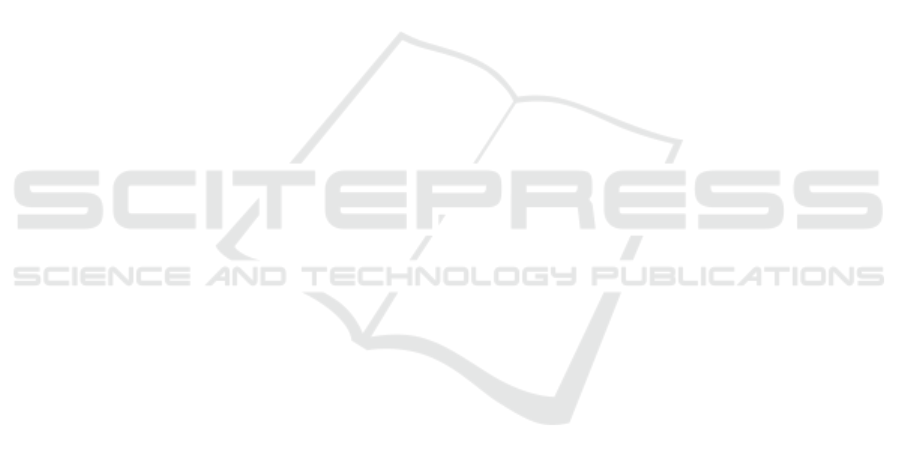
of e-portfolios. Computers in Human Behavior,
63:75–90.
Ajzen, I. (1991). The theory of planned behavior.
Organizational behavior and human decision
processes, 50(2):179–211.
Al-Gahtani, S. S. (2016). Empirical investigation of
e-learning acceptance and assimilation: A structural
equation model. Applied Computing and Informatics,
12(1):27–50.
Alhabeeb, A. and Rowley, J. (2018). E-learning
critical success factors: Comparing perspectives
from academic staff and students. Computers and
Education, 127(August):1–12.
Ali, M., Ali Raza, S., Qazi, W., and Puah, C.-H.
(2018). Assessing the e-learning system in
higher education institutes: Evidence from structural
equation modelling. Interactive Technology and
Smart Education.
Alsabawy, A. Y., Cater-Steel, A., and Soar, J. (2016).
Determinants of perceived usefulness of e-learning
systems. Computers in Human Behavior, 64:843–858.
Aparicio, M., Bacao, F., and Oliveira, T. (2016). Cultural
impacts on e-learning systems’ success. Internet and
Higher Education, 31:58–70.
Bagozzi, R. P. and Yi, Y. (1988). On the evaluation of
structural equation models. Journal of the academy
of marketing science, 16(1):74–94.
Baylari, A. and Montazer, G. A. (2009). Design a
personalized e-learning system based on item
response theory and artificial neural network
approach. Expert Systems with Applications,
36(4):8013–8021.
Bhattacherjee, A. (2001). An empirical analysis
of the antecedents of electronic commerce
service continuance. Decision support systems,
32(2):201–214.
Bhuasiri, W., Xaymoungkhoun, O., Zo, H., Rho, J. J.,
and Ciganek, A. P. (2012). Critical success factors
for e-learning in developing countries: A comparative
analysis between ICT experts and faculty. Computers
and Education, 58(2):843–855.
Cheng, Y. M. (2011). Antecedents and consequences of
e-learning acceptance. Information Systems Journal,
21(3):269–299.
Cheng, Y. M. (2014). Roles of interactivity and usage
experience in e-learning acceptance: A longitudinal
study. International Journal of Web Information
Systems, 10(1):2–23.
Cheung, R. and Vogel, D. (2013). Computers &
Education Predicting user acceptance of collaborative
technologies : An extension of the technology
acceptance model for e-learning. Computers &
Education, 63:160–175.
Chin, W. W. et al. (1998). The partial least squares approach
to structural equation modeling. Modern methods for
business research, 295(2):295–336.
Ching-Ter, C., Hajiyev, J., and Su, C. R. (2017). Examining
the students’ behavioral intention to use e-learning in
Azerbaijan? The General Extended Technology
Acceptance Model for E-learning approach.
Computers and Education, 111:128–143.
Cidral, W. A., Oliveira, T., Di Felice, M., and
Aparicio, M. (2018). E-learning success determinants:
Brazilian empirical study. Computers and Education,
122:273–290.
Clay, M. N., Rowland, S., and Packard, A. (2008).
Improving undergraduate online retention through
gated advisement and redundant communication.
Journal of College Student Retention: Research,
Theory and Practice, 10(1):93–102.
Davis, F. D. (1989). Perceived usefulness, perceived ease of
use, and user acceptance of information technology.
MIS quarterly, pages 319–340.
Fishbein, M. and Ajzen, I. (1977). Belief, attitude, intention,
and behavior: An introduction to theory and research.
Reading, MA: Addison-Wesley.
Fleming, J., Becker, K., and Newton, C. (2017). Factors
for successful e-learning: does age matter? Education
and Training, 59(1):76–89.
Fornell, C. and Larcker, D. (1981). Structural equation
models with unobservable variables and measurement
error Algebra and statistics. Journal of marketing
research. Advances Methods of Marketing Research,
18(3):382–388.
Garrison, D. R. (2011). E-learning in the 21st century: A
framework for research and practice. Routledge.
Goodhue, D. L. and Thompson, R. L. (1995).
Task-technology fit and individual performance.
MIS quarterly, pages 213–236.
Hair, J. F., Ringle, C. M., and Sarstedt, M. (19).
2011. PLS-SEM: Indeed a Silver Bullet. Journal of
Marketing Theory and Practice 19, 2:2011.
Hair, J. F., Sarstedt, M., Hopkins, L., and Kuppelwieser,
V. G. (2014). Partial least squares structural equation
modeling (PLS-SEM): An emerging tool in business
research. European Business Review, 26(2):106–121.
Hair Jr, J. F., Hult, G. T. M., Ringle, C., and Sarstedt, M.
(2017). A primer on partial least squares structural
equation modeling (PLS-SEM). Sage publications.
Henseler, J., Ringle, C. M., and Sinkovics, R. R. (2009).
The use of partial least squares path modeling in
international marketing. Advances in International
Marketing, 20(2009):277–319.
Hubalovsky, S., Hubalovska, M., and Musilek, M. (2019).
Assessment of the influence of adaptive E-learning
on learning effectiveness of primary school pupils.
Computers in Human Behavior, 92:691–705.
Hulland, J. (1999). Use of partial least squares (pls)
in strategic management research: A review of
four recent studies. Strategic management journal,
20(2):195–204.
Khairuddin, S. H., Ilona, D., and Zaitul (2018). Antecedents
of Intention to Use E-Learning. In MATEC Web of
Conferences.
Behavioural E-Learning Adoption among Higher Education Institution Students: A Possibility for Mentawaian Students Living in
Contemporary Culture
163