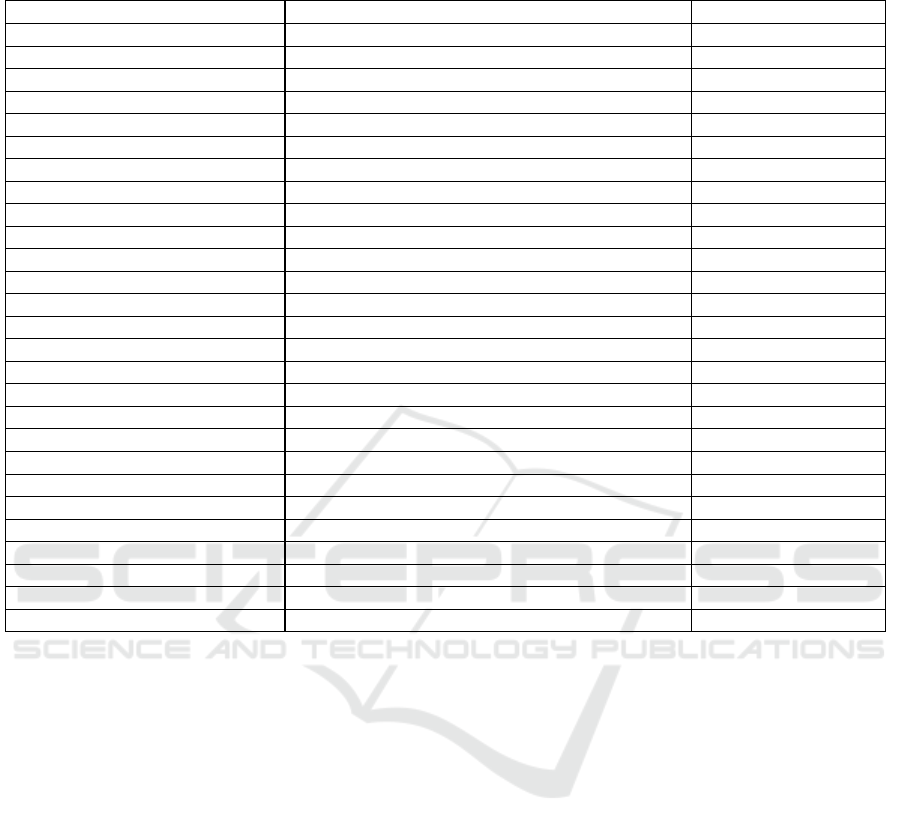
Table 4: Transparency Enhancing Tools (TETs), the technical requirements and GDPR Articles they help realising (* added
manually). Articles not addressed by TETs: 11, 12, 16, 18, 22, 25, 26, 32, 34.
TET Requirements GDPR Articles
Mozilla Lightbeam 211.5, 221.6 14, 15
P3P 111.2, 111.3, 111.16, 111.18, 111.19 5, 13, 14, 15, 19, 21
PrimeLife Policy Language 111.2, 111.3, 111.16, 111.18, 111.19 5, 13, 14, 15, 19, 21
Data Track 112.1, 221.5, 221.6, 221.7 5, 6, 7, 14, 15, 17, 30
Privacy Insight 112.1, 221.4, 221.5, 221.6, 221.7 5, 6, 7, 14, 15, 17, 30
Privacy Risk Analysis 111.9, 111.13
GDPR Privacy Dashboard 112.1, 211.5, 221.4, 221.6, 221.7 5, 6, 7, 14, 15, 17
Personal Data Table 112.1, 211.2, 211.3, 211.5, 221.4, 221.6, 221.7 5, 6, 7, 14, 15, 17
Disconnect me 211.5, 221.6 14, 15
Me and My Shadow 111.8, 111.13, 111.16, 111.19 5, 13, 14, 15
EuroPriSe 111.16, 221.1, 221.3, 221.4 5, 13, 14, 15
Privacy Score 111.6, 111.12, 111.13
Google Dashboard 112.1, 211.5, 221.6, 221.7 5, 6, 7, 14, 15, 17
Privacy Evidence 221.1, 221.4, 221.5, 222.1, 232.1 5, 30
TAMI Project 211.2, 211.3, 211.5, 221.1, 221.4, 222.1, 232.1 5, 14, 30
Privacy Through Transparency 211.2, 211.3, 221.1, 221.4, 221.5, 222.1, 232.1 5, 30
Private Verification of Access 211.2, 211.3, 221.1, 221.4, 222.1, 232.1 5, 30
Privacy Badger 211.5, 221.6 14, 15
Access My Info 112.1, 221.6 14, 15
TrustArc 111.16, 221.1, 221.3, 221.4 5, 13, 14, 15
openPDS 211.5, 221.6, 221.7 5, 6, 7, 14, 15, 17
Digi.me 221.6, 221.7 5, 6, 7, 14, 15, 17
Microsoft Dashboard 112.1, 211.5, 221.6, 221.7 5, 6, 7, 14, 15, 17
Privacy eSuite 221.1, 221.5, 221.7, 222.1, 232.1 5, 6, 7, 9*, 17, 30
Meeco 221.6, 221.7 5, 6, 7, 14, 15, 17
Blue Button 112.1, 221.6 14, 15
Usable Privacy 111.5, 111.10, 111.11, 111.15, 111.17, 111.19 13, 14, 15, 33
My Shadow (MMS), which reveals and highlights rel-
evant information in the policy of a few popular ser-
vices. We decided not to include those tools in our
study as the first only evaluates the quality of a policy,
without aiding data subjects understanding its con-
tents, and the second for only providing a few exam-
ples of policies. Nevertheless, it is possible to see the
matter is already subject of attention. We expect to
see a different scenario concerning tools for terms and
conditions in the future.
Another set of requirements which seem to have
gained less attention is regarding security breaches
and attacks. They constitute the majority of require-
ments not addressed by any TET: 111.7, 211.1, 211.4,
221.2, and 221.8. As security breaches are unforeseen
events, it does not come as a surprise that there are no
tools for aiding the understanding of issues related to
them. Nonetheless, it is important to notice that the
GDPR reserves two Articles to provisions on personal
data breaches (Art. 33 and 34), one of which is dedi-
cated to describing how to communicate such matters
to the affected data subjects. Being the health-care in-
dustry among the ones with most reported breaches,
and being medical data in the top three most com-
promised variety of data (for more details, see results
of the data breach investigation (Verizon, 2018)), we
consider this to be an area in need of further develop-
ment.
Legal Aspects. Only a few Articles from the GDPR
do not seem to be covered by any of our selected
transparency tools. We consider an Article as not cov-
ered when none of its paragraphs or sub-paragraphs
is correlated to at least one TET. Examples of this
are the Articles related to certification; Article 25 re-
gards data protection by design and by default, Article
32 has provisions on security of processing, but both
mention that compliance with such Articles may be
demonstrated through the use of approved certifica-
tion mechanisms referred to in Article 42.
Despite having included two certification seals in
our study (i.e., EuroPriSe, and TrustArc), we cannot
confirm they are approved certification mechanisms.
According to EuroPriSe, their criteria catalogue has
not been approved pursuant to Article 42(5) GDPR,
and they have not been accredited as a certification
ICISSP 2019 - 5th International Conference on Information Systems Security and Privacy
122