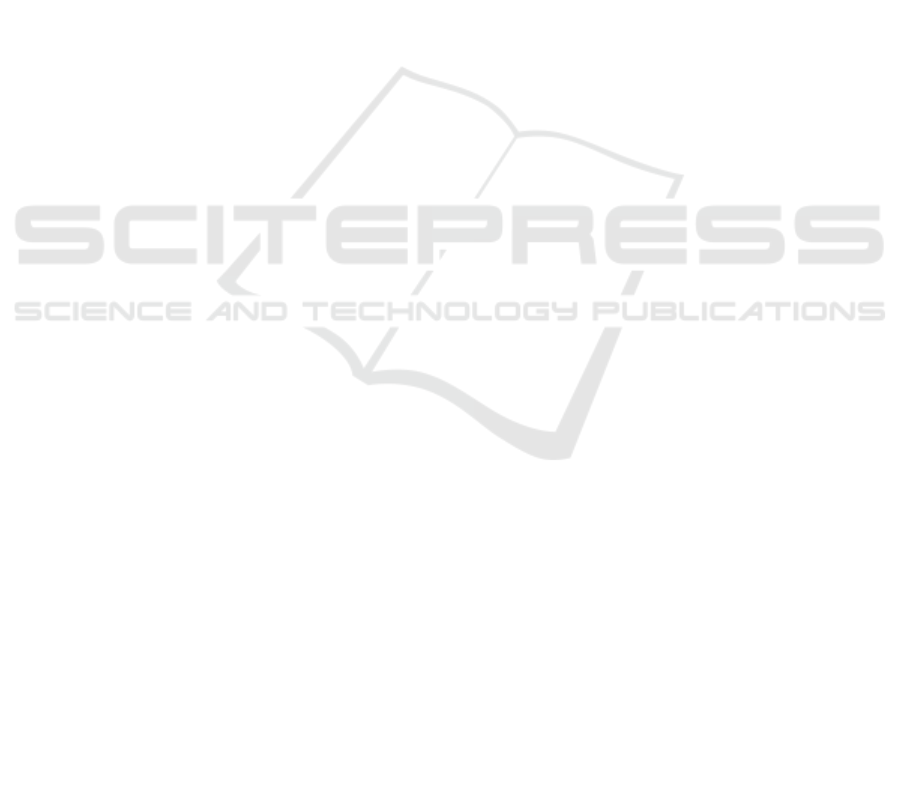
matter (PM10). Therefore, there was air pollution in
various regions in Riau Province and even in the areas
outside of Riau. In addition to causing illness, fog
smoke in Riau, especially in Pekanbaru causes
community activities disturbed, such as all education
activities in Riau, especially Pekanbaru City have
been stopped. One of the universities which halts its
academic activities, for 4 days, was the State Islamic
University of Sultan Syarif Kasim Riau. Moreover,
the visibility on the highway is only ± 200 meters,
thus causing rider activity is hampered. Air pollution
by particulate matter (PM10) has a dynamic
relationship with meteorological elements such as
rainfall, solar radiation, air temperature, humidity and
wind speed. In addition, the number of hotspots also
has a dynamic relationship with air pollution caused
by particulate matter (PM10) (Brown and Davis, 1973).
The guidance of Allah SWT about the duty of His
people to be grateful for the blessings that Allah
Almighty gives which is much explained in the
Qur'an, including the favor of the universe that Allah
has created for His people. Allah SWT asserted in
Qur'an that is for His people who are not grateful for
the blessings that Allah Almighty gives, then Allah
SWT will give a very painful penalty, which is
described in surah Ibrahim verse 7 is “And when your
Lord proclaimed: “If you give thanks, I will grant you
increase; but if you are ungrateful, My punishment is
severe”.
Several studies related to the study of air pollution
modeling and number of hotspots using vector
autoregressive (VAR) models have been conducted
by, such as a research conducted by Cai (2008) used
VAR analysis to predict the time series data of CO
pollution in California. Another research is Ahmad,
et al (2013) discusses the prediction of air pollution
by particulate matter (PM10) using the Box-Jenkins
method. Based on the explanation of air pollution, it
is necessary to predict the concentration of air
pollutant that is especially gas particulate matter
(PM10) and relating elements for the future by using
vector autoregressive model (VAR). Given the
importance of knowing the concentration of particle
matter (PM10) in Pekanbaru, this research tries to
provide a suitable statistical model for particulate
matter (PM10) data in Pekanbaru by using vector
autoregressive model (VAR). The purpose of this
research is to find the best model for particulate
matter density data (PM10) along with
meteorological elements in Pekanbaru city by using
vector autoregressive model (VAR). And determine
the prediction result of particulate matter
concentration (PM10) in the future by using vector
autoregressive (VAR) model in Pekanbaru city.
2 METHODS
2.1 Literature Review
Particulate matter (PM10) is particles which diameter
is less than 10 µm which can cause more hazardous
effect on human health, animal and plant than some
other larger particles formed of stationary source such
as vehicles (vehicle ekzos). Particulate Matter
(PM10) is largely produced from wild forest and land
burning. Rainfall is the height of rainwater collected
in a flat, non-volatile, non-pervasive and non-flowing
place (Chelani et al, 2004).
Solar radiation is energy radiance which comes
from thermonuclear process in the sun. Solar energy
is the energy source for all of existence. The air
temperature is a measure of the average kinetic
energy of molecule improvements or the temperature
condition of the air. The hotspot is the terminology of
a single pixel that has a higher temperature than the
surrounding area or location captured by a digital data
satellite sensor. Air humidity is the amount of water
vapor in the air (atmosphere) at a given time and
place. Wind is the air movement parallel to the
surface of the earth. Air moves from high pressure
areas to low pressure areas (Liew, 2002).
Prediction or forecasting is a forecasting process
for the future based on past data. Forecasting is a
fundamental thing in determining a plan or policy in
an agency this is due to the uncertainty of the values
of a variable in the future. Therefore, predictions are
very important in many fields because predictions of
future events must be incorporated into the process of
making a decision. The definition of the VAR model
is that all variables present in the VAR model are
endogenous. If there is a relationship associate
between variables observed, then the variables need
to be done the same way. So, there is no longer
endogenous and exogenous variables (Bowerman et
al, 2005). In general, the model VAR lag p for n
variables can be formulated as follows (Makridakis,
1998):
with
is a vector which size is
containing
variables entered in the VAR model at t time and
t – 1, i = 1,2,…, p,
is a vector of intercept which
size is n × 1,
is a coefficient matrix of sizes n × n
for each, , i = 1,2,…, p,
is a vector of sized n × 1
that is
,
is lag VAR, t is a period
of observation. The VAR model consisting of two
variables and 1 lag is the VAR(1) model:
ICMIs 2018 - International Conference on Mathematics and Islam
320