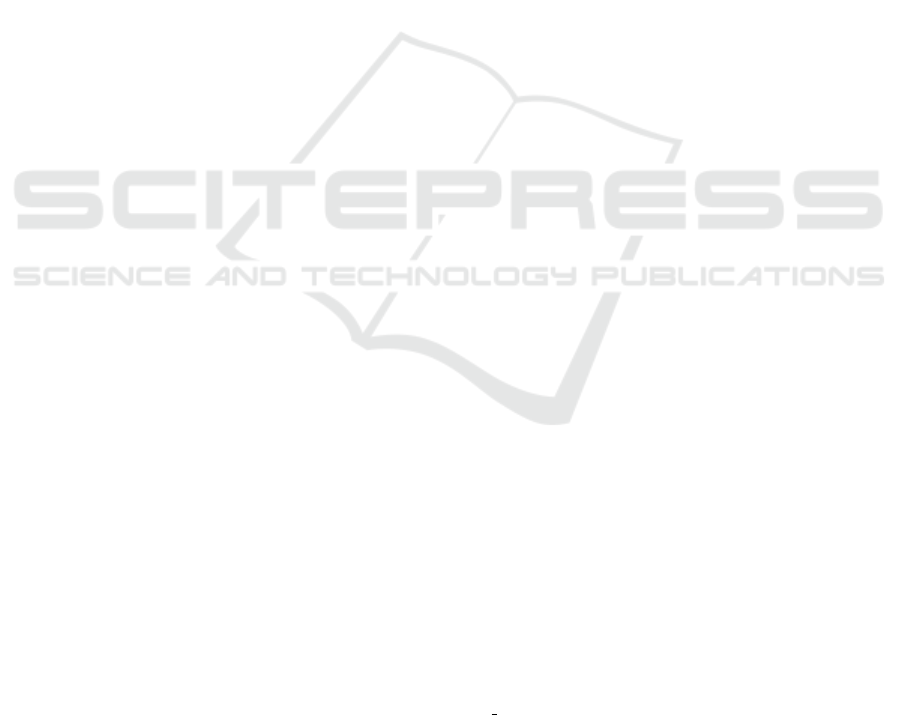
16. Chou, Y.k.: Actionable gamification. Beyond Points, Badges, and Leaderboards (2015)
17. Ciabattoni, L., Ferracuti, F., Longhi, S., Pepa, L., Romeo, L., Verdini, F.: Real-time mental
stress detection based on smartwatch. In: Consumer Electronics (ICCE), 2017 IEEE Interna-
tional Conference on. pp. 110–111. IEEE (2017)
18. Cristea, M., Paran, F., Delhomme, P.: The role of motivations for eco-driving and social
norms on behavioural intentions regarding speed limits and time headway. World Academy
of Science, Engineering and Technology 6, 1307–6884 (2012)
19. De Santos Sierra, A., Sanchez Avila, C., Guerra Casanova, J., Bailador Del Pozo, G.: A
stress-detection system based on physiological signals and fuzzy logic. IEEE Transactions on
Industrial Electronics 58(10), 4857–4865 (2011). https://doi.org/10.1109/TIE.2010.2103538
20. del R Millan, J., Mourino, J., Franze, M., Cincotti, F., Varsta, M., Heikkonen, J., Ba-
biloni, F.: A local neural classifier for the recognition of EEG patterns associated
to mental tasks. IEEE Transactions on Neural Networks (3), 678–686 (may 2002).
https://doi.org/10.1109/TNN.2002.1000132
21. DriveSim: Drivesim simulator (2014), http://drivesimsimulator.com/en/
22. Eco-Drive, Q.A.: Ecodriver (2015), http://www.play-ecodriver.ch/en/
23. Electrophysiology, T.F.o.t.E.S.o.C.t.N.A.S.o.P.: Heart Rate Variability : Standards of Mea-
surement, Physiological Interpretation, and Clinical Use. Circulation 93(5), 1043–1065 (mar
1996). https://doi.org/10.1161/01.CIR.93.5.1043
24. Fiat: eco:drive app (2014), http://ecodrive.driveuconnect.eu/
25. Ford: Smart eco driving (2013), https://ford.devpost.com/submissions/16640-smart-eco-
driving
26. Hobert, L., Festag, A., Llatser, I., Altomare, L., Visintainer, F., Kovacs, A.: Enhancements
of v2x communication in support of cooperative autonomous driving. IEEE communications
magazine 53(12), 64–70 (2015)
27. Honda: Insight eco assist system (2017), http://world.honda.com/INSIGHT/eco/
28. Kalofolias, V., Bresson, X., Bronstein, M., Vandergheynst, P.: Matrix completion on graphs.
arXiv preprint arXiv:1408.1717 (2014)
29. Kontaxis, S., L
´
azaro, J., Hernando, A., Arza, A., Garz
´
on, J.M., Gil, E., Laguna, P., Aguil
´
o,
J., Bail
´
on, R.: Mental stress detection using cardiorespiratory wavelet cross-bispectrum. In:
Computing in Cardiology Conference (CinC), 2016. pp. 725–728. IEEE (2016)
30. Kumar, M., Weippert, M., Vilbrandt, R., Kreuzfeld, S., Stoll, R.: Fuzzy Evaluation of Heart
Rate Signals for Mental Stress Assessment. IEEE Transactions on Fuzzy Systems 15(5),
791–808 (oct 2007). https://doi.org/10.1109/TFUZZ.2006.889825
31. Lauper, E., Moser, S., Fischer, M., Matthies, E., Kaufmann-Hayoz, R.: Psychological predic-
tors of eco-driving: A longitudinal study. Transportation research part F: traffic psychology
and behaviour 33, 27–37 (2015)
32. Magana, V.C., Munoz-Organero, M.: Gafu: using a gamification tool to save fuel. IEEE
Intelligent Transportation Systems Magazine 7(2), 58–70 (2015)
33. McDuff, D., Gontarek, S., Picard, R.: Remote measurement of cognitive stress via
heart rate variability. In: 2014 36th Annual International Conference of the IEEE
Engineering in Medicine and Biology Society. pp. 2957–2960. IEEE (aug 2014).
https://doi.org/10.1109/EMBC.2014.6944243
34. Mensing, F., Bideaux, E., Trigui, R., Ribet, J., Jeanneret, B.: Eco-driving: An economic or
ecologic driving style? Transportation Research Part C: Emerging Technologies 38, 110–121
(2014)
35. Mili, G.: Low to High Frequency Ratio of Heart Rate Variability Spectra Fails to Describe
Sympatho-Vagal Balance in Cardiac Patients. Coll. Antropol 29172(1), 295–300 (2005)
36. Mitsubishi: Eco drive support (2013), http://www.mitsubishi-
motors.com/en/innovation/technology/library/eco drive.html
121
Gamification of EcoDriving Behaviours through Intelligent Management of Dynamic Car and Driver Information
121