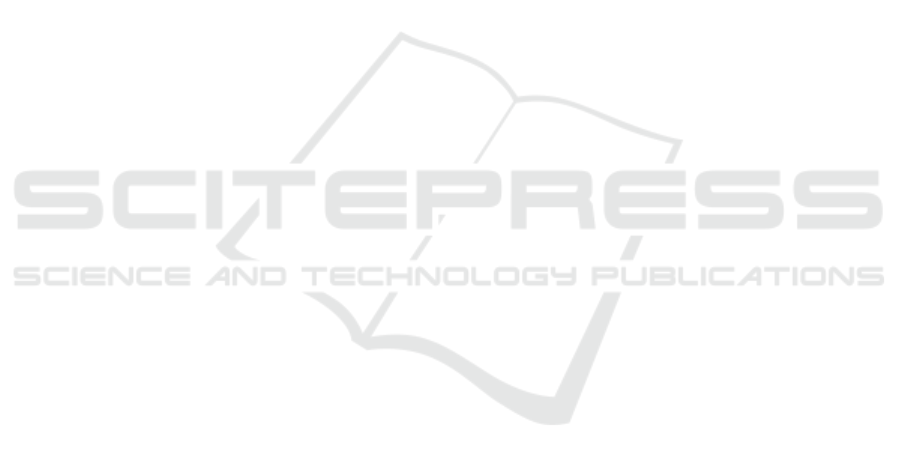
DFG (2015). Leitlinien zum umgang mit forschungsdaten.
http://www.allianzinitiative.de/de/handlungsfelder/
forschungsdaten/grundsaetze.html.
DIN (2017). Din spec 91357: Referenzarchi-
tekturmodell offene urbane plattform (oup).
https://www.din.de/de/wdc-beuth:din21:281077528.
Edwards, J. and Wolfe, S. (2005). Compliance: A re-
view. Journal of Financial Regulation and Compli-
ance, 13(1):48–59.
European Comission (2003). Directive 2003/98/ec
of the european parliament and of the council
of 17 november 2003 on the re-use of public
sector information. https://eur-lex.europa.eu/legal-
content/en/TXT/?uri=CELEX:32003L0098.
European Comission (2016). General data protection re-
gulation (gdpr): Regulation (eu) 2016/679 of the
european parliament and of the council of 27 april
2016 on the protection of natural persons with re-
gard to the processing of personal data and on
the free movement of such data, and repealing
directive 95/46/ec. http://eur-lex.europa.eu/legal-
content/EN/TXT/?uri=CELEX:32016R0679.
European Commission (2016). Open innovation, open
science, open to the world: A vision for Europe. Publi-
cations Office of the European Union, Luxembourg.
Ferle, C. H. and Spath, D., editors (2012). Marktstudie di-
gitale Langzeitarchivierung: Im Spannungsfeld zwis-
chen Digital Preservation und Enterprise Information
Archiving. Fraunhofer Verl., Stuttgart.
Glowalla, P. and Sunyaev, A. (2013). Process-driven data
quality management through integration of data qua-
lity into existing process models. Business & Infor-
mation Systems Engineering, 5(6):433–448.
Kindling, M. (2013). Qualitätssicherung im umgang mit di-
gitalen forschungsdaten. 2013, H. 1-6, 2 (2013):137–
147.
KoSIT (2018). Xöv-standards. https://www.xoev.de/.
Maletic, J. I. and Marcus, A. (2005). Data cleansing. In
Data Mining and Knowledge Discovery Handbook,
pages 21–36. Springer.
Morbey, G. (2011). Datenqualität für Entscheider in Unter-
nehmen: Ein Dialog zwischen einem Unternehmens-
lenker und einem DQ-Experten. Gabler Verlag /
Springer Fachmedien, Wiesbaden, 1. aufl. edition.
Mosley, M. (2008). Dama-dmbok functional framework
version 3. DAMA International.
