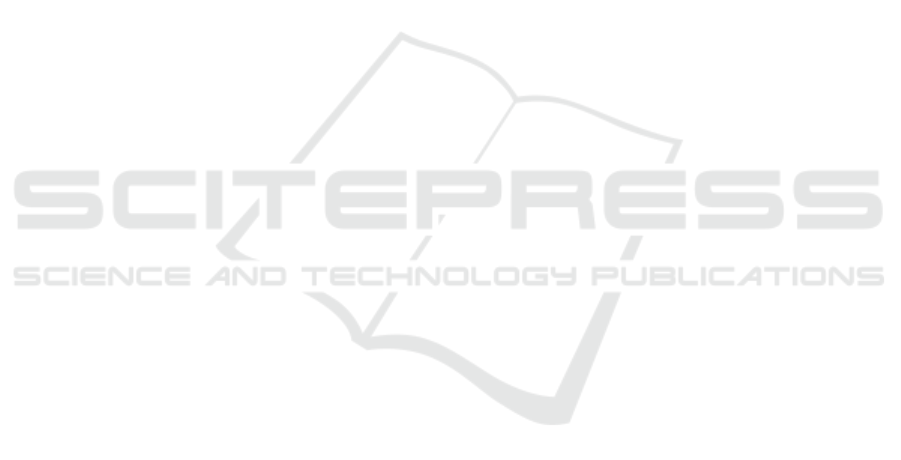
ACKNOWLEDGEMENTS
This work was supported by the BIA2013-43462-P
project funded by the Spanish Ministry of Economy
and Competitiveness and by the Regional European
Fund FED.
REFERENCES
Aldwaik S.Z. & Pontius Jr. R.G., 2012, Intensity analysis
to unify measurements of size and stationaity of land
changes by interval, category, and transition,
Landscape and Urban Planning 106, 103-114
Allen T.H.F. and Starr B., 1982, Hierarchy: Perspectives
for ecological complexity. University of Chicago
Press. Chicago, 310 p.
Burnicki A.C., Brown D.G. and Goovaerts P., 2007,
Simulating error propagation in land-cover change
analysis: The implications of temporal dependence.
Computers, Environment and Urban Systems 31, 282–
302
Camacho Olmedo M.T., Pontius Jr. R.G., Paegelow M.,
Mas J.F , 2015, Comparison of simulation models in
terms of quantity and allocation of land change.
Environmental Modelling & Software, v. 69 May
2015, p. 214-221.
Gómez Delgado, M. and Tarantola, S., 2006, “Global
sensitivity analysis, GIS and multi-criteria evaluation
for a sustainable planning of hazardous waste disposal
site in Spain”. International Journal of Geographical
Information Science, 20, 449-466.
Hu G., Hu J., Zhang C., Zhuang L., Song J., 2003, Short-
term traffic flow forecasting based on Markov chain
model. In: Intelligent Vehicles Symposium 9-11 June
2003. Proceedings IEEE, pp. 208-212.
Jokar Arsanjani, J., 2012, Dynamic Land-Use/Cover
Change Simulation: Geosimulation and Multi Agent-
Based Modelling, Springer Theses, Springer Verlag.
Kim J.H., 2013, Spatiotemporal scale dependency and
other sensitivities in dynamic land-use change
simulations. International Journal of Geographical
Information Science 27, 1782–1803
Lee Y.J., Lee J.W., Chai D.J., Hwang B.H. and Ryu K.H.,
2009, Mining temporal interval relational rules from
temporal data. Journal of Systems and Software 82,
155–167
Liu J. and Deng X., 2010, Progress of the research
methodologies on the temporal and spatial process of
LUCC. Chin. Sci. Bull. 55, 1354–1362
Mas J.F., Kolb M., Paegelow M., Camacho Olmedo M.T.,
Houet T., 2014, “Inductive pattern-based land
use/cover change models: A comparison of four
software packages”. Environmental Modelling &
Software, v 51 January 2014 P.94-111
Paegelow M., Camacho Olmedo M.T., Mas J.F., Houet T.,
2014, Benchmarking of LUCC modelling tools by
various validation techniques and error analysis.
Cybergeo, document 701, mis en ligne le 22 décembre
2014. URL : http://cybergeo.revues.org
Pontius Jr. R.G., 2000, “Quantification error versus
location error in comparison of categorical maps”.
Photogrammetric Engineering & Remote Sensing, 66
(8), 1011-1016.
Pontius Jr. R.G., Huffaker, D., Denman, K., 2004a,
„Useful techniques of validation for spatially explicit
land-change models”. Ecological Modelling, 179 (4),
445-461.
Pontius Jr. R.G., Shusas E. and McEachern M., 2004b.
Detecting important categorical land changes while
accounting for persistence. Agriculture, Ecosystems &
Environment 101(2-3) p.251-268
Pontius Jr R.G., Boersma, W., Castella, J.C., Clarke, K.,
de Nijs, T., Dietzel, C., Duan, Z., Fotsing, E.,
Goldstein, N., Kok, K., Koomen, E., Lippitt, C.D.,
McConnell, W,, Sood, A.M., Pijankowski, B.,
Pidhadia, S., Sweeney, S., Trung, T.N., Veldkamp,
A.T., Verburg, P.H., 2008, “Comparing the input,
output, and validation maps for several models of land
change”. Annals of Regional Science, 42 (1), 11-27.
Pontius Jr. R.G. and Lippitt C.D., 2006, Can error explain
map differences over time? Cartography and
Geographic Information Science, 33 (2), 159-171
Pontius JR. R.G., Gao Y., Giner N.M., Kohyama T., Osaki
M. and Hirose K., 2013. Design and interpretation of
intensity analysis illustrated by land change in Central
Kalimantan, Indonesia. Land, 2 (3), 351–369. DOI:
http://dx.doi.org/10.3390/land2030351.
GAMOLCS 2017 - International Workshop on Geomatic Approaches for Modelling Land Change Scenarios
356