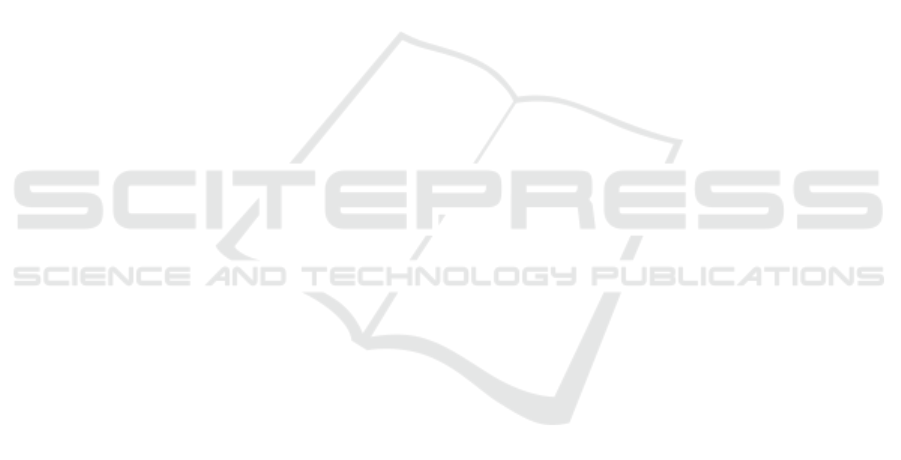
Desquesnes, G., Lozenguez, G., Doniec, A., and Duviella,
E. (2016). Dealing with large mdps, case study of
waterway networks supervision. Advances in Distri-
buted Computing and Artificial Intelligence Journal,
5:71–84.
Ducharne, A., Habets, F., Pag
´
e, C., Sauquet, E., Viennot,
P., D
´
equ
´
e, M., Gascoin, S., Hachour, A., Martin, E.,
Oudin, L., Terray, L., and Thi
´
ery, D. (2010). Cli-
mate change impacts on water resources and hydro-
logical extremes in northern france. XVIII Conference
on Computational Methods in Water Resources, June,
Barcelona, Spain.
Duviella, E., Doniec, A., and Nouasse, H. (2018). Adap-
tive water-resource allocation planning of inland wa-
terways in the context of global change. Journal of
water resources planning and management - Accep-
ted.
Duviella, E., Nouasse, H., Doniec, A., and Chuquet, K.
(2016). Dynamic optimization approaches for re-
source allocation planning in inland navigation net-
works. ETFA2016, Berlin, Germany, September 6-9.
Duviella, E., Rajaoarisoa, L., Blesa, J., and Chuquet, K.
(2013). Adaptive and predictive control architecture
of inland navigation networks in a global change con-
text: application to the cuinchy-fontinettes reach. in
IFAC MIM conference, Saint Petersburg, 19-21 June.
EnviCom (2008). Climate change and navigation - water-
borne transport, ports and waterways: A review of cli-
mate change drivers, impacts, responses and mitiga-
tion. EnviCom - Task Group 3.
IWAC (2009). Climate change mitigation and adaptation.
implications for inland waterways in england and wa-
les. Report.
Jonkeren, O., Rietveld, P., and van Ommeren, J. (2007). Cli-
mate change and inland waterway transport: welfare
effects of low water levels on the river rhine. Journal
of Transport Economics and Policy, 41:387–412.
Koetse, M. J. and Rietveld, P. (2009). The impact of cli-
mate change and weather on transport: An overview
of empirical findings. Transportation Research Part
D: Transport and Environment, 14(3):205 – 221.
Nouasse, H., Doniec, A., Duviella, E., and Chuquet, K.
(2016a). Efficient management of inland navigation
reaches equipped with lift pumps in a climate change
context. 4
th
IAHR Europe Congress, Liege, Belgium
27-29 July.
Nouasse, H., Doniec, A., Lozenguez, G., Duviella, E., Chi-
ron, P., Archim
`
ede, B., and Chuquet, K. (2016b). Con-
straint satisfaction problem based on flow transport
graph to study the resilience of inland navigation net-
works in a climate change context. IFAC Conference
MIM, Troyes, France, 28-30 June.
Nouasse, H., Horv
`
ath, K., Rajaoarisoa, L., Doniec, A., Du-
viella, E., and Chuquet, K. (2016c). Study of global
change impacts on the inland navigation management:
Application on the nord-pas de calais network. Trans-
port Research Arena, Varsovie, Poland.
Nouasse, H., Rajaoarisoa, L., Doniec, A., Chiron, P., Du-
viella, E., Archim
`
ede, B., and Chuquet, K. (2015).
Study of drought impact on inland navigation systems
based on a flow network model. ICAT, Sarajevo, Bos-
nie Herzegovia.
Tafidis, P., Macedo, E., Coelho, M., Niculescu, M., Voicu,
A., Barbu, C., Jianu, N., Pocostales, F., Laranjeira, C.,
and Bandeira, J. (2017). Exploring the impact of ict on
urban mobility in heterogenic regions. Transportation
Research Procedia, 27:309 – 316. 20th EURO Wor-
king Group on Transportation Meeting, EWGT 2017,
4-6 September 2017, Budapest, Hungary.
Wang, S., Kang, S., Zhang, L., and Li, F. (2007). Model-
ling hydrological response to different land-use and
climate change scenarios in the zamu river basin of
northwest china. Hydrological Processes, 22:2502–
2510.
ICINCO 2018 - 15th International Conference on Informatics in Control, Automation and Robotics
312