, D. and Picard, R. (2018). Continuous pain
intensity estimation from autonomic signals with re-
current neural networks. In 40th Annual International
Conference of the IEEE Engineering in Medecine and
Biology Society (EMBC), pages 5624–5627.
Pedregosa, F., Varoquaux, G., Gramfort, A., Michel, V.,
Thirion, B., Grisel, O., Blondel, M., Prettenhofer,
P., Weiss, R., Dubourg, V., Vanderplas, J., Passos,
A., Cournapeau, D., Brucher, M., Perrot, M., and
Duchesnay, E. (2011). Scikit-learn: Machine learning
in Python. Journal of Machine Learning Research,
12:2825–2830.
Squires, N. K., Squires, K. C., and Hillyard, S. A. (1975).
Two varieties of long-latency positive waves evoked
by unpredictable auditory stimuli in man. Elec-
troencephalography and Clinical Neurophysiology,
38(4):387–401.
Thiam, P., Kessler, V., Amirian, M., Bellmann, P., Layher,
G., Zhang, Y., Velana, M., Gruss, S., Walter, S., Traue,
H. C., Kim, J., Schork, D., Andr
´
e, E., Neumann, H.,
and Schwenker, F. (2019). Multi-modal pain inten-
sity recognition based on the senseemotion database.
IEEE Transactions on Affective Computing, pages 1–
1.
Thiam, P., Meudt, S., Palm, G., and Schwenker, F. (2018). A
temporal dependency based multi-modal active learn-
ing approach for audiovisual event detection. Neural
Processing Letters, 48(2):709–732.
Thiam, P. and Schwenker, F. (2017). Multi-modal data fu-
sion for pain intensity assessement and classification.
In 2017 Seventh International Conference on Image
Processing Theory, Tools and Applications (IPTA),
pages 1–6.
Vukoti
´
c, V., Raymond, C., and Gravier, G. (2016). Bidi-
rectional joint representation learning with symmetri-
cal deep neural networks for multimodal and cross-
modal applications. In Proceedings of the 2016 ACM
on International Conference on Multimedia Retrieval,
pages 343–346.
Walter, S., Gruss, S., Ehleiter, H., Tan, J., Traue, H. C.,
Crawcour, S., Werner, P., Al-Hamadi, A., and An-
drade, A. (2013). The BioVid heat pain database data
for the advancement and systematic validation of an
automated pain recognition system. In IEEE Interna-
tional Conference on Cybernetics, pages 128–131.
Werner, P., Al-Hamadi, A., Niese, R., Walter, S., Gruss, S.,
and Traue, H. C. (2014). Automatic pain recognition
from video and biomedical signals. In Proceedings of
the International Conference on Pattern Recognition
(ICPR), pages 4582–4587.
Yang, X., Ramesh, P., Chitta, R., Madhvanath, S., Bernal,
E. A., and Luo, J. (2017). Deep multimodal repre-
sentation learning from temporal data. In IEEE Con-
ference on Computer Vision and Pattern Recognition,
pages 5447–5455.
ICPRAM 2020 - 9th International Conference on Pattern Recognition Applications and Methods
296