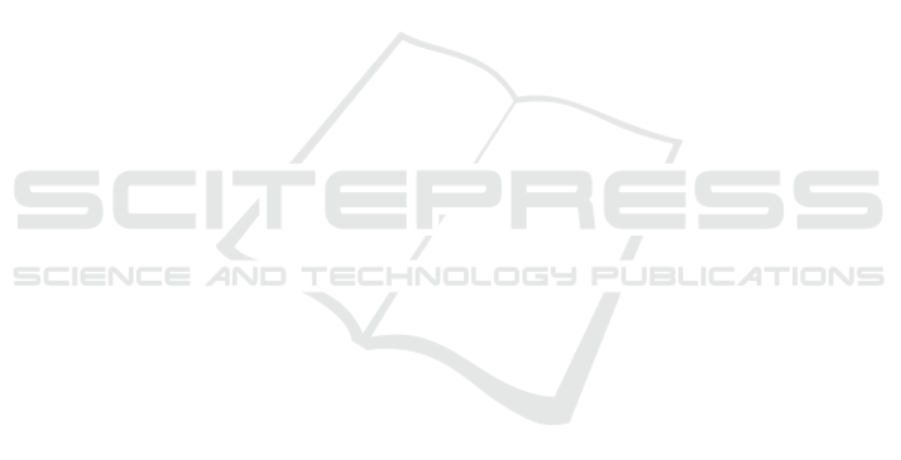
by X
8
(Percentage of Malnutrition) are West
Sulawesi, Central Sulawesi and West Kalimantan
Provinces. The percentage of poverty in other
provinces in Indonesia is influenced by more than
one variable. The 11 provinces influenced by
variables X
1
and X
8
are North Sumatra Province,
West Sumatera Province, Riau Province, Jambi
Province, South Sumatera Province, Lampung
Province, Bangka Belitung Island. One province
affected by variables X
6
and X
8
is the province of
Papua. The two provinces affected by variables X
1
,
X
5
and X
7
are Bengkulu and Gorontalo Provinces.
One province affected by variables X
1
, X
6
and X
7
is
Aceh Province. A total of 9 provinces or 26.47% of
the percentage of poverty is not affected by X
1
, X
2
,
X
3
, X
4
, X
5
, X
6
, X
7
, X
8
and X
9
.
The overall classification accuracy is 79.41%.
The result of the estimation of areas with low
poverty level is 10 regions being classified into low
poverty level, while the regions wrongly classified
(from low to high) are 7 regions, namely Jambi,
Kep. Bangka Belitung, Bali Province, West
Kalimantan Province, North Kalimantan Province,
South Sulawesi Province, and North Maluku
Province with classification accuracy of 58.20%.
Meanwhile, there are 17 regions classified into high
poverty level appropriately classified into the
category of high poverty level with 100%
classification accuracy.
5 CONCLUSION
In 2017, as many as 17 (50%) of Indonesian
provinces have a high percentage of poverty, and the
province with the highest percentage of poverty is
the Province of Papua with 28.4%, while the lowest
percentage of poverty is present in DKI Jakarta
Province with 3.75%. Based on the results of the
analysis, the best model for the percentage of
poverty using Kernel functional weights is the Fixed
Gaussian.
The variables that significantly influence the
percentage of poverty are literacy rate (X
1
),
percentage of household with proper sanitation (X
5
),
percentage of slum household (X
6
), percentage of
households occupying habitable homes (X
7
),
percentage of malnutrition (X
8
). Based on the
GWLR model, the higher the literacy rate, the
percentage of households with proper sanitation, and
the percentage of households living in habitable
homes, the greater the tendency of a province to
have a lower percentage of poverty, while the higher
the percentage of slum houses and malnutrition
percentage, the higher the propensity for a province
to have a high percentage of poverty.
From the results of the discussion, the two
provinces with the highest percentage of poverty
were Papua and West Papua Provinces. Papua
Province is influenced by variable percentage of
slum household (X
6
) and malnutrition percentage
(X
8
). The higher the percentage of slum household
(X
6
) and malnutrition percentage (X
8
), the higher the
percentage of poverty. The Province of West Papua
is influenced by the percentage variable of proper
sanitation (X
5
). The lower the percentage of a proper
sanitation RT, the higher the percentage rate of
poverty.
As a suggestion, the government should pay
more attention to provinces with high-category
poverty rates, such as Papua and West Papua. For
the province of Papua, the government should build
housing and provide health treatment for people
affected by malnutrition. As for the Province of
West Papua, the government should hold
socialization programs and assist in the development
of proper sanitation. In addition to an active
government, it is hoped that the Indonesian people
will also support the government's programs in the
success of SDGs 2030 with one of its objectives
being to alleviate poverty in Indonesia.
REFERENCES
Chamidah, N., Saifuddin, T., & Marisa, R. (2014). The
Vulnerability Modeling of Dengue Hemorrhagic Fever
Disease in Surabaya Based on Spatial Logistic
Regression Approach. Applied Mathematical Sciences
HIKARI. 28. 1369 – 1379.
Hastuti dan Syakur. (2017). Spatial Characteristics of
Slum Settlement of South Sulawesi Province. Palopo:
Universitas Cokroaminoto Palopo. (Text in
Indonesian)
Kurnia, A. (2011). Modeling of Logistic Regression
Analysis and Geographically Weighted Regression
Semiparametric (Case Study: Human Development
Index Modeling of East Java Province Year 2008).
Surabaya: Institut Teknologi Sepuluh November,
2011. (Text in Indonesian)
Rusdarti dan Karolina, S. 2013. Factors Affecting Poverty
Level in Central Java Province. Semarang:
Universitas Negeri Semarang. (Text in Indonesian)
Sumekar S., dan Haryadi U. 2016. Socialization of
Sustainable Development Goals (SDGs)
Implementation in the Library. Perpusnas. (Text in
Indonesian)
World Bank. 2006. A New Era in Poverty Alleviation in
Indonesia. Jakarta: The World Bank Office Jakarta.
(Text in Indonesian)
ICPS 2018 - 2nd International Conference Postgraduate School
938