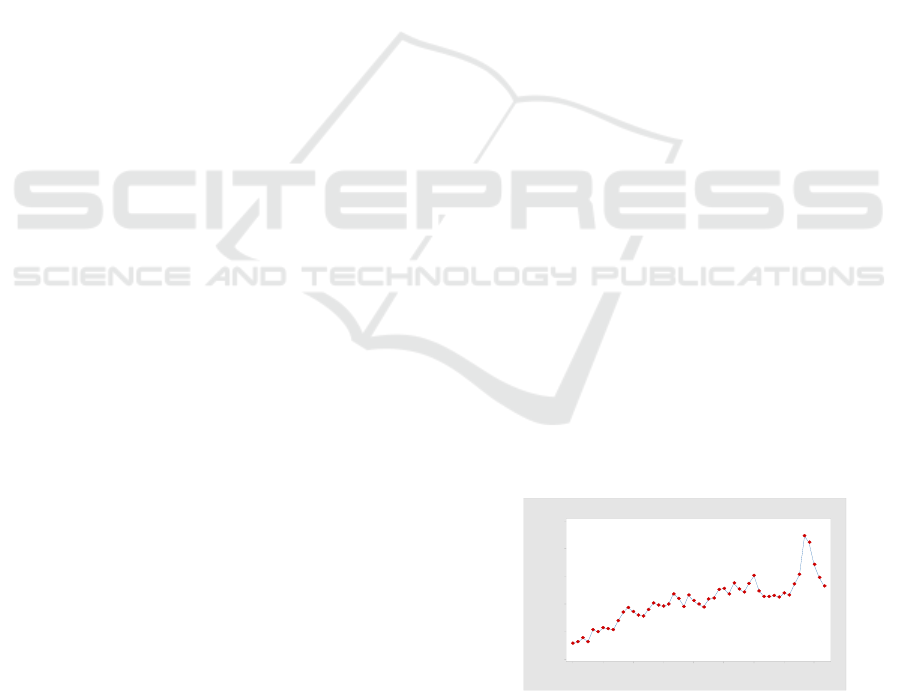
accurately and also as an indicator of decision support
systems in the health industry (Choudhury, 2019).
In line with Putri's research (2018), the
exponential smoothing model that shows the
possibility of hypertension cases shows a MAPE
value of 25.71% for men and 19.63% for women so
that it can be concluded that this forecasting model
can help the decision making process for a long
period of time will come based on forecast data that
has similarities with actual data (Putri, Herawati and
Ramani, 2018).
Based on Choudhury's research (2019), the
density in the ED is triggered by various factors
including the patient population, physical capacity,
practical capacity, functional capacity, and fiscal
capacity. Density in the emergency room can
potentially hinder patient care which causes treatment
delay and the possibility of errors in the medical
treatment process (Choudhury, 2019).
RSUD Kembangan is a hospital that has
emergency services. The situation in the Emergency
Department is generally overcrowded where many
patients come and are unpredictable. The overcrowded
emergency room also results in reduced productivity
and mobility of services for doctors and nurses so as to
reduce the quality of excellent health services.
Based on the results of preliminary observational
data in 2015-2019, patient visits in the emergency
room tend to increase with an increase of 42% per
year and the average number of patients who come
per day in the span of the year is 29 patients. With a
limited number of beds which are as many as 6 units,
this is considered less effective in conducting
emergency services. Moreover, the problem that
occurs is that patients in the ED are generally queued
like in a polyclinic and not infrequently they are
served in the patient's waiting chair. This condition if
left unchecked can trigger many errors in medical
services. Therefore, there is a need for modeling and
forecasting of patient visits in the emergency room so
that health care providers can have anticipation when
there is excessive density.
However, it is necessary to conduct research on
modeling and forecasting of patient visits in
emergency departments using the Autoregressive
Integrated Moving Average (ARIMA) and
Exponential Smoothing methods at RSUD
Kembangan.
2 METHOD
This research is a time-series study that uses the
Autoregressive Integrated Moving Average
(ARIMA) and Exponential Smoothing method to
predict patient visits in Emergency Departments
based on observations of data from April 2015 to June
2019 to forecast the number of IGD patient visits in
July 2019 to December 2020.
The population in this study is the data of the
number of patient visits Emergency Services at
RSUD Kembangan in 2015-2019 as many as 53,633
patient data with details of 3,992 patients in 2015,
10,939 patients in 2016, 13,482 patients in 2017,
14,842 patients in 2018 and 10,378 patients until June
2019. This research using total sampling.
Collected data derived from secondary data
namely emergency patient visit data by looking
directly at the annual report data and the daily report
book of the emergency department of RSUD
Kembangan in 2015-2019. The instrument used in
this study was a checklist sheet.
Data processing techniques in this study were
done manually and computerized. The data
processing stage is started from editing, income,
categorization, cleaning, and presentation data.
3 RESULT
3.1 Identify of ED Patient Visit Data
Pattern in RSUD Kembangan
Historical data on patient visits in the ED for the past
5 years starting from April 2015 to June 2019 can be
seen in figure 1.
Based on Figure 1, overall the actual data tends to
increase even though there are some values that show
a decrease. The lowest patient visit data occurred in
April 2015 with 289 patients while the highest patient
visit during the study period occurred in February
2019 with a total of 2227 patients due to cases of
Dengue Hemorrhagic Fever (DHF).
Figure 1: Total Patient Visits in RSUD Kembangan 2015-
2019.
Before forecasting, it is necessary to know the
decomposition of data patterns to determine whether
Year
Month
20192018201720162015
AprOktAprOktAprOktAprOktApr
2500
2000
1500
1000
500
0
JumlahKunjungan
Plot Jumlah Kunjungan Pasien IGD di RSUD Kembangan Tahun 2015-2019
Patient Visit Forecasting at Emergency Department using Autoregressive Integrated Moving Average (ARIMA) and Exponential Smoothing
Method in RSUD Kembangan
235