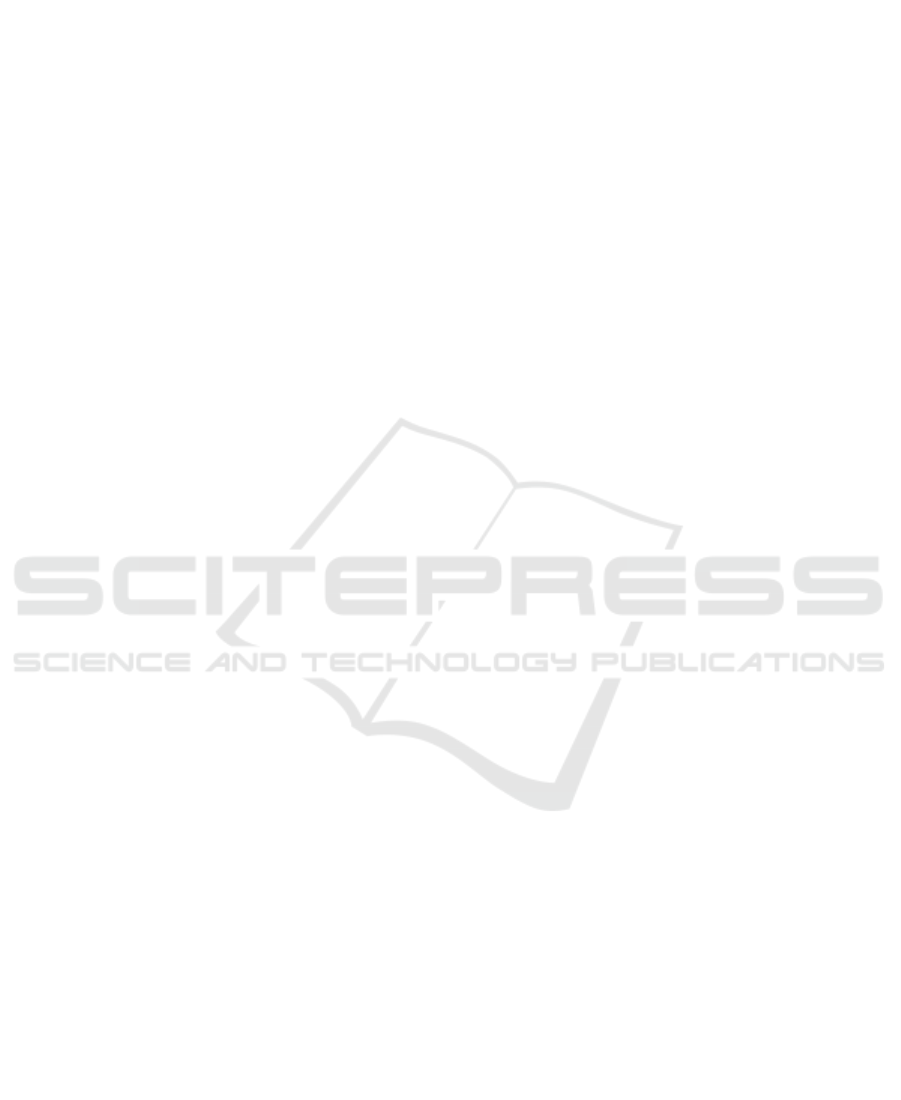
9. Kayyali, H., Weimer, S., Frederick, C., Martin, C., Basa, D., Juguilon, J., & Jugilioni, F.
(2008). Remotely attended home monitoring of sleep disorders. Telemedicine journal and
e-health, 14(4):371–4.
10. Pinto, A., Almeida, J. P., Pinto, S., Pereira, J., Oliveira, A.G., & de Carvalho, M. (2010).
Home telemonitoring of non-invasive ventilation decreases healthcare utilisation in a pro-
spective controlled trial of patients with amyotrophic lateral sclerosis. Journal of Neurology,
Neurosurgery & Psychiatry, 81(11):1238-42.
