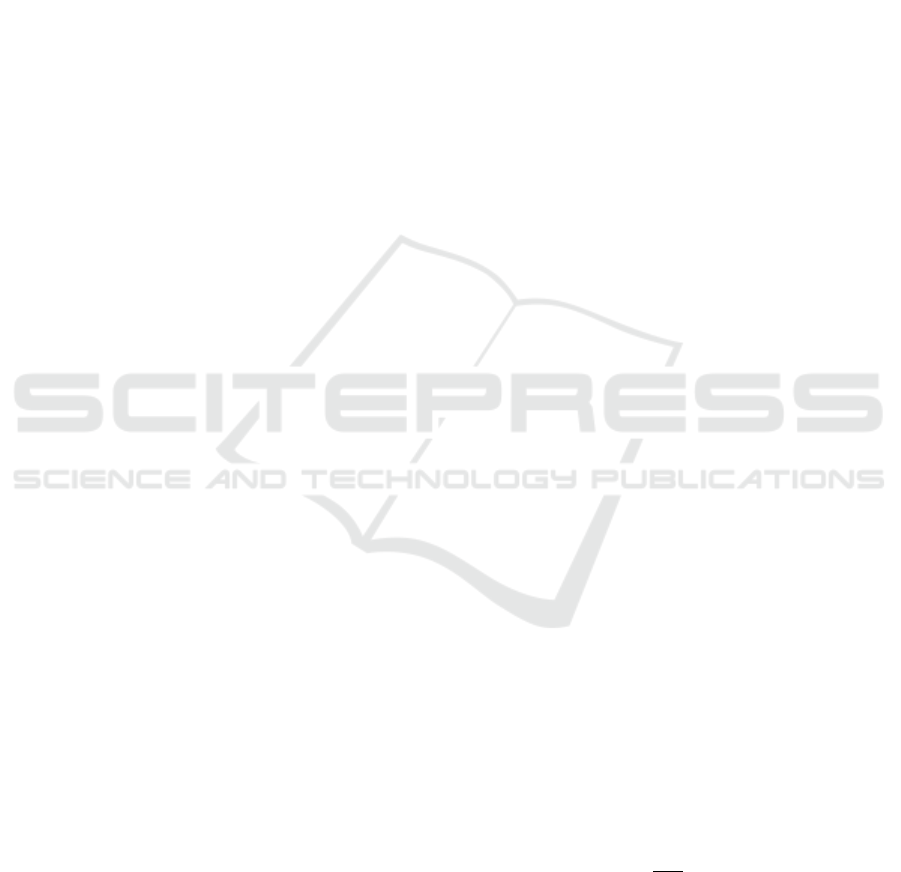
Detection of Financial Crisis in Indonesia based on Import and Yen
Exchange Rate to Rupiah Indicators using Combined of Volatility
and Markov Switching Models
Etik Zukhronah
1
, Sugiyanto
1
and Isna Ruwaidatul Azizah
2
1
Study Program of Statistics, Universitas Sebelas Maret Surakarta, Indonesia
2
Department of Mathematics, Universitas Sebelas Maret Surakarta, Indonesia
Keywords: Crisis, Detection, Import, Yen Exchange Rate to Rupiah, SWARCH.
Abstract: In 1997 and 1998 Indonesia experienced the most severe financial crisis, so early detection is needed to
anticipate the impact of the crisis. The financial crisis can be detected by import and yen exchange rate to
rupiah indicators. In this paper, it used import and yen exchange rate to rupiah data from January 1990 to
December 2016 to form the model, while the data from January until December 2017 were used to validate
the model. To overcome the problem of structural change in the data, it is used Markov switching model,
while to detect the volatility shift it is used ARCH model and the combination of both models is Markov
switching ARCH (SWARCH) model. The aim of this study is to determine the appropriate model and to
detect financial crisis based on import and yen exchange rate to rupiah indicators. The results show that the
appropriate model for import and yen exchange rate to rupiah data is SWARCH(2,1). Based on the model, it
can be predicted that Indonesia will not experience a financial crisis in 2018.
1 INTRODUCTION
The financial crisis in Asia came from the fall in
currency values bath in Thailand in 1997. In 1997 and
1998, Indonesia experienced a financial crisis.
Currently, global trade is already unavoidable, and
the exchange rate affects the economy of a country.
For example, when the rupiah becomes more valuable
to foreign currencies, the price of imported goods will
be cheaper for the Indonesian population and
Indonesian export goods are more expensive for
foreign countries (Mishkin, 2008). There are 15
indicators that could be used to detect financial crisis
for example import, export, price stock, and rupiah
exchange rate (Kaminsky et al., 1998).
Engle (1982) uses the Autoregressive Conditional
Heteroscedasticity ( ARCH ) model for resolving the
problem of heteroscedasticity. Model ARCH could
not be used to cover the data that have structural
changes . Therefore, Hamilton (1989) used the
Markov switching model for resolving the problem of
structural changes on data. However, Markov
switching model cannot solve the problem of
volatility so Hamilton and Susmel 1994) used the
Markov switching ARCH (SWARCH) model to
overcome structural changes and volatility of the
data. The aim of this paper is to determine the
appropriate model of import and yen exchange rate to
rupiah data. The model is used to detect the financial
crisis in 2018.
2 THEORY
2.1 Autoregressive (AR) and
Autoregressive Conditional
Heteroscedasticity (ARCH) Model
An AR model is as follows
(1)
where r
t
is log return in the t
th
period which is
formulated as
,
is a parameter of AR
model at p
th
time, and
is residue at t
th
time (Tsay,
2005). The next model that we are used is ARCH (p)
model. The model could be written as
Zukhronah, E., Sugiyanto, . and Azizah, I.
Detection of Financial Crisis in Indonesia based on Import and Yen Exchange Rate to Rupiah Indicators using Combined of Volatility and Markov Switching Models.
DOI: 10.5220/0008519402050209
In Proceedings of the International Conference on Mathematics and Islam (ICMIs 2018), pages 205-209
ISBN: 978-989-758-407-7
Copyright
c
2020 by SCITEPRESS – Science and Technology Publications, Lda. All rights reserved
205