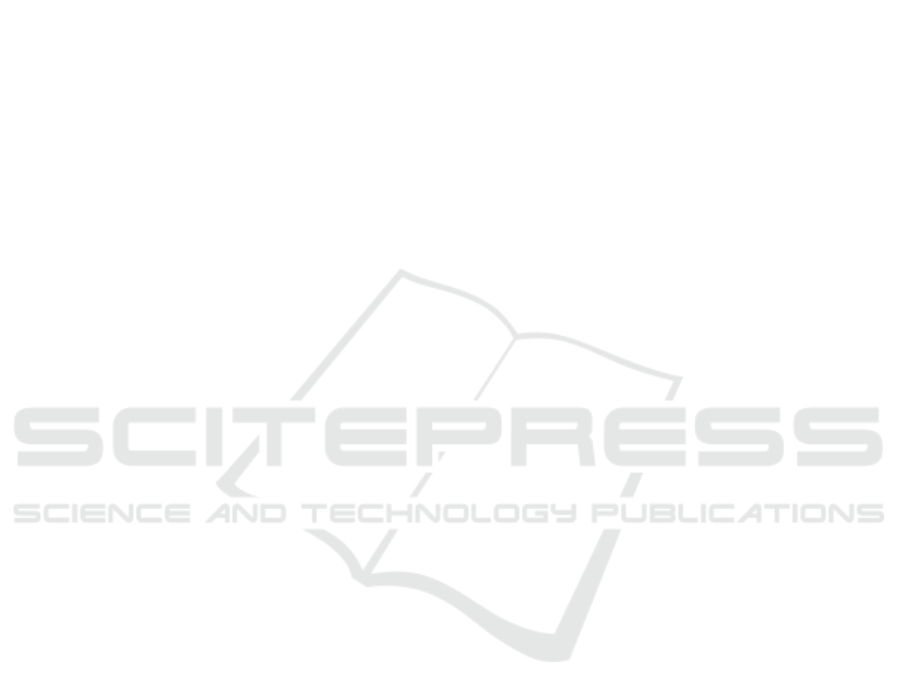
Intelligent Thermal Control Method for Small-Size Air Conditioning
System
Hung-Wen Lin
1
, Min-Der Wu
1
, Guan-Wen Chen
1
and Ying Xuan Tan
2
1
Green Energy and Research Laboratories, Industrial Technology Research Institute, Hsinchu, Taiwan
2
Department of Mechanical Engineering, University of Malaya, Kuala Lumpur, Malaysia
Keywords: HVAC, Least Enthalpy Difference Theory, Energy Saving.
Abstract: To decrease the energy consumption and maintaining the comfort of the area, a great deal of work has been
done on HVAC control algorithms. A control system with the least enthalpy difference theory applied is
proposed in this paper. By using the indoor air temperature and relative humidity as the feedback of the control
system, the temperature set for the air conditioner is able to satisfy the indoor thermal comfort. The simulation
and experimental results of this controller have shown positive energy saving while maintaining indoor
thermal comfort.
1 INTRODUCTION
Due to the significant increase of energy consumption
in buildings, energy saving strategies have become
the first priority in energy policies in most countries
around the world. In 2006, United States of America
had used about 35% of the total energy for HVAC
systems (US EIA, 2017). About 50% of the world’s
total electrical energy is consumed by HVAC systems
(Fagan, Refai and Tachwali, 2007). However, most of
the medium-small commercial building is still using
small typed of HVAC control system.
Many approaches have been published by
researchers including the algorithms to control the
energy of HVAC systems. A classification of the
control systems named model predictive control
(MPC) were presented including classical control,
hard control, soft control, hybrid control and other
control techniques (Afram and Farrokh, 2014). The
research then focused on the comprehensive review
of MPC techniques and comparisons with other
control techniques. Generally, MPC provides
superior performance in terms of lower energy
consumption, better transient response, robustness to
disturbances and consistent performance under
varying conditions. An HVAC control strategies
which exploit the existence of a Wireless Sensor
Network (WSN) which is capable of distributing
temperature and zone occupancy information was
analyzed. The research focused on a technique called
“Adaptive Algorithm” which requires an additional
parameter which is the expected residence time of the
occupants for each zone to be controlled (Dimitris,
Evangelos, John and Odysseus, 2014). An
occupancy-based feedback control algorithm for
variable-air volume HVAC systems that is applicable
to the under-actuated case in which multiple rooms
share the same HVAC equipment was implemented.
Despite the inability to condition rooms
independently, comfort was found to be well
maintained and significant energy savings was
offered (Jonathan, Saket, Siddharth, Rahul and
Prabir, 2014).
In this paper, a method of using the least enthalpy
difference theory is proposed. Focusing on small
commercial buildings, experiments have been done to
apply the least enthalpy difference algorithm which
provides the optimal setting of the dry bulb
temperature and relative humidity for the air
conditioning system. With this, the intelligent sensing
control system with the theory applied is built to
obtain an energy saving algorithm.
2 METHODOLOGY
This study focused on reducing the energy
consumption of HVAC system of small commercial
buildings while maintaining the indoor comfort. The
least enthalpy difference theory is introduced and
64
Lin, H., Wu, M., Chen, G. and Tan, Y.
Intelligent Thermal Control Method for Small-Size Air Conditioning System.
DOI: 10.5220/0006690900640069
In Proceedings of the 7th International Conference on Smart Cities and Green ICT Systems (SMARTGREENS 2018), pages 64-69
ISBN: 978-989-758-292-9
Copyright
c
2019 by SCITEPRESS – Science and Technology Publications, Lda. All rights reserved