11. Lim, J.J., Zitnick, C.L., & Dollár P. (2013). Sketch Tokens: A Learned Mid- level Repre-
sentation for Contour and Object Detection." CVPR, 2013. IEEE Conference on Computer
Vision and Pattern Recognition,
12. Girshick, R.B., Felzenszwalb, P.F., & Mcallester, D.A. (2011). Object detection with gram-
mar models. Advances in Neural Information Processing Systems. Available at:
http://cs.brown.edu/people/pfelzens/papers/grammar-nips11.pdf.
13. Yang, Y., Ge, S.S., & Wang, L.C. (2008). Facial expression recognition and tracking for
intelligent human-robot interaction. Intelligent Service Robotics,1,2: 143-157.
14. Xiao, R., Li, M., & Zhang, H. (2004). Robust multipose face detection in images. Circuits
and Systems for Video Technology, IEEE Transactions on, 14,1: 31-41.
15. Morimoto, C.H., & Mimica M. (2005). Eye gaze tracking techniques for interactive appli-
cations. Computer Vision and Image Understanding, 98,1: 4-24.
16. Guha, T., & Ward, R.K. (2012). Learning Sparse Representations for Human Action Recog-
nition. Pattern Analysis and Machine Intelligence, IEEE Transactions on, 34, 8:1576,1588.
17. Zhang, R., Vogler, C.H., & Metaxas, D. (2007). Human gait recognition at sagittal plane.
Image and Vision Computing, 25, 3:321–30.
18. Saponaro, G., Salvi, G., & Bernardino, A. (2013). Robot Anticipation of Human Intentions
through Continuous Gesture Recognition, In International Conference on Collaboration
Technologies and Systems (CTS). doi: 10.1109/CTS.2013.6567232.
19. Goffredo, M., Seely, R.D., Carter, J.N., & Nixon, M.S. (2008). Markerless view independent
gait analysis with self-camera calibration, In Proceedings of International Conference on
Automatic Face and Gesture Recognition. doi: 10.1109/AFGR.2008.4813366
20. Garn, A.C., Baker, B.L., Beasley, E.K., & Solmon, M.A. (2012). What are the benefits of a
commercial exergaming platform for college students? Examining physical activity, enjoy-
ment, and future intentions. Journal of physical activity & health, 9, 2:311.
21. Staiano, A.E., & Sandra L.C. (2011). Exergames for physical education courses: Physical,
social, and cognitive benefits. Child Development Perspectives, 5,2: 93- 98.
22. Ding, D., Liu, H.Y., Cooper, R., Cooper, R.A., Smailagic, A., & Siewiorek, D. (2010). Vir-
tual Coach Technology for Supporting Self-Care. Physical Medicine and Rehabilitation
Clinics of North America, 21(1), 179-194.
23. Siewiorek, D., Smailagic, A., & Dey, A. (2012). Architecture and Applications of Virtual
Coaches. In Proceedings of the IEEE, Special Issue on Quality of Life Technology.
Doi:10.1109/JPROC.2012.2200560.
24. Smailagic, A., Siewiorek, D., Chakravarthula, S., Kar, A., Jagdale, N., Gautam, S. … Jagtap,
S. (2013). Emotion Recognition Modulating Behavior of Intelligent Systems, Proc. IEEE
International Symposium on Multimedia, IEEE Computer Society Press. Doi:
10.1109/ISM.2013.72
25. Laver, K., George, S., Thomas, S., Deutsch, J.E., & Crotty, M. (2012). “Cochrane review:
virtual reality for stroke rehabilitation, European journal of physical and rehabilitation,
48(3):523-30.
26. Cameirão, M.S., Bermúdez, S., & Verschure, P. (2008). Virtual reality based upper extrem-
ity rehabilitation following stroke: A review. Journal of CyberTherapy & Rehabilitation,
1(1).
38
EPS Portugal 2017/2018 2017 - OPPORTUNITIES AND CHALLENGES for European Projects
38