OECD (2007). Oecd principles and guidelines for acces to
research data from public funding. https://www.oecd-
ilibrary.org/docserver/9789264034020-en-fr.pdf.
Otto, B. (2011). A morphology of the organisation of data
governance. In ECIS, volume 20, page 1.
Otto, B., Kokemüller, J., Weisbecker, A., and Gizanis, D.
(2011). Stammdatenmanagement: Datenqualität für
geschäftsprozesse. HMD - Praxis der Wirtschaftsin-
formatik, 48(279):5–15.
Otto, B. and Österle, H. (2016). Corporate Data Quality:
Voraussetzung erfolgreicher Geschäftsmodelle. Ga-
bler and Springer Gabler, Berlin, 1. aufl. 2016 edition.
Panian, Z. (2010). Some practical experiences in data go-
vernance. World Acad. Sci. Eng. Technol, 38:150–157.
Paskaleva, K., Evans, J., Martin, C., Linjordet, T., Yang,
D., and Karvonen, A. (2017). Data governance in the
sustainable smart city. Informatics, 4(4):41.
Pentek, T., Legner, C., and Otto, B. (2017). Towards a
reference model for data management in the digital
economy. In Maedche, A., Vom Brocke, J., and Hev-
ner, A., editors, Designing the Digital Transformation.
Karlsruhe.
Pfau-Weller, N. and Radecki, A. v. (2018). Was macht st-
ädte smart? – die morgenstadt-initiative: In der mor-
genstadt initiative entwickelt die fraunhofer gesell-
schaft gemeinsam mit partnern aus industrie und kom-
munen lösungen für die stadt der zukunft.
Piro, A., editor (2014). Informationsqualität bewerten:
Grundlagen, Methoden, Praxisbeispiele. Symposion,
Düsseldorf, 1. aufl. edition.
Rusev, S. J. and Salonitis, K. (2016). Operational excellence
assessment framework for manufacturing companies.
Procedia CIRP, 55:272–277.
Saujot, M. and Erard, T. (2015). Smart city innovations for
sustainable cities? an analysis based on data challen-
ges. https://www.iddri.org/sites/default/files/import/
publications/wp0215en.pdf.
Scheuch, R., Gansor, T., and Ziller, C. (2012). Master
Data Management: Strategie, Organisation, Architek-
tur. dpunkt-Verlag, Heidelberg, 1. aufl. edition.
Schuh, G., Anderl, R., Gausemeier, J., ten Hompel, M., and
Wahlster, W., editors (2017). Industrie 4.0 Maturity
Index: Managing the Digital Transformation of Com-
panies. Acatech Studie. Utz, Herbert, München.
Soto, F. (2013). A better definition of operational excel-
lence. http://www.wilsonperumal.com/blog/a-better-
definition-of-operational-excellence.
Wang, R. Y. (1998). A product perspective on total data
quality management. Communications of the ACM,
41(2):58–65.
Wang, R. Y. and Strong, D. M. (1996). Beyond accuracy:
What data quality means to data consumers. Journal
of management information systems, 12(4):5–33.
Weber, M. and Weber, M. (1980). Wirtschaft und Ge-
sellschaft: Grundriss der verstehenden Soziologie.
J.C.B. Mohr (Paul Siebeck), Tübingen, studienaus-
gabe, fünfte, revidierte auflage edition.
Wecker, G. and Ohl, B., editors (2013). Compliance in der
Unternehmerpraxis: Grundlagen, Organisation und
Umsetzung. Springer Gabler, Wiesbaden, 3. aufl. 2013
edition. http://dx.doi.org/10.1007/978-3-658-00893-
2.
Wilkinson, M. D. and other (2016). The fair guiding princi-
ples for scientific data management and stewardship.
Scientific data, 3:160018.
Zeng, M. (2004). Metadata types & functions.
http://marciazeng.slis.kent.edu/metadatabasics/
types.htm.
DATA 2018 - 7th International Conference on Data Science, Technology and Applications
252