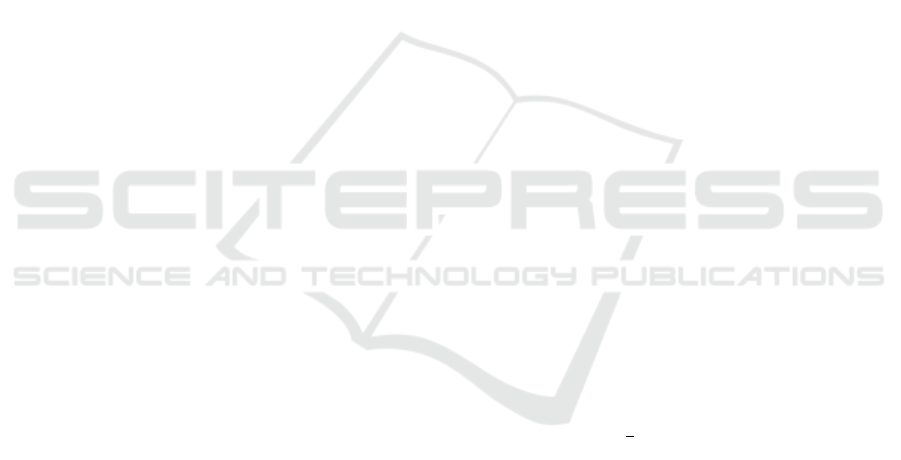
method have been carried out before. Research
conducted by Carr et.al (1986) applied disjunctive
kriging to estimate ground movements in the event of
an earthquake. Furthermore, Carr et al. (1989)
continued his research by comparing universal
kriging and ordinary kriging method to estimate the
ground motion in the event of an earthquake. Sugai et
al. (2015) introduced a practical method to estimate
the specific distribution of ground motion earthquake
events based on ordinary kriging analysis. Recent
research on the application of ordinary kriging was
carried out by Bekti et al. (2017) on the peak ground
acceleration (PGA) data in Banda Aceh in 2006 and
the results are Ordinary Kriging can be applied to
predict PGA.
The kriging method is considered as the best
estimation method in terms of the accuracy of its
assessment. The ordinary kriging method is a method
of estimating a random variable at a certain point
(location) by observing similar data in another
location with the mean data assumed to be constant
but unknown in value. This method is a method that
provides the best linear unbiased estimator (BLUE).
Hence, the ordinary kriging method can be used in
earthquake events. The other hand, in reality, the
population average of earthquake events is difficult to
be known.
Bengkulu Province is one of the provinces located
at the meeting of the Indo-Australia and Eurasia
tectonic plates which are the main generators of high
earthquake activity. The movements caused by these
two plates can cause active faults which are
generators of seismicity in parts of Sumatra.
Bengkulu is also among two active faults, the
Semangko and Mentawai faults. This condition
makes Bengkulu Province the most vulnerable to
earthquake disasters (Hadi et al., 2010).
Faisal (2014) compared Matheron (classic) and
Cressie-Hawkins (robust) isotropic semivariogram
models using the linear program method in the
earthquake event data sample in the coastal area of
Bengkulu. From the results of the case study, the
Spherical theoretical semivariogram model was the
best semivariogram model obtained from the results
of the Matheron isotropic experimental
semivariogram. However, in this study, the kriging
method has not been used to estimate earthquake
strength in Bengkulu area
Based on the previous research, we interested in
conducting further research. The purpose of this study
was to predict the strength of earthquakes in
Bengkulu Province and its surrounding areas based
on the epicenter of an earthquake that occurred in
some years. We use the ordinary kriging with the
isotropic semivariogram model. The final objective is
a mapping of the predicted strength of the earthquake
from the selected model. To facilitate the calculation
of the kriging and mapping, we use R software.
2 LITERATURE REVIEW
2.1 Semivariogram Analysis
Variogram is a tool in geostatistics that is used to
describe spatial correlations of data. In Plotting of
variogram, the low value will be close to other low
costs and large value will be near other large values.
The values can be visualized in a variogram graph as
a function of distance. Variogram determines the
distance where the values of the observation data are
independent or have no correlation. The symbol of the
variogram is 2γ, while semivariogram is half of the
variogram with the symbol γ. This semivariogram is
used to measure spatial correlation ( Wackernagel,
2003).
The experimental variogram is a variogram
calculated from the observed data. Variogram is
formulated as follows:
(1)
assume that the data are second order stationary,
equation (1) can be written as follows:
(2)
Since semivariogram is half of the variogram, then
from equation (2) can be written:
, (3)
where γ(h) is a semivariogram. The above
semivariogram is also called theoretical
semivariogram. There are two types of
semivariogram: isotropic semivariogram (γ(h)
depends only on distance h) and anisotropic
semivariogram (γ (h) depends on distance h and
direction).
A semivariogram is a statistical tool for
describing, modeling and explaining spatial
correlations between observations. An experimental
semivariogram is a semivariogram obtained from
known data. The estimator of semivariogram
proposed by Matheron (1962) in Cressie (1993) is:
Ordinary Kriging Method using Isotropic Semivariogram Model for Estimating the Earthquake Strength in Bengkulu Province
35