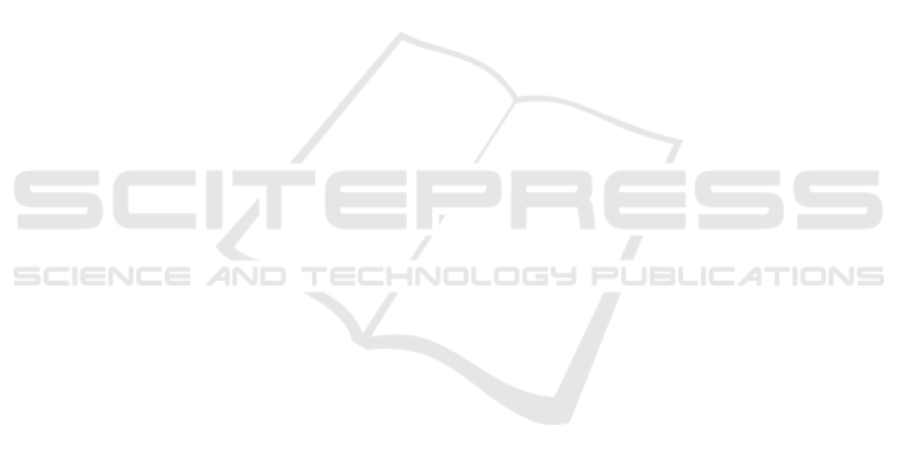
theoretic approach. Springer Science & Business
Media. Second edition. Springer: New York.
Calcagno, V., de Mazancourt, C. (2010). glmulti: an R
package for easy automated model selection with
(generalized) linear models. Journal of statistical
software, 34(12), pp. 1-29.
Chatman, D. G., Manville, M. (2014). Theory Versus
Implementation in Congestion-priced Parking: An
Evaluation of SFpark, 2011-2012. Research in
Transportation Economics, 44, pp. 52–60.
D’Aloia, M., Rizzi, M., Russo, R., Notarnicola, M. N.,
Pellicani, L. (2015). A marker-based image processing
method for detecting available parking slots from
UAVs, New Trends Image Analysis Processing. Cham,
Switzerland: Springer, pp. 275–281.
Dowling, C., Fiez, T., Ratliff, L., Zhang, B. (2017). How
Much Urban Traffic is Searching for Parking?
Simulating Curbside Parking as a Network of Finite
Capacity Queues. arXiv preprint arXiv:1702.06156.
Faheem, F., Mahmud, S.A., Khan, G.M., Rahman, M.,
Zafar, H. (2013) A Survey of Intelligent Car Parking
System, Journal of Applied Research and Technology,
11(5), pp. 714-726.
Fraifer, M., Fernström, M. (2016). Investigation of smart
parking systems and their technologies. 37
th
International Conference on Information Systems. IoT
Smart City Challenges Applications (ISCA 2016),
Dublin, Ireland, pp. 1-14.
Golias, K., Vlahogianni, E. I. (2018). Convolutional Neural
Networks for On-Street Parking Space Detection in
Urban Networks. IEEE Transactions on Intelligent
Transportation Systems.
Hampshire, R. C., Shoup, D. (2018). What share of traffic
is cruising for parking?. Journal of Transport
Economics and Policy (JTEP), 52(3), 184-201.
Ibeas, A., dell’Olio, L., Bordagaray, M., Ortúzar, J.D.
(2014). Modelling parking choices considering user
heterogeneity. Transportation Research Part A: Policy
and Practice, 70, pp. 41–49.
Lin, T., Rivano, H., Le Mouël, F., Baron, B., Spathis, P.,
Rivano, H., Voge, M. E. (2017). A survey of smart
parking solutions, IEEE Transactions on Intelligent
Transportation Systems, 18(12), pp. 3229-3253.
Margreiter, M., Mayer, P., Orfanou, F. (2015). A Concept
for Crowdsourcing of In-vehicle Data to Improve
Urban On-street Parking, mobil.TUM International
Scientific Conference on Mobility and Transport
“Technologies, Solutions and Perspectives for
Intelligent Transport Systems”, June 2015.
Margreiter, M., Orfanou, F., Mayer, P. (2017).
Determination of the parking place availability using
manual data collection enriched by crowdsourced in-
vehicle data, Transportation research procedia, 25, pp.
497-510.
Monteiro, F.V., Ioannou, P. (2018) On-Street Parking
Prediction Using Real-Time Data, 21
st
International
Conference on Intelligent Transportation Systems
(ITSC), November 4-7, Maui, Hawaii, USA.
Orfanou, F., Margreiter, M., Mayer, Ph., Alpas, M.,
Vlahogianni E. (2017). Driver’s Willingness to Use
Parking Assistance Tools and their Expectations: A
Case Study for the Cities of Munich and Athens, 8
th
International Congress on Transportation Research in
Greece “Transportation by 2030: Trends and
Perspectives”, Thessaloniki, Greece.
Revathi, G., Dhulipala, V. S. (2012). Smart parking systems
and sensors: A survey. Computing, Communication and
Applications (ICCCA), 2012 International Conference,
pp. 1-5, IEEE.
Shiftan, Y., Burd-Eden, R. Modeling Response to Parking
Policy (2001). Transportation Research Record:
Journal of the Transportation Research Board, 1765,
pp. 27–34.
Shoup, D. C. (2006). Cruising for Parking. Transport
Policy, 13(6), pp. 479–486.
Soto, J. J., Márquez, L., Macea, L. F. (2018). Accounting
for attitudes on parking choice: An integrated choice
and latent variable approach. Transportation Research
Part A: Policy and Practice, 111, pp. 65-77.
Van Ommeren, J., Wentink, D., Dekkers, J. (2011). The
Real Price of Parking Policy. Journal of Urban
Economics, 70(1), pp. 25–31.
Vlahogianni, E. I., Karlaftis, M. G., Golias, J. C. (2014).
Short-term traffic forecasting: Where we are and where
we’re going. Transportation Research Part C:
Emerging Technologies, 43, 3-19.
Vlahogianni, E. I., Kepaptsoglou, K., Tsetsos, V., Karlaftis,
M.G. (2015). A Real-Time Parking Prediction System
for Smart Cities, Journal of Intelligent Transportation
Systems, 2450(November), pp. 1–13.
Worldsensing (2019). [Online]. Available at:
http://www.fastprk.com/ [Accessed: 3 Jan. 2019]
Yang, W.X. (2004). An Improved Genetic Algorithm
Adopting Immigration Operator. Intelligent Data
Analysis, 8, pp. 385–401.
Smart Parking Assistance Services and User Acceptance: A European Model
497