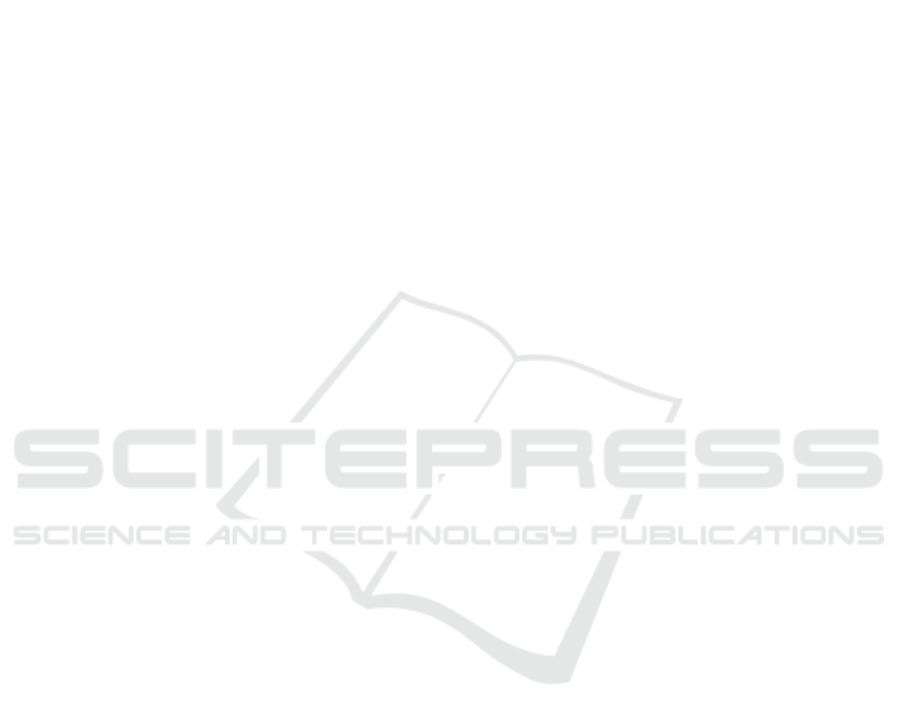
Financial Crisis Model in Indonesia Based on Indonesia Composite
Index (ICI) and Dollar (US) Exchange Rates to Rupiah Indicators
Sugiyanto, Isnandar Slamet, Sri Subanti, Etik Zukhronah and Winita Sulandari
Study Program of Statistics, Universitas Sebelas Maret Surakarta, Indonesia
Keywords: Crisis, ICI, Dollar Exchange Rates to Rupiah, SWARCH.
Abstract: An open economy is a new order of the world economic system that has provided space for all countries to
interact and have integrity with one another. The economy facilitates the entry of foreign investors, but it also
impacts the threat of financial crisis that is transmitted through increasingly open trade relations. The
movement of the Indonesia Composite Index (ICI) and the dollar (US) exchange rates to rupiah are used to
compile a model of the financial crisis in Indonesia. Crisis occurs due to high volatility and changing
conditions. Volatility models are used to explain volatility, while changes in conditions can be explained
through Markov switching. Therefore combining the volatility and Markov switching models is the best
solution to explain the crisis. The goal of this research is to find the model of the financial crisis in Indonesia
based on ICI and dollar (US) exchange rates to rupiah. The data that users are monthly data from 1990 to the
2017 year. The result showed that for ICI indicator the combining model is MS(2)-ARCH(1) or
SWARCH(2,1) model with a conditional average of AR (2). While based on the dollar (US) exchange rates
to rupiah indicator, SWARCH (3.3) model with conditional average of AR(1).
1 INTRODUCTION
The open economy system has presented challenges
to developing countries such as Indonesia, with the
integration of the country's financial sector. But on
the other hand, it can facilitate the spread of crises
between countries, as happened in 1997 when the
value of the Thailand currency fell sharply, and the
impact spread to various countries. The crisis is a
disruption of financial system stability in the
economic order. To maintain this stability, it is
necessary to monitor the occurrence of crisis, so that
prevention and crisis recovery efforts can be carried
out as early as possible.
Banking and capital markets in Indonesia become
indicators of financial systems that continue to
increase every year. This has caused the development
of capital market developments and the growth of the
banking sector because transactions in the capital
market are carried out through the banking system.
The higher the investment, the greater the savings and
the opportunity to provide funds which will
ultimately accelerate economic growth. The
Indonesia Composite Index (ICI) and the dollar
exchange rates to rupiah have a vulnerability to
economic stability shocks, this causes these indicators
to fluctuate and condition changes. In anticipation,
Hamilton and Susmel (1994) introduced the
Autoregressive Conditional Heteroscedasticity
Markov Switching (SWARCH) model which is a
combination of volatility and Markov switching
models as an alternative time series data modeling by
observing fluctuations and changes in conditions in
the data. Sugiyanto et al. (2017) through the
SWARCH model has shown that the bank deposits,
real exchange rates and terms of trade indicators can
explain the crisis of 1997, 1998 and 2008. Sugiyanto
et al. (2018) through the SWARCH model has shown
that the output real, domestic credit per GDP, and ICI
indicators can explain the crisis of 1997, 1998 and
2008. In this study a combination of volatility and
Markov switching models was formed which
corresponded to the ICI indicator and the dollar
exchange rates to rupiah to detect the financial crisis
in Indonesia.
46
Sugiyanto, ., Slamet, I., Zukhronah, E., Subanti, S. and Sulandari, W.
Financial Crisis Model in Indonesia Based on Indonesia Composite Index (ICI) and Dollar (US) Exchange Rates to Rupiah Indicators.
DOI: 10.5220/0008517000460051
In Proceedings of the International Conference on Mathematics and Islam (ICMIs 2018), pages 46-51
ISBN: 978-989-758-407-7
Copyright
c
2020 by SCITEPRESS – Science and Technology Publications, Lda. All rights reserved