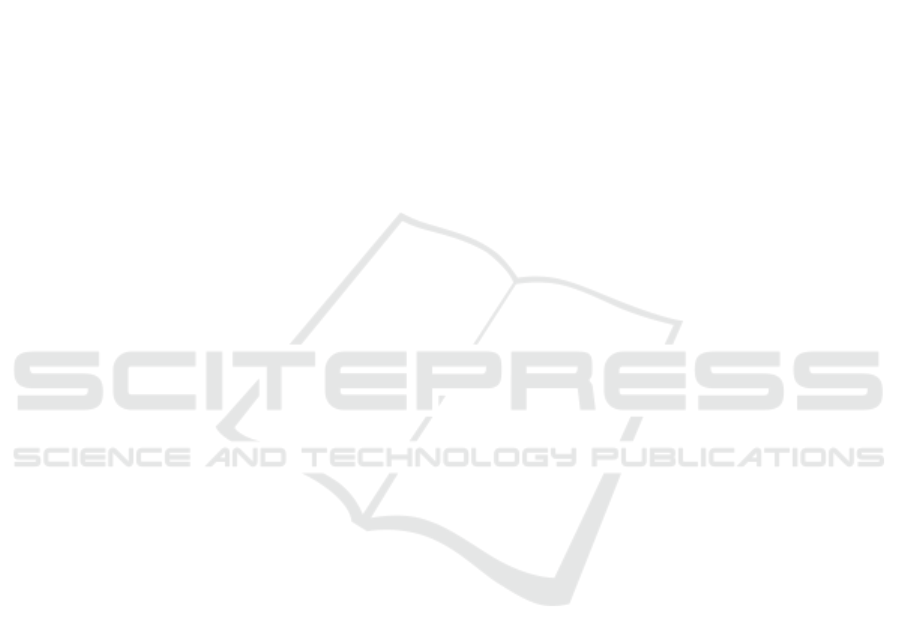
Modeling of Total Fertility Rate (TFR) in East Java Province using
Mixed Semiparametric Regression Spline Truncated and
Kernel Approach
Arip Ramadan
1
, I Nyoman Budiantara
1
and Ismaini Zain
2
1
Institut Teknologi Sepuluh Nopember, Jl. Raya ITS Sukolilo Surabaya, Jawa Timur, Indonesia
2
Department of Statistics, Faculty of Mathematics, Computing and Data Science,
Institut Teknologi Sepuluh Nopember, Surabaya, Indonesia
Keywords: Total Fertility Rate, Mix Estimator, Spline Truncated, Kernel, Semiparametric Regression and Knot Point.
Abstract: The problem of population growth in Indonesia year by year is relatively very high. Based on data from
Indonesia Demographic and Health Survey (SDKI), there is a very high increase of TFR and uncontrollable
increase of population in Indonesia. TFR in East Java is very high in 2012 reached 20%. The behavior of TFR
patterns is associated with the variables that are suspected to affect the Unmet Need, the Age Specific Fertility
Rates (ASFR), the Human Development Index (HDI) and the Infant Mortality Rate (IMR) has a very special
pattern. The relationship pattern between TFR and Unmet Need tends to be linear. While the pattern of
relationship between TFR with ASFR and HDI, in contrast to the Unmet Need that tends to be nonlinear
especially change as the increase or decrease value of these variables. The pattern of relationship between
TFR and IMR appears not to follow a certain pattern. Taking into account this description, this study modeled
using mixed semiparametric regression spline truncated and kernel spline models. The best model is a mixed
semiparametric regression spline truncated and kernel model with a combination of knots that has the smallest
GCV of 0.003964 and the coefficient of determination of 97.04%.
1 INTRODUCTION
Population is the most important thing in sustaining
the development of an area because it is both a subject
and an object of development. As the subject of
population development will play a role in achieving
the achievement of economic and social development
that can affect the increase in social welfare, while as
an object of population development is the party that
gets results from the development of a region.
The Total Fertility Rate (TFR) can affect the
development of the population in the future. In 2012,
there was a national TFR increase from 2.41 in 2008
to 2.6 in 2012. Based on the report, there were only
10 provinces that experienced a decline in their
fertility levels, while the rest were observed to
increase. TFR increases experienced by other
provinces ranged from 31 percent to 63 percent
(BKKBN, 2107).
East Java Province is a province that experienced
a very significant decline in TFR since the
introduction of family planning (KB) policies,
namely the TFR of East Java Province had reached
below 2.1 in 2002. However the 2012 East Java
Province TFR experienced a significant increase
since 2002 which had an effect on increasing the
return of TFR Indonesia. TFR East Java experienced
a TFR increase of more than 20 percent, from 2.1 in
2002 to 2.6 in 2012.
To see the shape of the relationship between TFR
and the variables that influence it can use the
regression method. But sometimes the form of
relationships that occur can vary, such as parametric
or nonparametric. Therefore obtaining a mixed
semiparametric spline truncated and kernel
regression model is important because it includes
parametric and nonparametric.
Ramadan, A., Budiantara, I. and Zain, I.
Modeling of Total Fertility Rate (TFR) in East Java Province using Mixed Semiparametric Regression Spline Truncated and Kernel Approach.
DOI: 10.5220/0008520602710277
In Proceedings of the International Conference on Mathematics and Islam (ICMIs 2018), pages 271-277
ISBN: 978-989-758-407-7
Copyright
c
2020 by SCITEPRESS – Science and Technology Publications, Lda. All r ights reserved
271