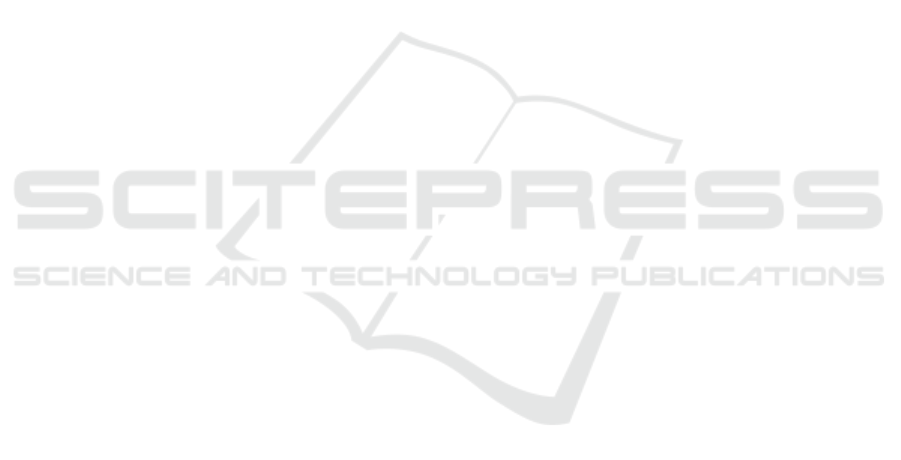
the worker's experience, the higher the decision to
migrate (settle) in Palembang City.
Educational variables have a coefficient value of
-0.184. and Odds Ratio value of 0.832. The results
show that the higher the education that is owned by
workers, the opportunity or opportunity to move the
job sector is 0.832 times lower than not moving the
work sector. But the influence of education variables
is not significant.
Education variables are not significant because
most respondents who have high school, D3, and S1
levels have the same opportunity to move the job
sector. The level of education can be accepted in any
sector. Even some respondents with secondary
school education are the most likely to move
because there are insiders who are known by some
respondents. In addition there are other factors,
namely their desire to change jobs or settle down.
Workers who have a higher level of education are
usually reluctant to move because they feel
comfortable with the current work environment.
Furthermore, the age variable of the age variable
has an Odds Ratio value of 0.942 with a coefficient
of -0.059. This means that the more the age of the
worker, the probability or probability of the worker
doing mobility becomes 0.942 lower. But education
variables also have no significant effect on the
mobility of workers between sectors in the city of
Palembang.
Based on the facts obtained in the field, the
reason for the age variable is insignificant, namely
that there are some workers whose ages are old but
are forced to change jobs because they are laid off
and have to find another job. The average worker
who is old and still working is the head of the family
and the backbone of the family.
5 CONCLUSION
The average wage of workers in the service sector is
Rp. 3,411,000. The average wage, which is only
Rp.2,294,000, can be classified as wages for workers
in the service sector. Experience and work is 7.18
years and things that are relevant to affordable work
in the service sector. The average level of education
in the service sector is secondary school, which can
be used in this sector, especially what is needed. The
average time given by workers in the service sector
is 30.8 years. This shows that workers in the service
sector are still relatively productive.
Variables that affect mobility between worker
sectors are wage variables and work experience.
Positive influential wage variables that provide high
yields are offered in sectors that generate a lot of
work in the Palembang city sector. Negative variable
work experience. If the work experience is longer,
the lower the chance to change jobs. education and
stock variables. While the level of education and the
influence of age are not significant.
REFERENCES
Agresti, Alan. 2007. An Introduction to Categorical Data
Analysis (Second Edition). New Jersey: John Willey &
Sons. Inc
Ahmed, Ayaz dan Ahsan, Henna. 2011. Contribution of
Service Sector in the economy of Pakistan. Vol 79.
Akkemik, K. Ali. 2005. Labor Productivity and Inter-
Sectoral Reallocation of Labor in Sigapore (1965-
2002). Forum of International Development Studies.
Alatas dan Trisilo. 1990. Struktur Ketenagakerjaan di
Indonesia, dalam Aris Ananta(ed). Ekonomi Sumber
Daya Manusia. LDFE-UI: Jakarta
Alexandi, Muhammad Findi. dan Marshafeni, Ovilla.
2013. Penyerapan Tenaga Kerja Pada Sektor
Pertanian dan Sektor Jasa Pasca Kebijakan Upah
Minimum di Provinsi Banten (Periode Tahun 2001-
2011). Jurnal Manajemen dan Agribisnis vol .10 No.2
Bungin, M Bungan. 2011. Metodologi Penelitian
Kuantitatif, Ekonomi, dan Kebijakan Publik Serta
Ilmu-Ilmu Sosial Lainnya. Kencana: Jakarta
Clark, C. 1941. The Condition of Economic Progress.
London: Macmillan
Euwals, R. 2001. Female Labor Supply, Flexibility of
Working hours, and Job Mobility. The Economic
Journal III (May) : C120-C134.
Fuchs, V. 1980. Economic Growth and The Rise of Service
Employment. Prepared for confrences: Towards
Explaining Economic Growth. Ninstitute of World
Economics. Kiel, Federal Republic of Germany.
Goldberg, L. Tracy J dan Aaronson, S.1999. Exchange
rate and Employment Instability : Evidence From
Matched CPS Data. The American review. (89) : 204-
210.
Herdianti, Yulia Maris. Burhan, M Umar. dan Pratomo,
Devanto Shasta. 2015. Analisis Transformasi Struktur
Ekonomi dan Perubahan Proporsi Tenaga Kerja
Provinsi Jawa Timur 1998-2012. Internasional Journal
of Social and Local Economic Governance.Vol 1
No.1 Hal.68-74
Hosmer, JR, David W., Stanley Lemeshow, Rodney X.
Sturdivant. 2013. Applied Logistic Regression (3
rd
edition). New Jersey : John Wiley& Sons Inc.
Kusreni, Sri. 2009. Pengaruh Perubahan Struktur
Ekonomi Terhadap Spesialisasi Sektoral dan Wilayah
Serta Struktur Penyerapan Tenaga Kerja Sektoral
Untuk Daerah Perkotaan di Jawa Timur. Majalah
Ekonomi. Vol. 19 No.1
Kuznets, S. 1957. Quantitative Aspects of The Economic
Growth of Nations. 11. Industrial Distribution of
National Product and Labor Forces.Economic
Economic and Socio-Demographic Factors of Labor Mobility in the Service Sector
385