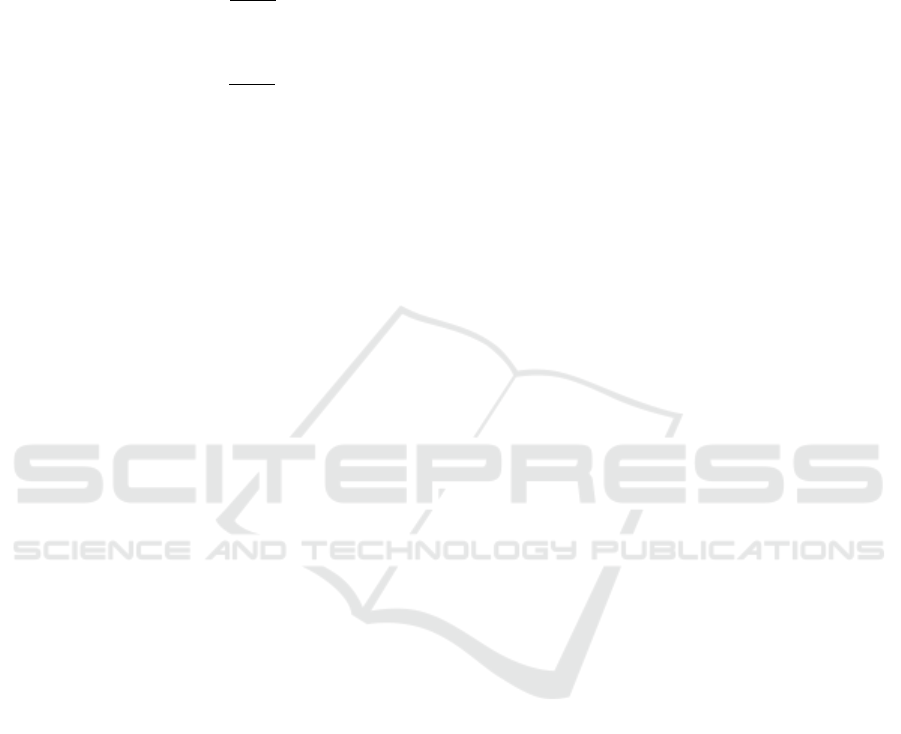
following equations must therefore be fulfilled for all
t ∈ [0, 96 − 1]:
−0.85 · P
t,CHP,c,G
= P
t,CHP,c,H
+ P
t,CHP,c,E
and
−0.85 · P
t,CHP,c,G
=
0.85
0.435
· P
t,CHP,c,E
and
−0.85 · P
t,CHP,c,G
=
0.85
0.415
· P
t,CHP,c,H
This example shows that the generic description of
flexibility made in this paper is suitable for applying
it to a wide range of cells.
5 CONCLUSION AND OUTLOOK
In order to create a common communication basis
for cells of different types enabling the exchange of
information regarding existing and thus usable flexi-
bility, a generic definition of flexibility is developed
in this paper. This is particularly important against
the background of the energy transition, since the
use of flexibility within a cellularly structured energy
system is one of the most widely pursued approaches
to deal with the challenges of an increasingly decen-
tralised energy system.
The basic idea is to define flexibility within cells
containing any number of plants and networks as
the set of all possible and permissible load profiles.
This can be described as a subset of R
p·T
, where
p describes the number of all existing connections
between plants and networks and T the number of
considered time steps. Each point defines a complete
load profile for all networks and all plants for a
pre-defined, future time interval. In order to deter-
mine the subset, conditions have been introduced
which include interdependencies between plants and
laws for energy conservation within networks and
individual plants. Further criteria for the definition of
flexibility are that it creates a suitable solution space
for load optimization problems within cells, enables
easy integration into higher-level cells and supports
the modelling of power flows at plant level.
Individual sub aspects in the plant behaviour, such
as start-up ramps, energy loss in networks or power-
dependent efficiency ratios, are currently still being
neglected, as these would not change the basic idea
of describing the system, but would create additional
complexity. However, such aspects can be added to
the given definition at any time.
Based on the now generally defined concept of
flexibility, it is to be investigated how it can be embed-
ded in different methods for the optimization of load
profiles within but also across cells. This also raises
the question of the visualization ability of flexibility,
which is currently still implicitly described by boun-
dary conditions to the permissible subset of R
p·T
.
Therefore, this aspect is also closely related to the in-
vestigation of possibilities for an explicit representa-
tion of flexibility. It may be necessary to make further
simplifying assumptions on the energy systems under
consideration in order to facilitate the application of
the definition within the context of load optimization
in real cells.
In addition, another application objective of the flexi-
bility definition given in this paper is to be seen in the
context of fleet management of electric vehicles. The
energy management system of a shared electric vehi-
cle fleet described in (Ostermann and Koetter, 2016)
implements the flexibility idea presented in this paper
for a special case in practice. This offers a possible
application case in which a transfer of the theoreti-
cal definition into practice can be the subject of future
research work.
ACKNOWLEDGEMENTS
The work published in this article was funded by
the Bundesministerium f
¨
ur Wirtschaft und Energie
(BMWi) under the project C/sells under the promo-
tional reference 03SIN125.
REFERENCES
Allerding, F., Mauser, I., and Schmeck, H. (2014). Customi-
zable Energy Management in Smart Buildings Using
Evolutionary Algorithms, pages 153–164. Springer
Berlin Heidelberg, Berlin, Heidelberg.
Gellings, C. W. (1985). The concept of demand-side mana-
gement for electric utilities. Proceedings of the IEEE,
73(10):1468–1470.
Gottwald, S., Ketter, W., Block, C., Collins, J., and Wein-
hardt, C. (2011). Demand side management - a si-
mulation of household behavior under variable prices.
Energy Policy, 39(12):8163–8174.
Greer, C., Wollman, D., Prochaska, D., Boynton, P., Mazer,
J., Nguyen, C., FritzPatrick, G., Nelson, T., Koepke,
G., and Jr, A. H. (2014). Nist framework and road-
map for smart grid interoperability standards. Techni-
cal Report 3.0, US National Institute of Standards and
Technology.
Khoury, J., Mbayed, R., Salloum, G., and Monmasson,
E. (2016). Predictive demand side management of
a residential house under intermittent primary energy
SMARTGREENS 2018 - 7th International Conference on Smart Cities and Green ICT Systems
138